


Using search-enhanced generation technology to solve the artificial intelligence hallucination problem
Author| Rahul Pradhan
##Source| https: //www.infoworld.com/article/3708254/addressing-ai-hallucinations-with-retrieval-augmented-generation.html
Artificial intelligence is expected to become the most influential technology of our time . Recent advances in transformer technology and generative artificial intelligence have demonstrated their potential to unleash innovation and ingenuity at scale.
However, generative AI is not without its challenges—challenges that may even seriously hinder the adoption and value creation of this transformative technology. As generative AI models continue to increase in complexity and capability, they also present unique challenges, including generating output that is not based on the input data. "Illusion" means that the output results produced by the model, although coherent, may be divorced from the facts or input context. This article will briefly introduce the transformative impact of generative artificial intelligence, examine the shortcomings and challenges of the technology, and discuss techniques that can be used to mitigate hallucinations.
The transformative effect of generative artificial intelligence
Re-stated as: Generative artificial intelligence models leverage the power of deep learning A complex computational process to identify patterns in large data sets and use this information to create new and compelling output. These models use neural networks in machine learning technology, which are inspired by the way the human brain processes and interprets information, and continuously learns and improves over time
OpenAI's GPT Generative AI models such as -4 and Google’s PaLM 2 promise to bring innovations in automation, data analysis, and user experience. These models can write code, summarize articles, and even help diagnose diseases. However, the feasibility and ultimate value of these models depends on their accuracy and reliability. In critical areas such as healthcare, financial or legal services, reliability of accuracy is critical. But for all users to realize the full potential of generative AI, these challenges must be addressedDrawbacks of Large Language Models
LLM is fundamentally probabilistic and non-deterministic. They generate text based on the likelihood that a specific word sequence will occur next. LLM has no notion of knowledge and relies entirely on navigation through a corpus of trained data as a recommendation engine. The text they generate generally follows grammatical and semantic rules, but is entirely based on statistical consistency with the prompt.
This probabilistic nature of LLM is both an advantage and a disadvantage. If the goal is to arrive at the correct answer or to make a critical decision based on the answer, then hallucination is bad and can even cause damage. However, if the goal is a creative endeavor, the LLM can be used to foster artistic creativity, resulting in the creation of artwork, storylines, and screenplays relatively quickly.
However, regardless of the goal, failure to trust the output of an LLM model can have serious consequences. Not only would this erode trust in the capabilities of these systems, it would also significantly reduce the impact of AI in accelerating human productivity and innovation.
Ultimately, artificial intelligence is only as good as the data it is trained on.
The illusion of LLM is mainly caused by defects in the data set and training, including the following aspects:
Overfitting: Overfitting occurs when a model learns too well on training data (including noise and outliers). Model complexity, noisy training data, or insufficient training data can all lead to overfitting. This results in low-quality pattern recognition where the model does not generalize well to new data, leading to classification and prediction errors, factually incorrect outputs, outputs with low signal-to-noise ratio, or outright hallucinations.
- Data Quality: Mislabeling and misclassification of data used for training may play a role in hallucinations effect. Biased data or a lack of relevant data can actually result in model output that appears to be accurate but could prove to be harmful, depending on the scope of decisions the model recommends.
- Data Scarcity: Data scarcity or the need for fresh or relevant data is what creates illusions and hinders enterprise adoption One of the important issues in generative artificial intelligence. Refreshing data with the latest content and contextual data helps reduce illusions and bias.
- Addressing hallucinations in large language models
Illusion problems in LLM, including techniques such as fine-tuning, cue engineering, and retrieval-augmented generation (RAG).
- Fine-tuning refers to retraining a model using a domain-specific dataset to more accurately generate content relevant to that domain. However, retraining or fine-tuning the model takes a long time, and in addition, the data quickly becomes outdated without continuous training. In addition, retraining the model also brings a huge cost burden.
- The Hint Project aims to help ## by providing more descriptive and illustrative features in the input as hints # LLM produces high quality results. Providing the model with additional context and grounding it in facts reduces the likelihood that the model is hallucinating.
- Retrieval Enhanced Generation (RAG) is a method that focuses on using the most accurate and up-to-date information for LLM Provide a basic framework. The responsiveness of the LLM can be improved by feeding the model with facts from external knowledge bases in real time.
Retrieval-augmented generation and real-time data
Retrieval-augmented generation is one of the most promising techniques for improving the accuracy of large language models one. It turns out that RAG combined with real-time data can significantly reduce hallucinations.
RAG enables enterprises to leverage LLM by leveraging the latest proprietary and contextual data. In addition, RAG can also enrich the input content with specific contextual information, thereby helping the language model generate more accurate and contextually relevant responses. In an enterprise environment, fine-tuning is often impractical, but RAG offers a low-cost, high-yield alternative for delivering a personalized, informed user experience
In order to improve the efficiency of RAG models, it is necessary to combine RAG with an operational data store capable of storing data in the native language of LLMs, i.e. high-dimensional mathematical vectors called embeddings, using on the meaning of the encoded text. When a user asks a query, the database converts it into a numeric vector. In this way, related texts can be queried through the vector database regardless of whether they contain the same terms or not.
Highly available, high-performance databases capable of storing and querying massive amounts of unstructured data using semantic search are key components of the RAG process.
The above is the detailed content of Using search-enhanced generation technology to solve the artificial intelligence hallucination problem. For more information, please follow other related articles on the PHP Chinese website!

Hot AI Tools

Undresser.AI Undress
AI-powered app for creating realistic nude photos

AI Clothes Remover
Online AI tool for removing clothes from photos.

Undress AI Tool
Undress images for free

Clothoff.io
AI clothes remover

Video Face Swap
Swap faces in any video effortlessly with our completely free AI face swap tool!

Hot Article

Hot Tools

Notepad++7.3.1
Easy-to-use and free code editor

SublimeText3 Chinese version
Chinese version, very easy to use

Zend Studio 13.0.1
Powerful PHP integrated development environment

Dreamweaver CS6
Visual web development tools

SublimeText3 Mac version
God-level code editing software (SublimeText3)

Hot Topics


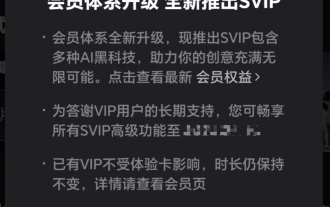
This site reported on June 27 that Jianying is a video editing software developed by FaceMeng Technology, a subsidiary of ByteDance. It relies on the Douyin platform and basically produces short video content for users of the platform. It is compatible with iOS, Android, and Windows. , MacOS and other operating systems. Jianying officially announced the upgrade of its membership system and launched a new SVIP, which includes a variety of AI black technologies, such as intelligent translation, intelligent highlighting, intelligent packaging, digital human synthesis, etc. In terms of price, the monthly fee for clipping SVIP is 79 yuan, the annual fee is 599 yuan (note on this site: equivalent to 49.9 yuan per month), the continuous monthly subscription is 59 yuan per month, and the continuous annual subscription is 499 yuan per year (equivalent to 41.6 yuan per month) . In addition, the cut official also stated that in order to improve the user experience, those who have subscribed to the original VIP
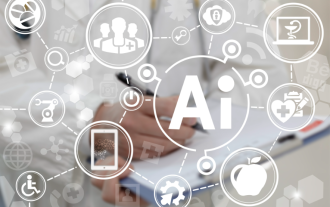
Translator | Bugatti Review | Chonglou This article describes how to use the GroqLPU inference engine to generate ultra-fast responses in JanAI and VSCode. Everyone is working on building better large language models (LLMs), such as Groq focusing on the infrastructure side of AI. Rapid response from these large models is key to ensuring that these large models respond more quickly. This tutorial will introduce the GroqLPU parsing engine and how to access it locally on your laptop using the API and JanAI. This article will also integrate it into VSCode to help us generate code, refactor code, enter documentation and generate test units. This article will create our own artificial intelligence programming assistant for free. Introduction to GroqLPU inference engine Groq
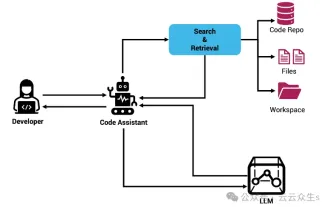
Improve developer productivity, efficiency, and accuracy by incorporating retrieval-enhanced generation and semantic memory into AI coding assistants. Translated from EnhancingAICodingAssistantswithContextUsingRAGandSEM-RAG, author JanakiramMSV. While basic AI programming assistants are naturally helpful, they often fail to provide the most relevant and correct code suggestions because they rely on a general understanding of the software language and the most common patterns of writing software. The code generated by these coding assistants is suitable for solving the problems they are responsible for solving, but often does not conform to the coding standards, conventions and styles of the individual teams. This often results in suggestions that need to be modified or refined in order for the code to be accepted into the application
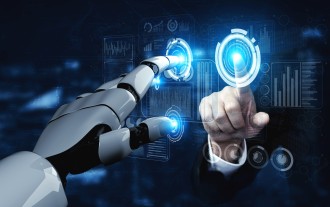
Large Language Models (LLMs) are trained on huge text databases, where they acquire large amounts of real-world knowledge. This knowledge is embedded into their parameters and can then be used when needed. The knowledge of these models is "reified" at the end of training. At the end of pre-training, the model actually stops learning. Align or fine-tune the model to learn how to leverage this knowledge and respond more naturally to user questions. But sometimes model knowledge is not enough, and although the model can access external content through RAG, it is considered beneficial to adapt the model to new domains through fine-tuning. This fine-tuning is performed using input from human annotators or other LLM creations, where the model encounters additional real-world knowledge and integrates it

To learn more about AIGC, please visit: 51CTOAI.x Community https://www.51cto.com/aigc/Translator|Jingyan Reviewer|Chonglou is different from the traditional question bank that can be seen everywhere on the Internet. These questions It requires thinking outside the box. Large Language Models (LLMs) are increasingly important in the fields of data science, generative artificial intelligence (GenAI), and artificial intelligence. These complex algorithms enhance human skills and drive efficiency and innovation in many industries, becoming the key for companies to remain competitive. LLM has a wide range of applications. It can be used in fields such as natural language processing, text generation, speech recognition and recommendation systems. By learning from large amounts of data, LLM is able to generate text
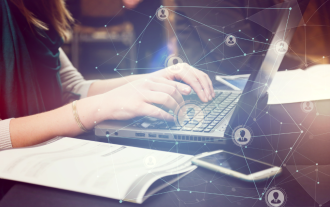
This article will open source the results of "Local Deployment of Large Language Models in OpenHarmony" demonstrated at the 2nd OpenHarmony Technology Conference. Open source address: https://gitee.com/openharmony-sig/tpc_c_cplusplus/blob/master/thirdparty/InferLLM/docs/ hap_integrate.md. The implementation ideas and steps are to transplant the lightweight LLM model inference framework InferLLM to the OpenHarmony standard system, and compile a binary product that can run on OpenHarmony. InferLLM is a simple and efficient L
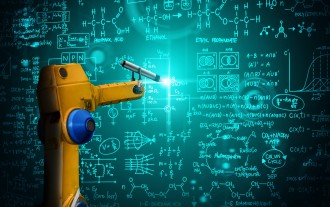
Machine learning is an important branch of artificial intelligence that gives computers the ability to learn from data and improve their capabilities without being explicitly programmed. Machine learning has a wide range of applications in various fields, from image recognition and natural language processing to recommendation systems and fraud detection, and it is changing the way we live. There are many different methods and theories in the field of machine learning, among which the five most influential methods are called the "Five Schools of Machine Learning". The five major schools are the symbolic school, the connectionist school, the evolutionary school, the Bayesian school and the analogy school. 1. Symbolism, also known as symbolism, emphasizes the use of symbols for logical reasoning and expression of knowledge. This school of thought believes that learning is a process of reverse deduction, through existing
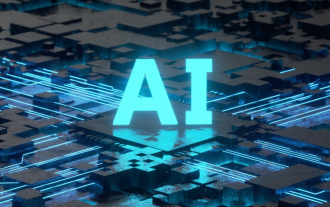
Editor |ScienceAI Question Answering (QA) data set plays a vital role in promoting natural language processing (NLP) research. High-quality QA data sets can not only be used to fine-tune models, but also effectively evaluate the capabilities of large language models (LLM), especially the ability to understand and reason about scientific knowledge. Although there are currently many scientific QA data sets covering medicine, chemistry, biology and other fields, these data sets still have some shortcomings. First, the data form is relatively simple, most of which are multiple-choice questions. They are easy to evaluate, but limit the model's answer selection range and cannot fully test the model's ability to answer scientific questions. In contrast, open-ended Q&A
