


An in-depth analysis of Apollo's lane changing strategy and its practical application
1. Introduction
1.1. The function of changing lanes
Simply put, the function of changing lanes is to select one of the reference lines from the reference lines to be selected. line, for use by Planning’s subsequent modules.
#It should be noted that the open source lane changing code provided by Apollo has been greatly deleted, and only a simple frame. Many functions, such as active lane changing, passive lane changing (due to obstacles), relatively complete state machine, lane changing window and Gap selection, etc. are missing. This technical article only shows content on the existing framework. Regarding the content of other lane changing functions, please look forward to the follow-up articles
1.2. Lane changing state machine
Apollo’s current lane changing state machine is as follows:
There are a few points worth noting about the state machine:
- The IN_CHANGE_LANE here includes both the lane change execution and lane change preparation stages. Under normal circumstances It should be distinguished. Apollo adopts the method of first switching the reference line and then performing the lane change action, while some friends will adopt the method of first performing the lane change action and then switching the reference line (the advantage is that in the frame when the reference line is switched, the lateral direction of the own car The distance is close to 0).
- The current code does not reflect when the lane change will fail. There is also reason to believe that it is caused by deleting the relevant code.
- The complete lane change status should At least include: lane change preparation, lane change execution, lane change maintenance, lane change cancellation, lane change completion and other statuses.
2. Code analysis
2.1. Overall process
The path where the relevant code is located: modules/planning/tasks/deciders/lane_change_decider/http://lane_change_decider.cc
- Handle the exception when
reference_line_info
is empty. - If forced lane change is configured
reckless_change_lane
istrue
, then directly Update Reference Line. - Get the lane changing status of the previous frame
prev_status
. When you first enter the program, there may be no status information inprev_status
(!prev_status-> has_status()
), at this time it is directly considered that the lane change is completed. - If the number of
reference_line_info
is 1 (that is,has_change_lane
isfalse
), indicating that the vehicle is in the patrol state. The lane where the vehicle is currently located has a unique lane line and there are no variable lanes around it, so you can directly update the lane change status. - Switch lane change The state machine
- The previous frame was in the lane change state
ChangeLaneStatus::IN_CHANGE_LANE
: If the Lane where the car was in the previous frame and the Lane where the car is in this frame If the same, it means that the vehicle is still walking towards the target lane. On the contrary, if the Lane where the vehicle was in the previous frame is different from the Lane where it is in this frame, it means that the vehicle is already in the target lane and the switch has been completed. - The previous frame was in the lane changing failed state
ChangeLaneStatus::CHANGE_LANE_FAILED
: If the lane changing fails, the state will be frozen for a period of time, and then switched to the lane changing state to prepare for the next lane changing. - The previous frame was in the lane change completed state
ChangeLaneStatus::CHANGE_LANE_FINISHED
: After the lane change is successful, it will also freeze for a period of time, and then switch to the lane changing state to prepare for the next lane change. The purpose of freezing the time here is to avoid frequent lane changes.
2.2. Safety check
IsClearToChangeLane
Determine whether the lane change is safe.
- This safety judgment method does not consider virtual obstacles (Virtual Obstacles) and static obstacles (Static Obstacles).
- Calculate the projection of dynamic obstacles on the Reference Line. The geometric meaning of the projection As shown below:
- If the vehicle is changing lanes
IsChangeLanePath
, use the projection relationship calculated above to ignore obstacles outside the lane of the target reference line - .
Determine whether the obstacle is traveling in the same direction as the vehiclesame_direction. Calculate the forward direction based on the relationship between the obstacle and the vehicle's traveling direction and speed relationship. Safety distance and backward safety distance
- .If the distance between the vehicle and the obstacle is greater than the safety distance calculated above, it is considered that it is safe to change lanes.
2.3. Update Reference Line
-
<span>Input:</span>
is_prioritize_change_lane
. Ifis_prioritize_change_lane
istrue
, then find the target reference line to switch to. . On the other hand, ifis_prioritize_change_lane
is false - , then find the reference line of the Lane where the current position of the vehicle is located.
reference_line_info->splice(reference_line_info-> begin(),*reference_line_info, iter)
;Put the reference line pointed to byiter
found in step 1 at the front of the linked list. Since Planning subsequent modules use reference_line_info( ).front(), so the front of the linked list indicates that the frame is the reference line used. For detailed instructions on the use of splice - , see C Basics.Output:
The above is the detailed content of An in-depth analysis of Apollo's lane changing strategy and its practical application. For more information, please follow other related articles on the PHP Chinese website!

Hot AI Tools

Undresser.AI Undress
AI-powered app for creating realistic nude photos

AI Clothes Remover
Online AI tool for removing clothes from photos.

Undress AI Tool
Undress images for free

Clothoff.io
AI clothes remover

AI Hentai Generator
Generate AI Hentai for free.

Hot Article

Hot Tools

Notepad++7.3.1
Easy-to-use and free code editor

SublimeText3 Chinese version
Chinese version, very easy to use

Zend Studio 13.0.1
Powerful PHP integrated development environment

Dreamweaver CS6
Visual web development tools

SublimeText3 Mac version
God-level code editing software (SublimeText3)

Hot Topics


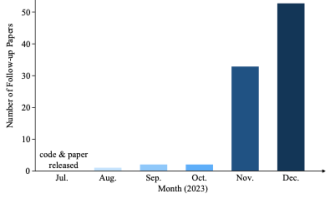
Written above & the author’s personal understanding Three-dimensional Gaussiansplatting (3DGS) is a transformative technology that has emerged in the fields of explicit radiation fields and computer graphics in recent years. This innovative method is characterized by the use of millions of 3D Gaussians, which is very different from the neural radiation field (NeRF) method, which mainly uses an implicit coordinate-based model to map spatial coordinates to pixel values. With its explicit scene representation and differentiable rendering algorithms, 3DGS not only guarantees real-time rendering capabilities, but also introduces an unprecedented level of control and scene editing. This positions 3DGS as a potential game-changer for next-generation 3D reconstruction and representation. To this end, we provide a systematic overview of the latest developments and concerns in the field of 3DGS for the first time.
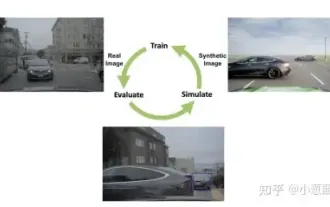
Yesterday during the interview, I was asked whether I had done any long-tail related questions, so I thought I would give a brief summary. The long-tail problem of autonomous driving refers to edge cases in autonomous vehicles, that is, possible scenarios with a low probability of occurrence. The perceived long-tail problem is one of the main reasons currently limiting the operational design domain of single-vehicle intelligent autonomous vehicles. The underlying architecture and most technical issues of autonomous driving have been solved, and the remaining 5% of long-tail problems have gradually become the key to restricting the development of autonomous driving. These problems include a variety of fragmented scenarios, extreme situations, and unpredictable human behavior. The "long tail" of edge scenarios in autonomous driving refers to edge cases in autonomous vehicles (AVs). Edge cases are possible scenarios with a low probability of occurrence. these rare events
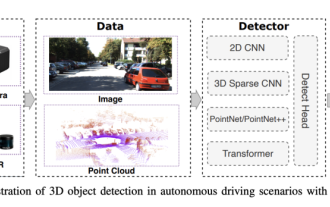
0.Written in front&& Personal understanding that autonomous driving systems rely on advanced perception, decision-making and control technologies, by using various sensors (such as cameras, lidar, radar, etc.) to perceive the surrounding environment, and using algorithms and models for real-time analysis and decision-making. This enables vehicles to recognize road signs, detect and track other vehicles, predict pedestrian behavior, etc., thereby safely operating and adapting to complex traffic environments. This technology is currently attracting widespread attention and is considered an important development area in the future of transportation. one. But what makes autonomous driving difficult is figuring out how to make the car understand what's going on around it. This requires that the three-dimensional object detection algorithm in the autonomous driving system can accurately perceive and describe objects in the surrounding environment, including their locations,
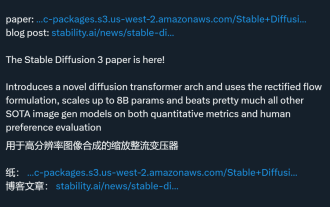
StableDiffusion3’s paper is finally here! This model was released two weeks ago and uses the same DiT (DiffusionTransformer) architecture as Sora. It caused quite a stir once it was released. Compared with the previous version, the quality of the images generated by StableDiffusion3 has been significantly improved. It now supports multi-theme prompts, and the text writing effect has also been improved, and garbled characters no longer appear. StabilityAI pointed out that StableDiffusion3 is a series of models with parameter sizes ranging from 800M to 8B. This parameter range means that the model can be run directly on many portable devices, significantly reducing the use of AI
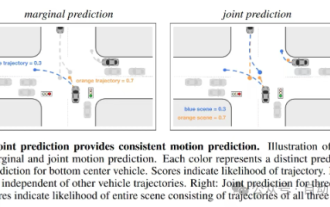
Trajectory prediction plays an important role in autonomous driving. Autonomous driving trajectory prediction refers to predicting the future driving trajectory of the vehicle by analyzing various data during the vehicle's driving process. As the core module of autonomous driving, the quality of trajectory prediction is crucial to downstream planning control. The trajectory prediction task has a rich technology stack and requires familiarity with autonomous driving dynamic/static perception, high-precision maps, lane lines, neural network architecture (CNN&GNN&Transformer) skills, etc. It is very difficult to get started! Many fans hope to get started with trajectory prediction as soon as possible and avoid pitfalls. Today I will take stock of some common problems and introductory learning methods for trajectory prediction! Introductory related knowledge 1. Are the preview papers in order? A: Look at the survey first, p

Original title: SIMPL: ASimpleandEfficientMulti-agentMotionPredictionBaselineforAutonomousDriving Paper link: https://arxiv.org/pdf/2402.02519.pdf Code link: https://github.com/HKUST-Aerial-Robotics/SIMPL Author unit: Hong Kong University of Science and Technology DJI Paper idea: This paper proposes a simple and efficient motion prediction baseline (SIMPL) for autonomous vehicles. Compared with traditional agent-cent
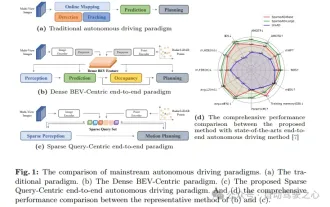
Written in front & starting point The end-to-end paradigm uses a unified framework to achieve multi-tasking in autonomous driving systems. Despite the simplicity and clarity of this paradigm, the performance of end-to-end autonomous driving methods on subtasks still lags far behind single-task methods. At the same time, the dense bird's-eye view (BEV) features widely used in previous end-to-end methods make it difficult to scale to more modalities or tasks. A sparse search-centric end-to-end autonomous driving paradigm (SparseAD) is proposed here, in which sparse search fully represents the entire driving scenario, including space, time, and tasks, without any dense BEV representation. Specifically, a unified sparse architecture is designed for task awareness including detection, tracking, and online mapping. In addition, heavy
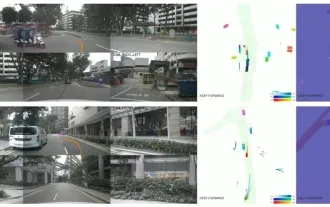
In the past month, due to some well-known reasons, I have had very intensive exchanges with various teachers and classmates in the industry. An inevitable topic in the exchange is naturally end-to-end and the popular Tesla FSDV12. I would like to take this opportunity to sort out some of my thoughts and opinions at this moment for your reference and discussion. How to define an end-to-end autonomous driving system, and what problems should be expected to be solved end-to-end? According to the most traditional definition, an end-to-end system refers to a system that inputs raw information from sensors and directly outputs variables of concern to the task. For example, in image recognition, CNN can be called end-to-end compared to the traditional feature extractor + classifier method. In autonomous driving tasks, input data from various sensors (camera/LiDAR
