


Is the big model taking shortcuts to 'beat the rankings'? The problem of data pollution deserves attention
In the first year of generative AI, everyone’s work pace has become much faster.
Especially this year, everyone is working hard to roll out large models: Recently, domestic and foreign technology giants and startups have taken turns to launch large models. As soon as the press conference started, all of them were major breakthroughs. , each company has refreshed the important Benchmark list, either ranking first or in the first tier.
After being excited about the rapid progress of technology, many people find that there seems to be something wrong: Why does everyone have a share in the top spot in the rankings? What is this mechanism?
As a result, the issue of “ranking cheating” has also begun to attract attention.
Recently, we have noticed that there are more and more discussions in WeChat Moments and Zhihu communities on the issue of "swiping the rankings" of large models. In particular, a post on Zhihu: How do you evaluate the phenomenon that the Tiangong Large Model Technical Report pointed out that many large models use data in the field to boost rankings? It aroused everyone's discussion.
Link: https://www.zhihu.com/question/628957425
Many The large model ranking mechanism exposed
The research comes from Kunlun Wanwei’s “Tiangong” large model research team. They released a technical report in a preprint version of the paper at the end of last month. on the platform arXiv.
Paper link: https://arxiv.org/abs/2310.19341
The paper itself is Introducing Skywork-13B, which is a large language model (LLM) series of Tiangong. The authors introduce a two-stage training method using segmented corpora, targeting general training and domain-specific enhanced training respectively.
As usual with new research on large models, the authors stated that their model not only performed well on popular test benchmarks, but also achieved state-of-the-art results on many Chinese branch tasks. of-art level (the best in the industry).
The point is that the report also verified the real effects of many large models and pointed out that some other large domestic models were suspected of being opportunistic. This is Table 8:
Here, the author wants to verify the mathematical applications of several common large models in the industry. Regarding the degree of overfitting on the problem benchmark GSM8K, GPT-4 was used to generate some samples that were in the same form as GSM8K. The correctness was manually checked, and these models were tested in the generated data set and the original training set and test set of GSM8K. Comparisons were made and losses were calculated. Then there are two more metrics:
Δ1 As an indicator of potential test data leakage during model training, the lower value Indicates a possible leak. Without training on the test set, the value should be zero.
Δ2 measures the degree of overfitting of the training split of the dataset. A higher Δ2 value means overfitting. If it has not been trained on the training set, the value should be zero.
To explain it in simple words: if a model is training, directly use the "real questions" and "answers" in the benchmark test as learning materials, and want to use them to brush up. points, then there will be an exception here.
Okay, the problematic areas of Δ1 and Δ2 are thoughtfully highlighted in gray above.
Netizens commented that someone finally told the open secret of "data set pollution".
Some netizens also said that the intelligence level of large models still depends on zero-shot capabilities, which cannot be achieved by existing test benchmarks.
Picture: Screenshot from Zhihu netizen comments
During the interaction between the author and the readers, the author also expressed the hope that "it will make everyone look at the issue of rankings more rationally. There is still a big gap between many models and GPT4."
Picture: Screenshot from Zhihu article https://zhuanlan.zhihu.com/p/664985891
The problem of data pollution deserves attention
In fact, this is not a temporary phenomenon. Since the introduction of Benchmark, such problems have occurred from time to time, as the title of a very ironic article on arXiv in September this year pointed out: Pretraining on the Test Set Is All You Need.
In addition, a recent formal study by Renmin University and the University of Illinois at Urbana-Champaign also pointed out problems in large model evaluation. The title is very eye-catching "Don't Make Your LLM an Evaluation Benchmark Cheater":
Paper link: https://arxiv.org/abs/ 2311.01964
The paper points out that the current hot field of large models has made people care about the ranking of benchmarks, but its fairness and reliability are being questioned. The main issue is data contamination and leakage, which may be triggered unintentionally because we may not know the future evaluation data set when preparing the pre-training corpus. For example, GPT-3 found that the pre-training corpus contained the Children's Book Test data set, and the LLaMA-2 paper mentioned extracting contextual web content from the BoolQ data set.
Data sets require a lot of effort from many people to collect, organize and label. If a high-quality data set is good enough to be used for evaluation, it may naturally be used by other people. Used for training large models.
On the other hand, when evaluating using existing benchmarks, the results for the large models we evaluated were mostly obtained by running on a local server or through API calls. During this process, any improper means (such as data contamination) that could lead to abnormal improvements in assessment performance were not rigorously examined.
What’s worse is that the detailed composition of the training corpus (such as data sources) is often regarded as the core “secret” of existing large models. This makes it more difficult to explore the problem of data pollution.
In other words, the amount of excellent data is limited, and on many test sets, GPT-4 and Llama-2 are not necessarily the best. no problem. For example, GSM8K was mentioned in the first paper, and GPT-4 mentioned using its training set in the official technical report.
Don’t you say that data is very important? Then, will the performance of a large model that uses “real questions” become better because the training data is better? the answer is negative.
Researchers have experimentally found that benchmark leaks can cause large models to run exaggerated results: for example, a 1.3B model can surpass a model 10 times the size on some tasks. But the side effect is that if we only use this leaked data to fine-tune or train the model, the performance of these large test-specific models on other normal testing tasks may be adversely affected.
Therefore, the author suggests that in the future, when researchers evaluate large models or study new technologies, they should:
- Use more benchmarks from different sources covering basic abilities (e.g. text generation) and advanced abilities (e.g. complex reasoning) to fully assess LLM capabilities.
- When using an evaluation benchmark, it is important to perform data sanitization checks between the pre-training data and any related data (such as training and test sets). In addition, the pollution analysis results for the assessment baseline need to be reported as a reference. If possible, it is recommended to make the detailed composition of the pre-training data public.
- It is recommended that diversified test prompts should be used to reduce the impact of prompt sensitivity. It also makes sense to perform contamination analysis between the baseline data and existing pre-training corpora to alert on any potential contamination risks. For the purpose of evaluation, it is recommended that each submission be accompanied by a special contamination analysis report.
Finally, I would like to say that fortunately, this issue has gradually attracted everyone's attention. Whether it is technical reports, paper research or community discussions, everyone has begun to pay attention to the "swiping the list" of large models. problem.
What are your opinions and effective suggestions on this?
The above is the detailed content of Is the big model taking shortcuts to 'beat the rankings'? The problem of data pollution deserves attention. For more information, please follow other related articles on the PHP Chinese website!

Hot AI Tools

Undresser.AI Undress
AI-powered app for creating realistic nude photos

AI Clothes Remover
Online AI tool for removing clothes from photos.

Undress AI Tool
Undress images for free

Clothoff.io
AI clothes remover

AI Hentai Generator
Generate AI Hentai for free.

Hot Article

Hot Tools

Notepad++7.3.1
Easy-to-use and free code editor

SublimeText3 Chinese version
Chinese version, very easy to use

Zend Studio 13.0.1
Powerful PHP integrated development environment

Dreamweaver CS6
Visual web development tools

SublimeText3 Mac version
God-level code editing software (SublimeText3)

Hot Topics


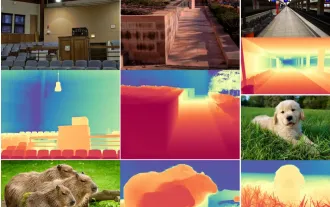
0.What does this article do? We propose DepthFM: a versatile and fast state-of-the-art generative monocular depth estimation model. In addition to traditional depth estimation tasks, DepthFM also demonstrates state-of-the-art capabilities in downstream tasks such as depth inpainting. DepthFM is efficient and can synthesize depth maps within a few inference steps. Let’s read about this work together ~ 1. Paper information title: DepthFM: FastMonocularDepthEstimationwithFlowMatching Author: MingGui, JohannesS.Fischer, UlrichPrestel, PingchuanMa, Dmytr
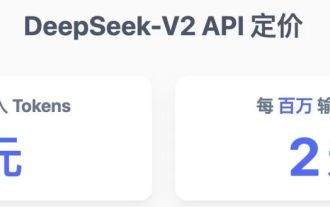
Imagine an artificial intelligence model that not only has the ability to surpass traditional computing, but also achieves more efficient performance at a lower cost. This is not science fiction, DeepSeek-V2[1], the world’s most powerful open source MoE model is here. DeepSeek-V2 is a powerful mixture of experts (MoE) language model with the characteristics of economical training and efficient inference. It consists of 236B parameters, 21B of which are used to activate each marker. Compared with DeepSeek67B, DeepSeek-V2 has stronger performance, while saving 42.5% of training costs, reducing KV cache by 93.3%, and increasing the maximum generation throughput to 5.76 times. DeepSeek is a company exploring general artificial intelligence
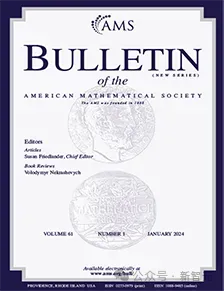
AI is indeed changing mathematics. Recently, Tao Zhexuan, who has been paying close attention to this issue, forwarded the latest issue of "Bulletin of the American Mathematical Society" (Bulletin of the American Mathematical Society). Focusing on the topic "Will machines change mathematics?", many mathematicians expressed their opinions. The whole process was full of sparks, hardcore and exciting. The author has a strong lineup, including Fields Medal winner Akshay Venkatesh, Chinese mathematician Zheng Lejun, NYU computer scientist Ernest Davis and many other well-known scholars in the industry. The world of AI has changed dramatically. You know, many of these articles were submitted a year ago.
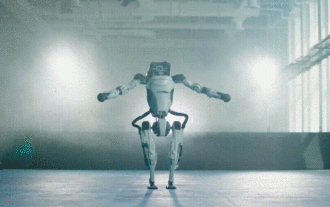
Boston Dynamics Atlas officially enters the era of electric robots! Yesterday, the hydraulic Atlas just "tearfully" withdrew from the stage of history. Today, Boston Dynamics announced that the electric Atlas is on the job. It seems that in the field of commercial humanoid robots, Boston Dynamics is determined to compete with Tesla. After the new video was released, it had already been viewed by more than one million people in just ten hours. The old people leave and new roles appear. This is a historical necessity. There is no doubt that this year is the explosive year of humanoid robots. Netizens commented: The advancement of robots has made this year's opening ceremony look like a human, and the degree of freedom is far greater than that of humans. But is this really not a horror movie? At the beginning of the video, Atlas is lying calmly on the ground, seemingly on his back. What follows is jaw-dropping
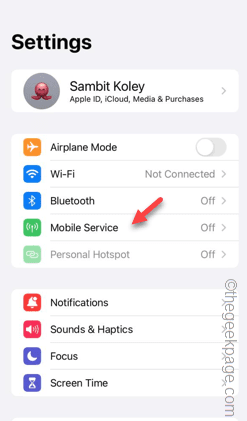
Facing lag, slow mobile data connection on iPhone? Typically, the strength of cellular internet on your phone depends on several factors such as region, cellular network type, roaming type, etc. There are some things you can do to get a faster, more reliable cellular Internet connection. Fix 1 – Force Restart iPhone Sometimes, force restarting your device just resets a lot of things, including the cellular connection. Step 1 – Just press the volume up key once and release. Next, press the Volume Down key and release it again. Step 2 – The next part of the process is to hold the button on the right side. Let the iPhone finish restarting. Enable cellular data and check network speed. Check again Fix 2 – Change data mode While 5G offers better network speeds, it works better when the signal is weaker
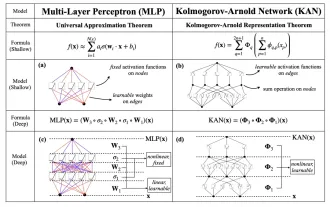
Earlier this month, researchers from MIT and other institutions proposed a very promising alternative to MLP - KAN. KAN outperforms MLP in terms of accuracy and interpretability. And it can outperform MLP running with a larger number of parameters with a very small number of parameters. For example, the authors stated that they used KAN to reproduce DeepMind's results with a smaller network and a higher degree of automation. Specifically, DeepMind's MLP has about 300,000 parameters, while KAN only has about 200 parameters. KAN has a strong mathematical foundation like MLP. MLP is based on the universal approximation theorem, while KAN is based on the Kolmogorov-Arnold representation theorem. As shown in the figure below, KAN has
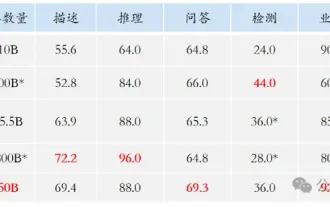
I cry to death. The world is madly building big models. The data on the Internet is not enough. It is not enough at all. The training model looks like "The Hunger Games", and AI researchers around the world are worrying about how to feed these data voracious eaters. This problem is particularly prominent in multi-modal tasks. At a time when nothing could be done, a start-up team from the Department of Renmin University of China used its own new model to become the first in China to make "model-generated data feed itself" a reality. Moreover, it is a two-pronged approach on the understanding side and the generation side. Both sides can generate high-quality, multi-modal new data and provide data feedback to the model itself. What is a model? Awaker 1.0, a large multi-modal model that just appeared on the Zhongguancun Forum. Who is the team? Sophon engine. Founded by Gao Yizhao, a doctoral student at Renmin University’s Hillhouse School of Artificial Intelligence.
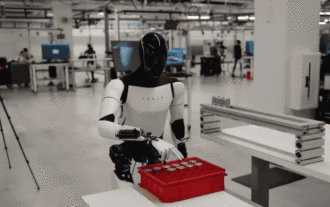
The latest video of Tesla's robot Optimus is released, and it can already work in the factory. At normal speed, it sorts batteries (Tesla's 4680 batteries) like this: The official also released what it looks like at 20x speed - on a small "workstation", picking and picking and picking: This time it is released One of the highlights of the video is that Optimus completes this work in the factory, completely autonomously, without human intervention throughout the process. And from the perspective of Optimus, it can also pick up and place the crooked battery, focusing on automatic error correction: Regarding Optimus's hand, NVIDIA scientist Jim Fan gave a high evaluation: Optimus's hand is the world's five-fingered robot. One of the most dexterous. Its hands are not only tactile
