


Don't let big models get fooled by benchmark evaluations! The test set is randomly included in the pre-training, the scores are falsely high, and the model becomes stupid.
"Don't let large models get fooled by benchmark evaluations."
This is the title of a latest study, from the School of Information at Renmin University, the School of Artificial Intelligence at Hillhouse, and the University of Illinois at Urbana-Champaign.
Research has found that it is becoming more and more common for relevant data in benchmark tests to be accidentally used for model training.
Because the pre-training corpus contains a lot of public text information, and the evaluation benchmark is also based on this information, this situation is inevitable.
Now the problem is getting worse as big models try to collect more public data.
You must know that the harm caused by this kind of data overlap is very great.
Not only will it lead to falsely high test scores for some parts of the model, but it will also cause the model's generalization ability to decline and the performance of irrelevant tasks to plummet. It may even cause large models to cause "harm" in practical applications.
So this study officially issued a warning and verified the actual hazards that may be induced through multiple simulation tests, specifically.
It is very dangerous for large models to "miss questions"
The research mainly tests and observes the impact of large models by simulating extreme data leakage situations.
There are four ways to extremely leak data:
- Use the training set of MMLU
- Use the training set of all test benchmarks except MMLU
- Use all training sets to test prompt
- Use all training sets, test sets and test prompts (This is the most extreme case, only an experimental simulation, it will not happen under normal circumstances)
Then the researchers "poisoned" the four large models, and then observed their performance in different benchmarks, mainly evaluating their performance in tasks such as question and answer, reasoning, and reading comprehension.
The models used are:
- GPT-Neo (1.3B)
- phi-1.5 (1.3B)
- OpenLLaMA (3B )
- LLaMA-2 (7B)
Also use LLaMA (13B/30B/65B) as a control group.
The results found that when the pre-training data of a large model contains data from a certain evaluation benchmark, it will perform better in this evaluation benchmark, but its performance in other unrelated tasks will decline.
For example, after training with the MMLU data set, while the scores of multiple large models improved in the MMLU test, their scores in the common sense benchmark HSwag and the mathematics benchmark GSM8K dropped.
This indicates that the generalization ability of large models is affected.
On the other hand, it may also result in falsely high scores on irrelevant tests.
The four training sets used to "poison" the large model as mentioned above only contain a small amount of Chinese data. However, after the large model was "poisoned", the scores in C3 (Chinese benchmark test) all became higher. .
This increase is unreasonable.
This kind of training data leakage can even cause model test scores to abnormally exceed the performance of larger models.
For example, phi-1.5 (1.3B) performs better than LLaMA65B on RACE-M and RACE-H, the latter being 50 times the size of the former.
But this kind of score increase is meaningless, it’s just cheating.
What’s more serious is that even tasks that have not had data leaked will be affected and their performance will decline.
As can be seen in the table below, in the code task HEval, the scores of both large models have dropped significantly.
After the data was leaked at the same time, the fine-tuning improvement of the large model was far inferior to the situation without leakage.
This study analyzes various possibilities in the event of data overlap/leakage.
For example, large model pre-training corpus and benchmark test data will use public texts (webpages, papers, etc.), so overlap is inevitable.
And currently large model evaluations are performed locally, or the results are obtained through API calls. This method cannot strictly check some abnormal numerical increases.
and the pre-training corpus of current large models are regarded as core secrets by all parties and cannot be evaluated by the outside world.
This resulted in large models being accidentally "poisoned".
How to avoid this problem? The research team also made some suggestions.
How to avoid it?
The research team gave three suggestions:
First, it is difficult to completely avoid data overlap in actual situations, so large models should use multiple benchmark tests for a more comprehensive evaluation.
Second, for large model developers, they should desensitize the data and disclose the detailed composition of the training corpus.
Third, for benchmark maintainers, benchmark data sources should be provided, the risk of data contamination should be analyzed, and multiple evaluations should be conducted using more diverse prompts.
However, the team also said that there are still certain limitations in this research. For example, there is no systematic testing of different degrees of data leakage, and the failure to directly introduce data leakage in pre-training for simulation.
This research was jointly brought by many scholars from the School of Information at Renmin University of China, the School of Artificial Intelligence at Hillhouse, and the University of Illinois at Urbana-Champaign.
In the research team, we found two giants in the field of data mining: Wen Jirong and Han Jiawei.
Professor Wen Jirong is currently the Dean of the Hillhouse School of Artificial Intelligence and the Dean of the School of Information at Renmin University of China. The main research directions are information retrieval, data mining, machine learning, and the training and application of large-scale neural network models.
Professor Han Jiawei is an expert in the field of data mining. He is currently a professor in the Department of Computer Science at the University of Illinois at Urbana-Champaign, an academician of the American Computer Society and an IEEE academician.
Paper address: https://arxiv.org/abs/2311.01964.
The above is the detailed content of Don't let big models get fooled by benchmark evaluations! The test set is randomly included in the pre-training, the scores are falsely high, and the model becomes stupid.. For more information, please follow other related articles on the PHP Chinese website!

Hot AI Tools

Undresser.AI Undress
AI-powered app for creating realistic nude photos

AI Clothes Remover
Online AI tool for removing clothes from photos.

Undress AI Tool
Undress images for free

Clothoff.io
AI clothes remover

Video Face Swap
Swap faces in any video effortlessly with our completely free AI face swap tool!

Hot Article

Hot Tools

Notepad++7.3.1
Easy-to-use and free code editor

SublimeText3 Chinese version
Chinese version, very easy to use

Zend Studio 13.0.1
Powerful PHP integrated development environment

Dreamweaver CS6
Visual web development tools

SublimeText3 Mac version
God-level code editing software (SublimeText3)

Hot Topics


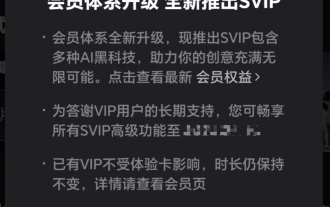
This site reported on June 27 that Jianying is a video editing software developed by FaceMeng Technology, a subsidiary of ByteDance. It relies on the Douyin platform and basically produces short video content for users of the platform. It is compatible with iOS, Android, and Windows. , MacOS and other operating systems. Jianying officially announced the upgrade of its membership system and launched a new SVIP, which includes a variety of AI black technologies, such as intelligent translation, intelligent highlighting, intelligent packaging, digital human synthesis, etc. In terms of price, the monthly fee for clipping SVIP is 79 yuan, the annual fee is 599 yuan (note on this site: equivalent to 49.9 yuan per month), the continuous monthly subscription is 59 yuan per month, and the continuous annual subscription is 499 yuan per year (equivalent to 41.6 yuan per month) . In addition, the cut official also stated that in order to improve the user experience, those who have subscribed to the original VIP

On May 30, Tencent announced a comprehensive upgrade of its Hunyuan model. The App "Tencent Yuanbao" based on the Hunyuan model was officially launched and can be downloaded from Apple and Android app stores. Compared with the Hunyuan applet version in the previous testing stage, Tencent Yuanbao provides core capabilities such as AI search, AI summary, and AI writing for work efficiency scenarios; for daily life scenarios, Yuanbao's gameplay is also richer and provides multiple features. AI application, and new gameplay methods such as creating personal agents are added. "Tencent does not strive to be the first to make large models." Liu Yuhong, vice president of Tencent Cloud and head of Tencent Hunyuan large model, said: "In the past year, we continued to promote the capabilities of Tencent Hunyuan large model. In the rich and massive Polish technology in business scenarios while gaining insights into users’ real needs
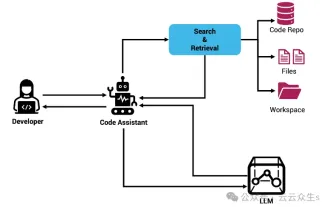
Improve developer productivity, efficiency, and accuracy by incorporating retrieval-enhanced generation and semantic memory into AI coding assistants. Translated from EnhancingAICodingAssistantswithContextUsingRAGandSEM-RAG, author JanakiramMSV. While basic AI programming assistants are naturally helpful, they often fail to provide the most relevant and correct code suggestions because they rely on a general understanding of the software language and the most common patterns of writing software. The code generated by these coding assistants is suitable for solving the problems they are responsible for solving, but often does not conform to the coding standards, conventions and styles of the individual teams. This often results in suggestions that need to be modified or refined in order for the code to be accepted into the application
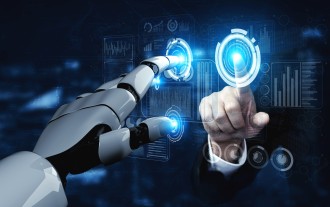
Large Language Models (LLMs) are trained on huge text databases, where they acquire large amounts of real-world knowledge. This knowledge is embedded into their parameters and can then be used when needed. The knowledge of these models is "reified" at the end of training. At the end of pre-training, the model actually stops learning. Align or fine-tune the model to learn how to leverage this knowledge and respond more naturally to user questions. But sometimes model knowledge is not enough, and although the model can access external content through RAG, it is considered beneficial to adapt the model to new domains through fine-tuning. This fine-tuning is performed using input from human annotators or other LLM creations, where the model encounters additional real-world knowledge and integrates it

To learn more about AIGC, please visit: 51CTOAI.x Community https://www.51cto.com/aigc/Translator|Jingyan Reviewer|Chonglou is different from the traditional question bank that can be seen everywhere on the Internet. These questions It requires thinking outside the box. Large Language Models (LLMs) are increasingly important in the fields of data science, generative artificial intelligence (GenAI), and artificial intelligence. These complex algorithms enhance human skills and drive efficiency and innovation in many industries, becoming the key for companies to remain competitive. LLM has a wide range of applications. It can be used in fields such as natural language processing, text generation, speech recognition and recommendation systems. By learning from large amounts of data, LLM is able to generate text
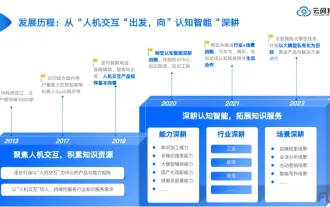
1. Background Introduction First, let’s introduce the development history of Yunwen Technology. Yunwen Technology Company...2023 is the period when large models are prevalent. Many companies believe that the importance of graphs has been greatly reduced after large models, and the preset information systems studied previously are no longer important. However, with the promotion of RAG and the prevalence of data governance, we have found that more efficient data governance and high-quality data are important prerequisites for improving the effectiveness of privatized large models. Therefore, more and more companies are beginning to pay attention to knowledge construction related content. This also promotes the construction and processing of knowledge to a higher level, where there are many techniques and methods that can be explored. It can be seen that the emergence of a new technology does not necessarily defeat all old technologies. It is also possible that the new technology and the old technology will be integrated with each other.
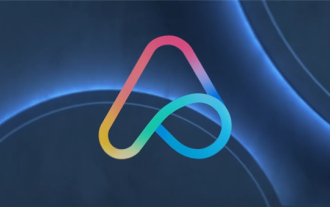
According to news on June 13, according to Byte's "Volcano Engine" public account, Xiaomi's artificial intelligence assistant "Xiao Ai" has reached a cooperation with Volcano Engine. The two parties will achieve a more intelligent AI interactive experience based on the beanbao large model. It is reported that the large-scale beanbao model created by ByteDance can efficiently process up to 120 billion text tokens and generate 30 million pieces of content every day. Xiaomi used the beanbao large model to improve the learning and reasoning capabilities of its own model and create a new "Xiao Ai Classmate", which not only more accurately grasps user needs, but also provides faster response speed and more comprehensive content services. For example, when a user asks about a complex scientific concept, &ldq
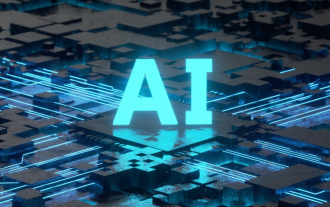
Editor |ScienceAI Question Answering (QA) data set plays a vital role in promoting natural language processing (NLP) research. High-quality QA data sets can not only be used to fine-tune models, but also effectively evaluate the capabilities of large language models (LLM), especially the ability to understand and reason about scientific knowledge. Although there are currently many scientific QA data sets covering medicine, chemistry, biology and other fields, these data sets still have some shortcomings. First, the data form is relatively simple, most of which are multiple-choice questions. They are easy to evaluate, but limit the model's answer selection range and cannot fully test the model's ability to answer scientific questions. In contrast, open-ended Q&A
