


In-depth analysis of the application practice of MongoDB in big data scenarios
In-depth analysis of the application practice of MongoDB in big data scenarios
Abstract: With the advent of the big data era, the scale of data continues to increase, and the need for database storage and processing The needs are becoming more and more urgent. As a non-relational database, MongoDB has been widely used in big data scenarios with its high scalability and flexible data model. This article will provide an in-depth analysis of the application practice of MongoDB in big data scenarios, including data modeling, data storage and query optimization. We hope that the introduction in this article can help readers better understand and apply MongoDB.
1. Data Modeling
In big data scenarios, data modeling is an important part of achieving efficient storage and query. Compared with traditional relational databases, MongoDB uses BSON (Binary JSON) format to store data. Compared with traditional row and column storage, BSON is more compact and has better scalability. When performing data modeling, the document structure needs to be designed according to specific business needs and query requirements to avoid data redundancy and frequent data association operations to improve query performance.
2. Data Storage
MongoDB supports horizontal expansion and can easily use the cluster architecture to handle large data storage requirements. In big data scenarios, sharding is usually used to achieve horizontal slicing and load balancing of data. Sharding can be divided according to a certain field value of the data to keep the amount of data on each shard balanced. At the same time, MongoDB also provides a variety of data replication mechanisms to ensure high data availability and disaster recovery capabilities.
3. Query Optimization
In big data scenarios, query performance is very critical. MongoDB provides a powerful query engine and flexible query language, allowing users to perform complex query operations based on specific business needs. To improve query performance, appropriate indexes can be used to speed up queries. MongoDB supports various types of indexes, including single-key indexes, composite indexes, and geographical indexes. By rationally selecting index fields, you can reduce the scanning scope of the query and improve the query efficiency.
4. Integration with Hadoop
In big data scenarios, Hadoop is usually used for data analysis and mining. MongoDB provides an integrated interface with Hadoop, which can easily import data from MongoDB into Hadoop for distributed computing. At the same time, MongoDB also supports an interface to output to Hadoop, and calculation results can be written back to MongoDB for storage and query. Through integration with Hadoop, the respective advantages of MongoDB and Hadoop can be fully utilized to achieve more complex big data analysis tasks.
Conclusion:
With the development of the big data era, MongoDB is increasingly used in big data scenarios. Through reasonable data modeling, optimized data storage and query operations, and integration with Hadoop, MongoDB's potential in big data scenarios can be maximized. In actual applications, the appropriate MongoDB version and configuration parameters need to be selected based on specific business requirements and system architecture. I hope the introduction in this article will be helpful to readers in applying MongoDB in big data scenarios.
The above is the detailed content of In-depth analysis of the application practice of MongoDB in big data scenarios. For more information, please follow other related articles on the PHP Chinese website!

Hot AI Tools

Undresser.AI Undress
AI-powered app for creating realistic nude photos

AI Clothes Remover
Online AI tool for removing clothes from photos.

Undress AI Tool
Undress images for free

Clothoff.io
AI clothes remover

AI Hentai Generator
Generate AI Hentai for free.

Hot Article

Hot Tools

Notepad++7.3.1
Easy-to-use and free code editor

SublimeText3 Chinese version
Chinese version, very easy to use

Zend Studio 13.0.1
Powerful PHP integrated development environment

Dreamweaver CS6
Visual web development tools

SublimeText3 Mac version
God-level code editing software (SublimeText3)

Hot Topics


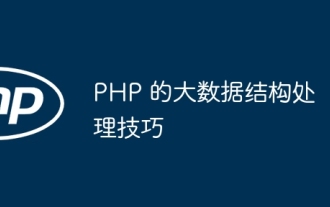
Big data structure processing skills: Chunking: Break down the data set and process it in chunks to reduce memory consumption. Generator: Generate data items one by one without loading the entire data set, suitable for unlimited data sets. Streaming: Read files or query results line by line, suitable for large files or remote data. External storage: For very large data sets, store the data in a database or NoSQL.
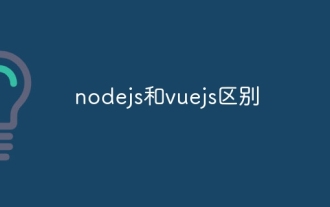
Node.js is a server-side JavaScript runtime, while Vue.js is a client-side JavaScript framework for creating interactive user interfaces. Node.js is used for server-side development, such as back-end service API development and data processing, while Vue.js is used for client-side development, such as single-page applications and responsive user interfaces.
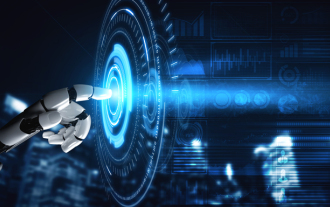
AEC/O (Architecture, Engineering & Construction/Operation) refers to the comprehensive services that provide architectural design, engineering design, construction and operation in the construction industry. In 2024, the AEC/O industry faces changing challenges amid technological advancements. This year is expected to see the integration of advanced technologies, heralding a paradigm shift in design, construction and operations. In response to these changes, industries are redefining work processes, adjusting priorities, and enhancing collaboration to adapt to the needs of a rapidly changing world. The following five major trends in the AEC/O industry will become key themes in 2024, recommending it move towards a more integrated, responsive and sustainable future: integrated supply chain, smart manufacturing
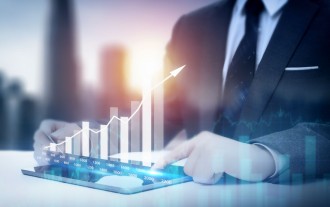
1. Background of the Construction of 58 Portraits Platform First of all, I would like to share with you the background of the construction of the 58 Portrait Platform. 1. The traditional thinking of the traditional profiling platform is no longer enough. Building a user profiling platform relies on data warehouse modeling capabilities to integrate data from multiple business lines to build accurate user portraits; it also requires data mining to understand user behavior, interests and needs, and provide algorithms. side capabilities; finally, it also needs to have data platform capabilities to efficiently store, query and share user profile data and provide profile services. The main difference between a self-built business profiling platform and a middle-office profiling platform is that the self-built profiling platform serves a single business line and can be customized on demand; the mid-office platform serves multiple business lines, has complex modeling, and provides more general capabilities. 2.58 User portraits of the background of Zhongtai portrait construction
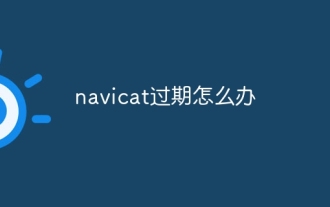
Solutions to resolve Navicat expiration issues include: renew the license; uninstall and reinstall; disable automatic updates; use Navicat Premium Essentials free version; contact Navicat customer support.
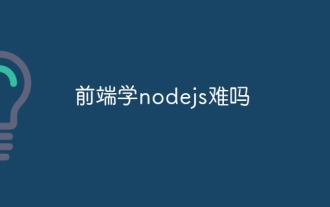
For front-end developers, the difficulty of learning Node.js depends on their JavaScript foundation, server-side programming experience, command line familiarity, and learning style. The learning curve includes entry-level and advanced-level modules focusing on fundamental concepts, server-side architecture, database integration, and asynchronous programming. Overall, learning Node.js is not difficult for developers who have a solid foundation in JavaScript and are willing to invest the time and effort, but for those who lack relevant experience, there may be certain challenges to overcome.
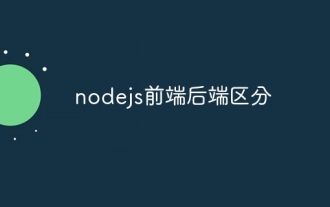
Node.js can be used for both front-end (handling user interface and interactions) and back-end (managing logic and data). The front-end uses HTML, CSS, and JavaScript frameworks, while the front-end uses Node.js framework, database, and cloud services. The focus is different (the front-end focuses on experience, the back-end focuses on functionality), the running environment is different (the front-end is in the browser, the back-end is on the server), and the tools are different (the front-end and back-end use different code compilation and packaging tool sets), although both use JavaScript , but with access to different APIs and libraries.
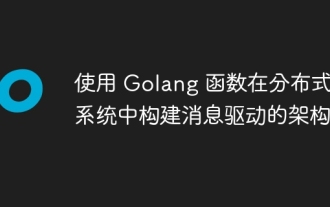
Building a message-driven architecture using Golang functions includes the following steps: creating an event source and generating events. Select a message queue for storing and forwarding events. Deploy a Go function as a subscriber to subscribe to and process events from the message queue.
