


Research on methods to solve concurrency conflicts encountered in MongoDB technology development
Research on methods to solve concurrency conflicts encountered in MongoDB technology development
Introduction:
With the development of Internet technology, concurrent operations of databases have become An important issue in modern application development. During the development process of MongoDB technology, concurrency conflicts are often encountered. This article will study methods to solve MongoDB concurrency conflicts and illustrate them through specific code examples.
1. Causes and effects of concurrency conflicts
MongoDB is a non-relational database that adopts a document-based data storage model and has high scalability and flexible data structure. However, since MongoDB's data reading and writing operations are executed concurrently, it is easy to cause concurrency conflicts when multiple threads or processes read and write the same data at the same time. Concurrency conflicts will have a serious impact on the data consistency and reliability of the system, and may lead to data errors, data loss and other problems.
2. Methods to solve MongoDB concurrency conflicts
- Optimistic lock
Optimistic lock is a concurrency control method based on version control, by adding a version number to the data structure fields to achieve. When reading and updating data, first read the data and save the version number, and then when updating the data, compare the current version number with the saved version number to see if they are consistent. If they are consistent, the update is successful, otherwise the update fails. Optimistic locking can avoid waiting for locks and improve concurrency performance.
Sample code:
from pymongo import MongoClient from pymongo.errors import PyMongoError def optimistic_locking(collection, document_id, update_data): document = collection.find_one({'_id': document_id}) if document: current_version = document['version'] # Save the current version updated_data = update_data.copy() updated_data['version'] = current_version try: result = collection.update_one({'_id': document_id, 'version': current_version}, {'$set': updated_data}) if result.modified_count == 1: return True else: return False except PyMongoError: return False else: return False
- Pessimistic lock
Pessimistic lock is a database-based concurrency control method that locks the data when reading it. , to prevent other threads from modifying the data. MongoDB provides the function of locking read and write operations. When reading data, you can implement pessimistic locking by setting a lock.
Sample code:
from pymongo import MongoClient from pymongo.errors import PyMongoError def pessimistic_locking(collection, document_id, update_data): collection.find_one_and_update({'_id': document_id}, {'$set': update_data})
3. Summary
In the development process of MongoDB technology, resolving concurrency conflicts is a key task. Optimistic locking and pessimistic locking can effectively solve the problem of concurrency conflicts and improve the concurrency performance and data consistency of the system. In actual development, we need to choose appropriate concurrency control methods according to specific application scenarios, and carry out reasonable design and optimization in code implementation.
References:
- MongoDB official documentation - https://docs.mongodb.com/
- Mao Huojie. MongoDB Technology Insider [M]. People Posts and Telecommunications Press, 2018.
The above is the detailed content of Research on methods to solve concurrency conflicts encountered in MongoDB technology development. For more information, please follow other related articles on the PHP Chinese website!

Hot AI Tools

Undresser.AI Undress
AI-powered app for creating realistic nude photos

AI Clothes Remover
Online AI tool for removing clothes from photos.

Undress AI Tool
Undress images for free

Clothoff.io
AI clothes remover

AI Hentai Generator
Generate AI Hentai for free.

Hot Article

Hot Tools

Notepad++7.3.1
Easy-to-use and free code editor

SublimeText3 Chinese version
Chinese version, very easy to use

Zend Studio 13.0.1
Powerful PHP integrated development environment

Dreamweaver CS6
Visual web development tools

SublimeText3 Mac version
God-level code editing software (SublimeText3)

Hot Topics


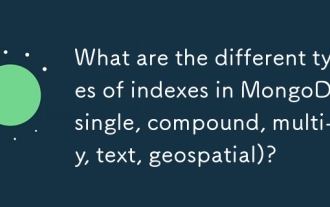
The article discusses various MongoDB index types (single, compound, multi-key, text, geospatial) and their impact on query performance. It also covers considerations for choosing the right index based on data structure and query needs.
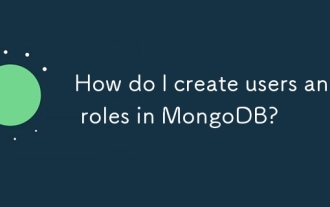
The article discusses creating users and roles in MongoDB, managing permissions, ensuring security, and automating these processes. It emphasizes best practices like least privilege and role-based access control.
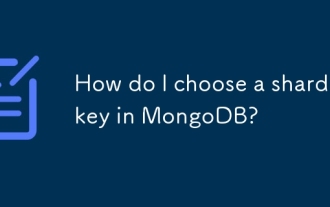
The article discusses selecting a shard key in MongoDB, emphasizing its impact on performance and scalability. Key considerations include high cardinality, query patterns, and avoiding monotonic growth.
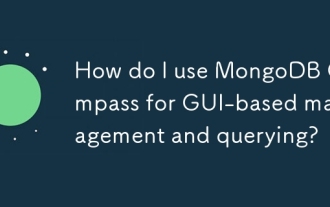
MongoDB Compass is a GUI tool for managing and querying MongoDB databases. It offers features for data exploration, complex query execution, and data visualization.
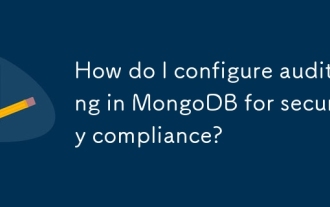
The article discusses configuring MongoDB auditing for security compliance, detailing steps to enable auditing, set up audit filters, and ensure logs meet regulatory standards. Main issue: proper configuration and analysis of audit logs for security
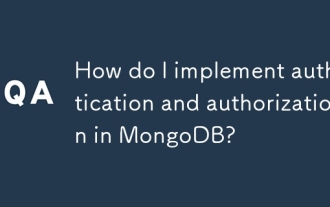
The article guides on implementing and securing MongoDB with authentication and authorization, discussing best practices, role-based access control, and troubleshooting common issues.
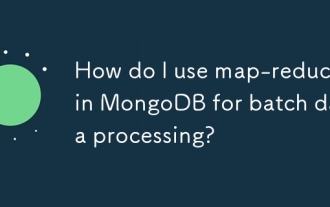
The article explains how to use map-reduce in MongoDB for batch data processing, its performance benefits for large datasets, optimization strategies, and clarifies its suitability for batch rather than real-time operations.
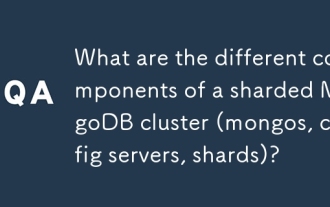
The article discusses components of a sharded MongoDB cluster: mongos, config servers, and shards. It focuses on how these components enable efficient data management and scalability.
