ChatGPT Java: How to build a personalized recommendation system
ChatGPT Java: How to build a personalized recommendation system, specific code examples are required
In today's era of information explosion, personalized recommendation systems have become a key issue in the business field an important technology. By analyzing users' historical behavior and interests, these systems are able to provide users with recommended content that matches their personal preferences and needs. This article will introduce how to use Java to build a simple personalized recommendation system and provide specific code examples.
- Data collection and preprocessing
The core of the personalized recommendation system is the user's behavioral data. We need to collect users' historical browsing records, purchasing behavior, rating data, etc. In Java, a database can be used to store and manage this data. The following is a simple code example that connects to the database via Java JDBC and inserts the user's browsing history data:
import java.sql.Connection; import java.sql.DriverManager; import java.sql.PreparedStatement; import java.sql.SQLException; public class DataCollector { private static final String JDBC_URL = "jdbc:mysql://localhost:3306/recommendation_system"; private static final String USERNAME = "root"; private static final String PASSWORD = "password"; public static void main(String[] args) { try(Connection connection = DriverManager.getConnection(JDBC_URL, USERNAME, PASSWORD)) { String sql = "INSERT INTO user_browsing_history (user_id, item_id, timestamp) VALUES (?, ?, ?)"; PreparedStatement statement = connection.prepareStatement(sql); // 假设有一个用户浏览了商品1和商品2 statement.setInt(1, 1); // 用户ID statement.setInt(2, 1); // 商品ID statement.setTimestamp(3, new java.sql.Timestamp(System.currentTimeMillis())); // 事件时间戳 statement.executeUpdate(); statement.setInt(1, 1); statement.setInt(2, 2); statement.setTimestamp(3, new java.sql.Timestamp(System.currentTimeMillis())); statement.executeUpdate(); } catch (SQLException e) { e.printStackTrace(); } } }
- User similarity calculation
In order to achieve personalization Recommendation, we need to find other users or products with similar interests to the target user. Here, we can use collaborative filtering algorithm to calculate the similarity between users. The following is a simple code example that uses cosine similarity to calculate the similarity between users:
import java.util.HashMap; import java.util.Map; public class SimilarityCalculator { public static void main(String[] args) { // 假设有两位用户 Map<Integer, Map<Integer, Integer>> userItems = new HashMap<>(); userItems.put(1, new HashMap<>()); userItems.get(1).put(1, 5); // 用户1对商品1的评分是5 userItems.get(1).put(2, 3); // 用户1对商品2的评分是3 userItems.put(2, new HashMap<>()); userItems.get(2).put(1, 4); // 用户2对商品1的评分是4 userItems.get(2).put(2, 2); // 用户2对商品2的评分是2 int userId1 = 1; int userId2 = 2; double similarity = calculateCosineSimilarity(userItems.get(userId1), userItems.get(userId2)); System.out.println("用户1和用户2的相似度为:" + similarity); } private static double calculateCosineSimilarity(Map<Integer, Integer> user1, Map<Integer, Integer> user2) { double dotProduct = 0.0; double normUser1 = 0.0; double normUser2 = 0.0; for (Integer itemId : user1.keySet()) { if (user2.containsKey(itemId)) { dotProduct += user1.get(itemId) * user2.get(itemId); } normUser1 += Math.pow(user1.get(itemId), 2); } for (Integer itemId : user2.keySet()) { normUser2 += Math.pow(user2.get(itemId), 2); } return dotProduct / (Math.sqrt(normUser1) * Math.sqrt(normUser2)); } }
- Recommendation algorithm implementation
With the similarity between users Based on the calculation results, we can use the neighborhood-based collaborative filtering algorithm to make recommendations. The following is a simple code example that generates recommendation results for target users based on the similarity between users:
import java.util.*; public class RecommendationEngine { public static void main(String[] args) { // 假设有3位用户 Map<Integer, Map<Integer, Integer>> userItems = new HashMap<>(); userItems.put(1, new HashMap<>()); userItems.get(1).put(1, 5); // 用户1对商品1的评分是5 userItems.get(1).put(2, 3); // 用户1对商品2的评分是3 userItems.get(1).put(3, 4); // 用户1对商品3的评分是4 userItems.put(2, new HashMap<>()); userItems.get(2).put(1, 4); // 用户2对商品1的评分是4 userItems.get(2).put(3, 2); // 用户2对商品3的评分是2 userItems.put(3, new HashMap<>()); userItems.get(3).put(2, 5); // 用户3对商品2的评分是5 userItems.get(3).put(3, 2); // 用户3对商品3的评分是2 int targetUserId = 1; Map<Integer, Double> recommendItems = generateRecommendations(userItems, targetUserId); System.out.println("为用户1生成的推荐结果为:" + recommendItems); } private static Map<Integer, Double> generateRecommendations(Map<Integer, Map<Integer, Integer>> userItems, int targetUserId) { Map<Integer, Double> recommendItems = new HashMap<>(); Map<Integer, Integer> targetUserItems = userItems.get(targetUserId); for (Integer userId : userItems.keySet()) { if (userId != targetUserId) { Map<Integer, Integer> otherUserItems = userItems.get(userId); double similarity = calculateCosineSimilarity(targetUserItems, otherUserItems); for (Integer itemId : otherUserItems.keySet()) { if (!targetUserItems.containsKey(itemId)) { double rating = otherUserItems.get(itemId); double weightedRating = rating * similarity; recommendItems.put(itemId, recommendItems.getOrDefault(itemId, 0.0) + weightedRating); } } } } return recommendItems; } private static double calculateCosineSimilarity(Map<Integer, Integer> user1, Map<Integer, Integer> user2) { // 略,与上一个代码示例中的calculateCosineSimilarity()方法相同 } }
Through the above steps, we can use Java to build a simple personalized recommendation system. Of course, this is only the basis of a personalized recommendation system, and there is still a lot of room for optimization and expansion. I hope this article will help you understand the process of building a personalized recommendation system.
The above is the detailed content of ChatGPT Java: How to build a personalized recommendation system. For more information, please follow other related articles on the PHP Chinese website!

Hot AI Tools

Undresser.AI Undress
AI-powered app for creating realistic nude photos

AI Clothes Remover
Online AI tool for removing clothes from photos.

Undress AI Tool
Undress images for free

Clothoff.io
AI clothes remover

Video Face Swap
Swap faces in any video effortlessly with our completely free AI face swap tool!

Hot Article

Hot Tools

Notepad++7.3.1
Easy-to-use and free code editor

SublimeText3 Chinese version
Chinese version, very easy to use

Zend Studio 13.0.1
Powerful PHP integrated development environment

Dreamweaver CS6
Visual web development tools

SublimeText3 Mac version
God-level code editing software (SublimeText3)

Hot Topics


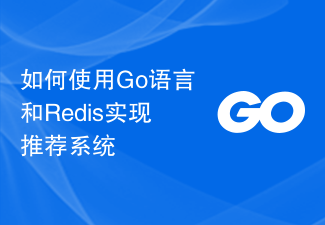
How to use Go language and Redis to implement a recommendation system. The recommendation system is an important part of the modern Internet platform. It helps users discover and obtain information of interest. The Go language and Redis are two very popular tools that can play an important role in the process of implementing recommendation systems. This article will introduce how to use Go language and Redis to implement a simple recommendation system, and provide specific code examples. Redis is an open source in-memory database that provides a key-value pair storage interface and supports a variety of data
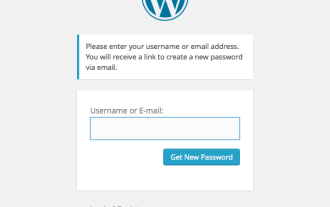
In the first two tutorials in this series, we built custom pages for logging in and registering new users. Now, there's only one part of the login flow left to explore and replace: What happens if a user forgets their password and wants to reset their WordPress password? In this tutorial, we'll tackle the last step and complete the personalized login plugin we've built throughout the series. The password reset feature in WordPress more or less follows the standard method on websites today: the user initiates a reset by entering their username or email address and requesting WordPress to reset their password. Create a temporary password reset token and store it in user data. A link containing this token will be sent to the user's email address. User clicks on the link. In the heavy
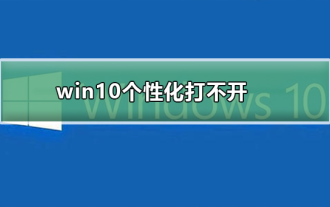
Many friends have found that after the win10 system is updated, the personalized settings cannot be opened. It keeps showing that the file does not have a program related to it to perform the operation. Please install a program. What is going on? Use winR to open "Run" , right-click to delete the entire ms-seeting, and you can open it. Let’s take a look at the details together. How to open personalization in win10 1. First, we press "Win+R" to call out the run, click and enter "services.msc", and then press Enter to confirm. 2. Then we click to open "windowsupdate" in the pop-up window and set the startup type to "disabled". 3. Then we put SoftwareDist in C:\Windows
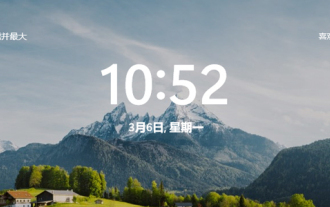
Windows 11’s Focus feature can automatically update your desktop wallpapers, themes, and lock screen interface, presenting you with a selection of beautiful pictures of landscapes, cities, animals, etc. every day. These images are all sourced from Bing search, which not only makes the user experience more personalized, but also occasionally displays practical suggestions and tips on the lock screen, bringing additional surprises and help to users. Method 1 to use Windows 11 Focus Desktop: Set Windows Focus Desktop Wallpaper 1 Press the Windows+I shortcut key to open "Settings" and select "Personalization" > "Background". 2 In the "Personalize background" drop-down list, select the "Windows Focus" option. Select Windows Spotlight Wallpaper
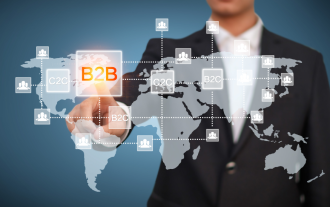
1. Problem background: The necessity and importance of cold start modeling. As a content platform, Cloud Music has a large amount of new content online every day. Although the amount of new content on the cloud music platform is relatively small compared to other platforms such as short videos, the actual amount may far exceed everyone's imagination. At the same time, music content is significantly different from short videos, news, and product recommendations. The life cycle of music spans extremely long periods of time, often measured in years. Some songs may explode after being dormant for months or years, and classic songs may still have strong vitality even after more than ten years. Therefore, for the recommendation system of music platforms, it is more important to discover unpopular and long-tail high-quality content and recommend them to the right users than to recommend other categories.
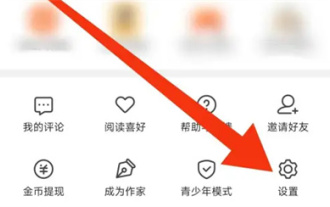
During the process of using Mao Mao Novel, the software will recommend some books of interest to us. How to set up personalized book recommendations? The following will introduce you to the specific operation method. After opening the "Seven Cats Free Novels" application on your phone, find the "My" option in the lower right corner of the page and look for the "Settings" function at the bottom of the page. Click to open the "Settings" option. 2. After coming to the settings page, there is a "Privacy Settings". When you see it, click on it to enter. 3. Next, find "Personalized Book Recommendations" on the privacy settings page. There is a switch button displayed behind it. Click the slider on it to set it to a colored state to turn on the function. The software will based on your reading preferences to recommend books that may be of interest.
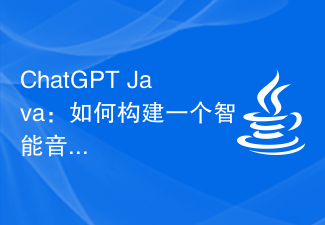
ChatGPTJava: How to build an intelligent music recommendation system, specific code examples are needed. Introduction: With the rapid development of the Internet, music has become an indispensable part of people's daily lives. As music platforms continue to emerge, users often face a common problem: how to find music that suits their tastes? In order to solve this problem, the intelligent music recommendation system came into being. This article will introduce how to use ChatGPTJava to build an intelligent music recommendation system and provide specific code examples. No.
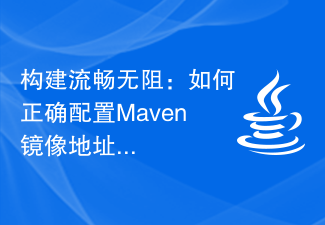
Smooth build: How to correctly configure the Maven image address When using Maven to build a project, it is very important to configure the correct image address. Properly configuring the mirror address can speed up project construction and avoid problems such as network delays. This article will introduce how to correctly configure the Maven mirror address and give specific code examples. Why do you need to configure the Maven image address? Maven is a project management tool that can automatically build projects, manage dependencies, generate reports, etc. When building a project in Maven, usually
