A brief analysis of the application of AI in medical physics
In recent years, the application of artificial intelligence (AI) and machine learning (ML) algorithms in biomedicine has continued to grow. This growth is most evident in areas related to radiation applications and medical physics, including the publication of special issues with sections on medical physics. This growth has inadvertently led to inconsistent reporting of AI/ML research results in the literature, confusing the interpretation of their results, and eroding trust in their potential impact.
Assessing MR Artifacts
As clinical magnetic resonance (MR) imaging increases in popularity and sophistication, it becomes increasingly difficult to gain a deep understanding of the physics underlying the ever-changing technology. This is especially true for practicing radiologists, whose primary responsibility is to interpret clinical images without necessarily understanding the complex equations that describe the underlying physics. However, the physics of magnetic resonance imaging play a role in clinical practice. important role as it determines image quality, and suboptimal image quality may hinder accurate diagnosis. This article provides an image-based explanation of the physics of common MR imaging artifacts and provides simple solutions for fixing each type of artifact.
Details solutions emerging from the latest technological advances that radiologists may not yet be familiar with. The types of artifacts discussed include those produced by voluntary and involuntary patient motion, magnetic susceptibility, magnetic field inhomogeneities, gradient nonlinearities, standing waves, aliasing, chemical shifts, and signal truncation. With increased awareness and understanding of these artifacts, radiologists will be better able to modify MR imaging protocols to optimize clinical image quality, thereby increasing diagnostic confidence.
Role in Radiation Oncology
Medical physics has a long tradition of modeling biological effects in radiation oncology. High-impact examples include the quantification of dose-volume effects based on clinical data, relevant to daily radiation treatment planning and optimization, and the adaptation and use of fractionation models aimed at converting physical doses into biologically equivalent doses to tumors.
Medical physicists possess the basic physical skills to establish mathematical descriptions of biological or clinical problems and have the ability to simplify complex relationships to the greatest extent possible. In addition, medical physics training in basic mathematics, statistics, biology, and clinical aspects allows medical physicists to interact relatively easily with the professionals needed for successful interdisciplinary teams to solve modeling problems. Machine learning and artificial intelligence-based models derived from data can be useful, but require an appropriate level of understanding and extensive validation to provide sufficient confidence for clinical use.
The role of medical physicists is not just to implement artificial intelligence, but also to act as facilitators of data collection and data farming, to establish and manage advanced data sharing platforms and to contribute to new innovations such as umbrella protocols and basket trials. Methods
Conclusion
In AI/ML applications in the field of medical physics, we need to clearly state and justify the problem of using these algorithms and emphasize the innovative nature of the method. We need to briefly describe how data is divided into subsets for training, validation, and independent testing of AI/ML algorithms. Next, we need to summarize the results and statistical indicators that quantify the performance of AI/ML algorithms
The above is the detailed content of A brief analysis of the application of AI in medical physics. For more information, please follow other related articles on the PHP Chinese website!

Hot AI Tools

Undresser.AI Undress
AI-powered app for creating realistic nude photos

AI Clothes Remover
Online AI tool for removing clothes from photos.

Undress AI Tool
Undress images for free

Clothoff.io
AI clothes remover

Video Face Swap
Swap faces in any video effortlessly with our completely free AI face swap tool!

Hot Article

Hot Tools

Notepad++7.3.1
Easy-to-use and free code editor

SublimeText3 Chinese version
Chinese version, very easy to use

Zend Studio 13.0.1
Powerful PHP integrated development environment

Dreamweaver CS6
Visual web development tools

SublimeText3 Mac version
God-level code editing software (SublimeText3)

Hot Topics


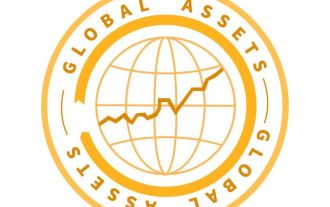
Global Assets launches a new AI intelligent trading system to lead the new era of trading efficiency! The well-known comprehensive trading platform Global Assets officially launched its AI intelligent trading system, aiming to use technological innovation to improve global trading efficiency, optimize user experience, and contribute to the construction of a safe and reliable global trading platform. The move marks a key step for global assets in the field of smart finance, further consolidating its global market leadership. Opening a new era of technology-driven and open intelligent trading. Against the backdrop of in-depth development of digitalization and intelligence, the trading market's dependence on technology is increasing. The AI intelligent trading system launched by Global Assets integrates cutting-edge technologies such as big data analysis, machine learning and blockchain, and is committed to providing users with intelligent and automated trading services to effectively reduce human factors.
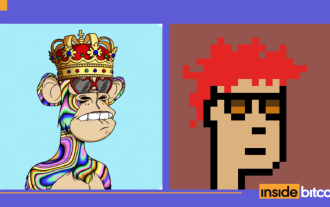
DefiDungeons, an NFT series in a fantasy placement RPG game powered by the Solana blockchain, showed strong market performance at the beginning of April 2025. The global NFT market started strongly in April after weeks of growth in trading volume. In the past 24 hours, the total NFT transaction volume reached US$14 million, with trading volume soaring 46% from the previous day. Here are the hottest NFT series in the first week of April: DefiDungeonsNFT series: This fantasy placement RPG game NFT on the Solana blockchain became the best-selling NFT series in the first week of April. In the past 24 hours, the transaction volume reached US$1.5 million, with a total market value of more than US$5 million.
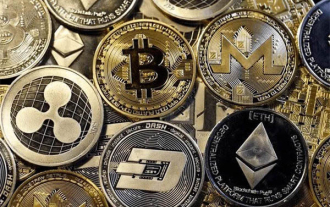
Binance, OKX, Coinbase, Kraken, Huobi, Gate.io, Gemini, XBIT, Bitget and MEXC are all strictly selected platforms for regulatory selection. 1. Binance adapts to supervision by setting up a compliance sub-platform. 2. OKX strengthens compliance management through KYB verification. 3.Coinbase is known for its compliance and user-friendliness. 4.Kraken provides powerful KYC and anti-money laundering measures. 5. Huobi operates in compliance with the Asian market. 6.Gate.io focuses on transaction security and compliance. 7. Gemini focuses on security and transparency. 8.XBIT is a model of decentralized transaction compliance. 9.Bitget continues to work in compliance operations. 10.MEXC passed
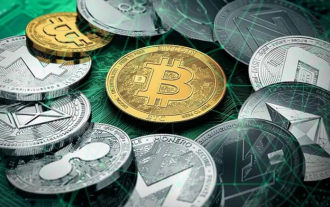
Factors of rising virtual currency prices include: 1. Increased market demand, 2. Decreased supply, 3. Stimulated positive news, 4. Optimistic market sentiment, 5. Macroeconomic environment; Decline factors include: 1. Decreased market demand, 2. Increased supply, 3. Strike of negative news, 4. Pessimistic market sentiment, 5. Macroeconomic environment.
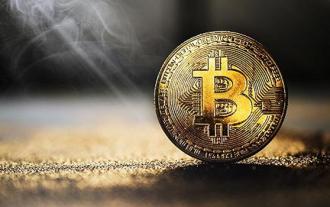
The investment prospects of crypto finance and AaaS businesses are analyzed as follows: 1. Opportunities of crypto finance include market size growth, gradual clear regulation and expansion of application scenarios, but face market volatility and technical security challenges. 2. The opportunities of AaaS business lie in the promotion of technological innovation, data value mining and rich application scenarios, but the challenges include technical complexity and market acceptance.
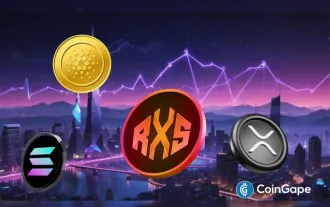
In the volatile cryptocurrency market, investors are looking for alternatives that go beyond popular currencies. Although well-known cryptocurrencies such as Solana (SOL), Cardano (ADA), XRP and Dogecoin (DOGE) also face challenges such as market sentiment, regulatory uncertainty and scalability. However, a new emerging project, RexasFinance (RXS), is emerging. It does not rely on celebrity effects or hype, but focuses on combining real-world assets (RWA) with blockchain technology to provide investors with an innovative way to invest. This strategy makes it hoped to be one of the most successful projects of 2025. RexasFi
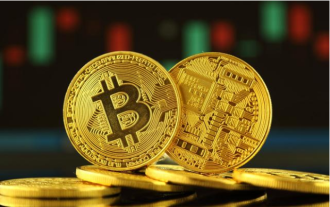
Recommended top ten trading app platforms in the 2025 currency circle: 1. OKX, 2. Binance, 3. Gate.io, 4. Bybit, 5. Kraken, 6. KuCoin, 7. Bitget, 8. HTX, 9. MEXC, 10. Coinbase. This ranking is based on comprehensive evaluations such as security, liquidity, user experience and feature richness.
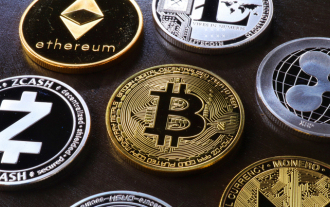
The top ten digital currency exchange apps in the world in 2025 are: 1. OKX, 2. Binance, 3. Gate.io, 4. Bybit, 5. Bitget, 6. MEXC, 7. KuCoin, 8. Kraken, 9. HTX, 10. Coinbase. These exchanges are evaluated from four dimensions: security, liquidity, product innovation and user experience, and each has different core advantages and innovative functions.
