


How to use ChatGPT and Python to implement multi-round dialogue management
How to use ChatGPT and Python to achieve multi-round dialogue management
Introduction:
With the rapid development of artificial intelligence technology, Chatbot (chatbot) has become a An important part of class applications. Multi-turn dialogue is a key issue in Chatbot, which requires Chatbot to be able to understand the user's multiple consecutive speeches and give correct responses. This article will introduce how to use ChatGPT (a GPT-based chat generation model) and Python language to implement multi-round dialogue management, and provide specific code examples.
1. Introduction to ChatGPT
ChatGPT is a chat generation model based on GPT-3 (generative pre-training model) developed by OpenAI. It can be fine-tuned with example conversations to learn to generate responses similar to human conversations. ChatGPT can be used to provide Chatbot with powerful dialogue generation capabilities.
2. Principle of multi-round dialogue management
The goal of multi-round dialogue management is to keep Chatbot relevant in the user's continuous speeches and generate reasonable replies. A common approach is to use a stateful model. The model generates responses in each conversation round by recording contextual information and taking previous conversations as input.
Specifically, the process of multi-round dialogue management includes the following steps:
- Initialize Chatbot state: When the conversation starts, Chatbot needs to initialize its state, including conversation history and Other necessary information.
- Receive user input: Chatbot receives user input and adds it to the conversation history.
- Generate replies: Use the ChatGPT model, taking the conversation history as input, to generate replies.
- Update Conversation History: Add the generated reply to the conversation history.
- Repeat steps 2-4 until the end condition is met.
3. Use Python to implement multi-round dialogue management
The following is a sample code for using Python language to implement multi-round dialogue management:
import openai openai.api_key = 'your_api_key' def initialize_chatbot_state(): # 初始化Chatbot状态 chatbot_state = { 'dialogue_history': [] } return chatbot_state def generate_reply(chatbot_state, user_input): # 将用户输入添加到对话历史 chatbot_state['dialogue_history'].append(user_input) # 使用ChatGPT生成回复 response = openai.Completion.create( engine='text-davinci-003', prompt=' '.join(chatbot_state['dialogue_history']), max_tokens=50, temperature=0.7, n = 1, stop = None ) # 更新对话历史 chatbot_state['dialogue_history'].append(response.choices[0].text.strip()) # 返回生成的回复 return response.choices[0].text.strip() def main(): # 初始化Chatbot状态 chatbot_state = initialize_chatbot_state() while True: # 接收用户输入 user_input = input("用户:") # 生成回复 reply = generate_reply(chatbot_state, user_input) # 打印回复 print("Chatbot:", reply) # 结束条件判断 if user_input == "结束": break if __name__ == "__main__": main()
This code is used to call OpenAI’s ChatGPT The model implements a simple conversational interaction. In the main function, we use the initialize_chatbot_state function to initialize the Chatbot's state and generate a reply through the generate_reply function. The conversation progresses by looping the interaction until the user enters "end".
Conclusion:
By using ChatGPT and Python to implement multi-round dialogue management, we can build a Chatbot with dialogue generation capabilities. This provides powerful tools and technical support for various application scenarios (such as customer service, intelligent assistants, etc.). I hope the introduction and sample code in this article can help you better implement multi-turn dialogue management.
The above is the detailed content of How to use ChatGPT and Python to implement multi-round dialogue management. For more information, please follow other related articles on the PHP Chinese website!

Hot AI Tools

Undresser.AI Undress
AI-powered app for creating realistic nude photos

AI Clothes Remover
Online AI tool for removing clothes from photos.

Undress AI Tool
Undress images for free

Clothoff.io
AI clothes remover

AI Hentai Generator
Generate AI Hentai for free.

Hot Article

Hot Tools

Notepad++7.3.1
Easy-to-use and free code editor

SublimeText3 Chinese version
Chinese version, very easy to use

Zend Studio 13.0.1
Powerful PHP integrated development environment

Dreamweaver CS6
Visual web development tools

SublimeText3 Mac version
God-level code editing software (SublimeText3)

Hot Topics


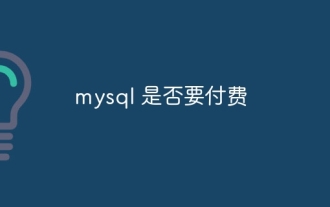
MySQL has a free community version and a paid enterprise version. The community version can be used and modified for free, but the support is limited and is suitable for applications with low stability requirements and strong technical capabilities. The Enterprise Edition provides comprehensive commercial support for applications that require a stable, reliable, high-performance database and willing to pay for support. Factors considered when choosing a version include application criticality, budgeting, and technical skills. There is no perfect option, only the most suitable option, and you need to choose carefully according to the specific situation.

HadiDB: A lightweight, high-level scalable Python database HadiDB (hadidb) is a lightweight database written in Python, with a high level of scalability. Install HadiDB using pip installation: pipinstallhadidb User Management Create user: createuser() method to create a new user. The authentication() method authenticates the user's identity. fromhadidb.operationimportuseruser_obj=user("admin","admin")user_obj.
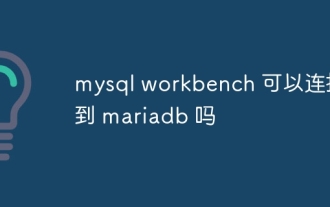
MySQL Workbench can connect to MariaDB, provided that the configuration is correct. First select "MariaDB" as the connector type. In the connection configuration, set HOST, PORT, USER, PASSWORD, and DATABASE correctly. When testing the connection, check that the MariaDB service is started, whether the username and password are correct, whether the port number is correct, whether the firewall allows connections, and whether the database exists. In advanced usage, use connection pooling technology to optimize performance. Common errors include insufficient permissions, network connection problems, etc. When debugging errors, carefully analyze error information and use debugging tools. Optimizing network configuration can improve performance
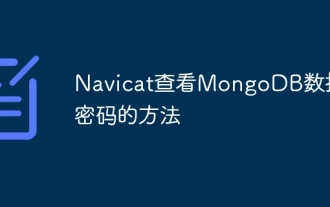
It is impossible to view MongoDB password directly through Navicat because it is stored as hash values. How to retrieve lost passwords: 1. Reset passwords; 2. Check configuration files (may contain hash values); 3. Check codes (may hardcode passwords).
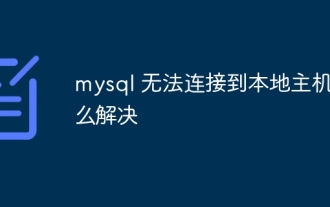
The MySQL connection may be due to the following reasons: MySQL service is not started, the firewall intercepts the connection, the port number is incorrect, the user name or password is incorrect, the listening address in my.cnf is improperly configured, etc. The troubleshooting steps include: 1. Check whether the MySQL service is running; 2. Adjust the firewall settings to allow MySQL to listen to port 3306; 3. Confirm that the port number is consistent with the actual port number; 4. Check whether the user name and password are correct; 5. Make sure the bind-address settings in my.cnf are correct.
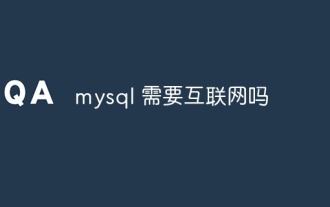
MySQL can run without network connections for basic data storage and management. However, network connection is required for interaction with other systems, remote access, or using advanced features such as replication and clustering. Additionally, security measures (such as firewalls), performance optimization (choose the right network connection), and data backup are critical to connecting to the Internet.
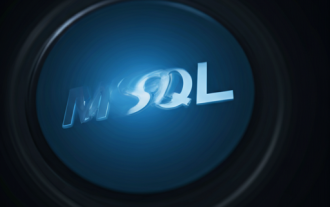
MySQL database performance optimization guide In resource-intensive applications, MySQL database plays a crucial role and is responsible for managing massive transactions. However, as the scale of application expands, database performance bottlenecks often become a constraint. This article will explore a series of effective MySQL performance optimization strategies to ensure that your application remains efficient and responsive under high loads. We will combine actual cases to explain in-depth key technologies such as indexing, query optimization, database design and caching. 1. Database architecture design and optimized database architecture is the cornerstone of MySQL performance optimization. Here are some core principles: Selecting the right data type and selecting the smallest data type that meets the needs can not only save storage space, but also improve data processing speed.
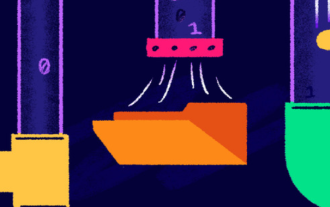
As a data professional, you need to process large amounts of data from various sources. This can pose challenges to data management and analysis. Fortunately, two AWS services can help: AWS Glue and Amazon Athena.
