How to do data visualization and exploration in Python
How to perform data visualization and exploration in Python
Data visualization and exploration is one of the important aspects of data analysis. In Python, with the help of various powerful libraries and Tools allow us to easily visualize and explore data. This article will introduce commonly used data visualization libraries and techniques in Python, and give specific code examples.
- Introduction
Data visualization is a method of displaying abstract data in an intuitive and easy-to-understand way. Through visualization, we can better understand the distribution, relationships and characteristics of data. There are many libraries and tools for data visualization in Python, such as Matplotlib, Seaborn, Plotly, etc. - Data preparation
Before performing data visualization, you first need to prepare the data to be analyzed. This article takes the Iris data set as an example. The Iris data set is a classic data set in the UCI machine learning library. It contains 150 samples of three varieties of iris flowers (Setosa, Versicolor, and Virginica). Each sample contains Four characteristics (Sepal length, Sepal width, Petal length, Petal width) are included.
First, you need to install the pandas library for data processing and analysis. Then, use the following code to read the Iris data set and prepare for simple data visualization:
import pandas as pd
Read the Iris data set
iris_data = pd.read_csv ('iris.csv')
View the first few rows of the data set
print(iris_data.head())
View the basic information of the data set
print(iris_data.info())
- Single variable data visualization
Single variable data visualization refers to visualizing the distribution of a single variable. Commonly used methods include histograms, histograms, and boxplots.
Taking Sepal length (calyx length) as an example, the code example of using the Matplotlib library to draw a histogram is as follows:
import matplotlib.pyplot as plt
Draw a column Figure
plt.bar(iris_data['Species'], iris_data['Sepal length'])
plt.xlabel('Species') # Set the x-axis label
plt.ylabel(' Sepal length') # Set the y-axis label
plt.title('Distribution of Sepal length') # Set the chart title
plt.show()
In addition, you can also use the Seaborn library to draw the histogram Figures and boxplots. The following is a code example for drawing a histogram:
import seaborn as sns
Drawing a histogram
sns.histplot(data=iris_data, x='Sepal length', kde =True)
plt.xlabel('Sepal length') # Set the x-axis label
plt.ylabel('Count') # Set the y-axis label
plt.title('Distribution of Sepal length') #Set chart title
plt.show()
- Double-variable data visualization
Double-variable data visualization refers to visualizing the relationship between two variables. Commonly used methods include scatter plots and heat maps.
Taking Sepal length and Petal length as an example, the code example for using the Matplotlib library to draw a scatter plot is as follows:
Draw a scatter plot
plt.scatter( iris_data['Sepal length'], iris_data['Petal length'])
plt.xlabel('Sepal length') # Set the x-axis label
plt.ylabel('Petal length') # Set the y-axis label
plt.title('Relationship between Sepal length and Petal length') #Set the chart title
plt.show()
In addition, you can also use the Seaborn library to draw a heat map to show the relationship between variables correlation. The following is a code example for drawing a heat map:
Calculate the correlation coefficient matrix between variables
correlation_matrix = iris_data[['Sepal length', 'Sepal width', 'Petal length', ' Petal width']].corr()
Draw a heat map
sns.heatmap(correlation_matrix, annot=True, cmap='coolwarm')
plt.title('Correlation Matrix ')
plt.show()
- Multivariable data visualization
Multivariable data visualization refers to visualizing the relationship between multiple variables. Commonly used methods include scatter matrices and parallel coordinate plots.
Taking the four features of the Iris data set as an example, the code example of using the Seaborn library to draw the scatter matrix is as follows:
Draw the scatter matrix
sns. pairplot(iris_data, hue='Species')
plt.show()
In addition, you can also use the Plotly library to draw parallel coordinate plots. The following is a code example for drawing parallel coordinate plots:
import plotly.express as px
Draw parallel coordinates graph
fig = px.parallel_coordinates(iris_data, color='Species')
fig.show()
Summary
This article introduces methods for data visualization and exploration in Python and gives specific code examples. Through data visualization and exploration, we can better understand the distribution, relationships, and characteristics of data, thereby providing a foundation and guidance for subsequent data analysis and modeling. In practical applications, appropriate visualization methods and technologies can also be selected based on specific needs and data characteristics to further explore the value of data.
The above is the detailed content of How to do data visualization and exploration in Python. For more information, please follow other related articles on the PHP Chinese website!

Hot AI Tools

Undresser.AI Undress
AI-powered app for creating realistic nude photos

AI Clothes Remover
Online AI tool for removing clothes from photos.

Undress AI Tool
Undress images for free

Clothoff.io
AI clothes remover

Video Face Swap
Swap faces in any video effortlessly with our completely free AI face swap tool!

Hot Article

Hot Tools

Notepad++7.3.1
Easy-to-use and free code editor

SublimeText3 Chinese version
Chinese version, very easy to use

Zend Studio 13.0.1
Powerful PHP integrated development environment

Dreamweaver CS6
Visual web development tools

SublimeText3 Mac version
God-level code editing software (SublimeText3)

Hot Topics
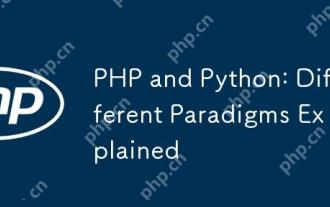
PHP is mainly procedural programming, but also supports object-oriented programming (OOP); Python supports a variety of paradigms, including OOP, functional and procedural programming. PHP is suitable for web development, and Python is suitable for a variety of applications such as data analysis and machine learning.
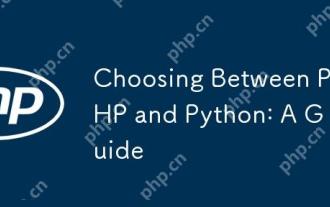
PHP is suitable for web development and rapid prototyping, and Python is suitable for data science and machine learning. 1.PHP is used for dynamic web development, with simple syntax and suitable for rapid development. 2. Python has concise syntax, is suitable for multiple fields, and has a strong library ecosystem.
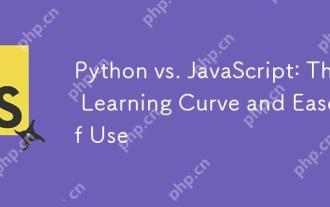
Python is more suitable for beginners, with a smooth learning curve and concise syntax; JavaScript is suitable for front-end development, with a steep learning curve and flexible syntax. 1. Python syntax is intuitive and suitable for data science and back-end development. 2. JavaScript is flexible and widely used in front-end and server-side programming.
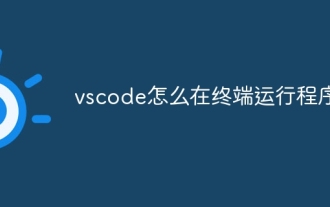
In VS Code, you can run the program in the terminal through the following steps: Prepare the code and open the integrated terminal to ensure that the code directory is consistent with the terminal working directory. Select the run command according to the programming language (such as Python's python your_file_name.py) to check whether it runs successfully and resolve errors. Use the debugger to improve debugging efficiency.
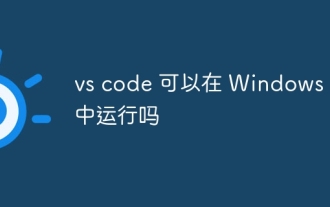
VS Code can run on Windows 8, but the experience may not be great. First make sure the system has been updated to the latest patch, then download the VS Code installation package that matches the system architecture and install it as prompted. After installation, be aware that some extensions may be incompatible with Windows 8 and need to look for alternative extensions or use newer Windows systems in a virtual machine. Install the necessary extensions to check whether they work properly. Although VS Code is feasible on Windows 8, it is recommended to upgrade to a newer Windows system for a better development experience and security.
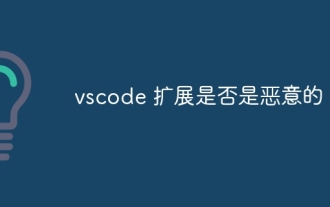
VS Code extensions pose malicious risks, such as hiding malicious code, exploiting vulnerabilities, and masturbating as legitimate extensions. Methods to identify malicious extensions include: checking publishers, reading comments, checking code, and installing with caution. Security measures also include: security awareness, good habits, regular updates and antivirus software.
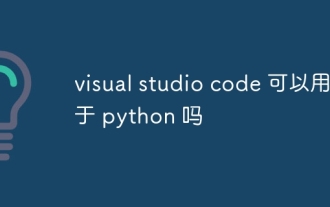
VS Code can be used to write Python and provides many features that make it an ideal tool for developing Python applications. It allows users to: install Python extensions to get functions such as code completion, syntax highlighting, and debugging. Use the debugger to track code step by step, find and fix errors. Integrate Git for version control. Use code formatting tools to maintain code consistency. Use the Linting tool to spot potential problems ahead of time.
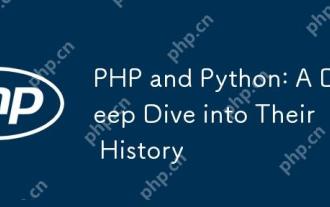
PHP originated in 1994 and was developed by RasmusLerdorf. It was originally used to track website visitors and gradually evolved into a server-side scripting language and was widely used in web development. Python was developed by Guidovan Rossum in the late 1980s and was first released in 1991. It emphasizes code readability and simplicity, and is suitable for scientific computing, data analysis and other fields.
