


A team from the Chinese Academy of Sciences uses AI large model training technology to process massive synchrotron radiation data
Without changing the original meaning, the sentence that needs to be rewritten into Chinese is: Edit| It is a coherent diffraction imaging technology that can theoretically achieve diffraction-limited resolution and has been widely used in research in various scientific fields such as materials, life, semiconductors, and energy.
The new generation of synchrotron radiation light source can provide high coherence and high brightness X-rays, promoting the development of coherent imaging technology in the direction of high-throughput and multi-dimensional, making ptychography useful in fine structure research and functional characterization of large-volume samples It has excellent application prospects. However, new experimental models and application scenarios have brought technical challenges to the online analysis of massive data. The amount of original diffraction pattern data for a single experiment can reach the PB level, becoming one of the largest data sources for scientific experiments on the fourth-generation synchrotron radiation light source. one. In addition, its phase recovery problem is also one of the most difficult problems in the field of synchrotron radiation data processing.
As a powerful tool for big data analysis and processing, artificial intelligence methods maintain the advantages of traditional algorithms and highlight their capabilities in online analysis of massive experimental data.
As a relatively time-consuming scanning imaging technology, one of the main goals of ptychography is to enable real-time analysis. However, it is difficult for the current traditional ptychography reconstruction algorithm to meet the needs of online reconstruction. Based on the convolutional neural network, the research team proposed a grouped convolutional neural network decoder structure, which makes the network training and reconstruction faster and the reconstruction effect better. Neural networks can learn to map from diffraction patterns to real objects. Thanks to the further improvement in the volume and quality of light source data in the future, the network scale, parameter volume, and training data volume will further increase, which will improve the performance and generalization capabilities of the network. The High Energy Synchrotron Radiation Source (HEPS) beamline software team of the Chinese Academy of Sciences has developed a convolutional neural network framework called PtyNet to recover objects from X-ray Ptychography experimental data accurate projection. With the support of powerful computing clusters, PtyNet can quickly obtain data from synchrotron radiation light sources for training, and quickly reconstruct images of users’ experimental data
Figure 1
The research is titled "Efficient ptychography reconstruction strategy by fine-tuning large pre-trained deep learning models" and was published in iScience magazine on November 9, 2023
Paper link:
Since the target objects recovered by different experimental data are different, the team also introduced a fine-tuning strategy Further optimize network parameters. The unsupervised fine-tuning strategy enables the network to have stronger generalization ability and higher reconstruction resolution. Synchrotron radiation sources can provide the network with sufficient data to obtain a more powerful pre-trained model. Even for a new sample that does not appear within the network, the network can be successfully reconstructed (Figure 2).
What needs to be rewritten is: The second picture
In the future, the team will continue to apply convolutional neural networks to X-ray coherence imaging research in the field. Using fine-tuning and large model strategies, a large model of coherent imaging was developed. The model itself can identify different imaging tasks and give recovery results. Users only need to input a few line station parameters for real-time reconstruction.
Facing the challenge of EB-scale data in the future, HEPS is actively promoting the innovative scientific research paradigm of "Large-scale scientific software framework AI for Science" and has established a professional scientific software team to carry out experimental control, large-scale Cross-field research such as data collection and processing, artificial intelligence, cutting-edge subject algorithms, multi-scale image processing and data mining has laid the foundation for the construction of "smart light sources".
The above is the detailed content of A team from the Chinese Academy of Sciences uses AI large model training technology to process massive synchrotron radiation data. For more information, please follow other related articles on the PHP Chinese website!

Hot AI Tools

Undresser.AI Undress
AI-powered app for creating realistic nude photos

AI Clothes Remover
Online AI tool for removing clothes from photos.

Undress AI Tool
Undress images for free

Clothoff.io
AI clothes remover

AI Hentai Generator
Generate AI Hentai for free.

Hot Article

Hot Tools

Notepad++7.3.1
Easy-to-use and free code editor

SublimeText3 Chinese version
Chinese version, very easy to use

Zend Studio 13.0.1
Powerful PHP integrated development environment

Dreamweaver CS6
Visual web development tools

SublimeText3 Mac version
God-level code editing software (SublimeText3)

Hot Topics


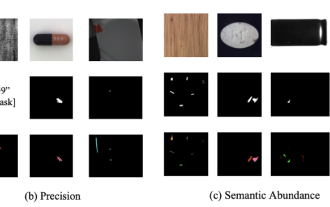
In modern manufacturing, accurate defect detection is not only the key to ensuring product quality, but also the core of improving production efficiency. However, existing defect detection datasets often lack the accuracy and semantic richness required for practical applications, resulting in models unable to identify specific defect categories or locations. In order to solve this problem, a top research team composed of Hong Kong University of Science and Technology Guangzhou and Simou Technology innovatively developed the "DefectSpectrum" data set, which provides detailed and semantically rich large-scale annotation of industrial defects. As shown in Table 1, compared with other industrial data sets, the "DefectSpectrum" data set provides the most defect annotations (5438 defect samples) and the most detailed defect classification (125 defect categories
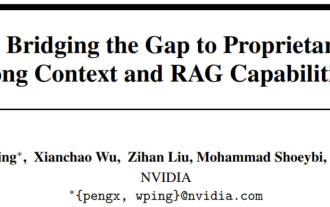
The open LLM community is an era when a hundred flowers bloom and compete. You can see Llama-3-70B-Instruct, QWen2-72B-Instruct, Nemotron-4-340B-Instruct, Mixtral-8x22BInstruct-v0.1 and many other excellent performers. Model. However, compared with proprietary large models represented by GPT-4-Turbo, open models still have significant gaps in many fields. In addition to general models, some open models that specialize in key areas have been developed, such as DeepSeek-Coder-V2 for programming and mathematics, and InternVL for visual-language tasks.
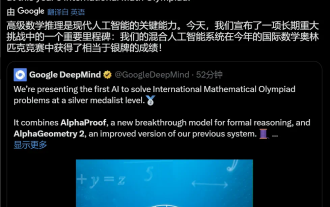
For AI, Mathematical Olympiad is no longer a problem. On Thursday, Google DeepMind's artificial intelligence completed a feat: using AI to solve the real question of this year's International Mathematical Olympiad IMO, and it was just one step away from winning the gold medal. The IMO competition that just ended last week had six questions involving algebra, combinatorics, geometry and number theory. The hybrid AI system proposed by Google got four questions right and scored 28 points, reaching the silver medal level. Earlier this month, UCLA tenured professor Terence Tao had just promoted the AI Mathematical Olympiad (AIMO Progress Award) with a million-dollar prize. Unexpectedly, the level of AI problem solving had improved to this level before July. Do the questions simultaneously on IMO. The most difficult thing to do correctly is IMO, which has the longest history, the largest scale, and the most negative
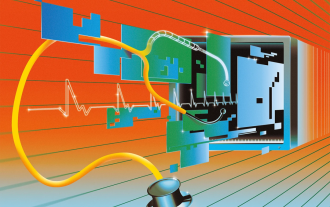
Editor | ScienceAI Based on limited clinical data, hundreds of medical algorithms have been approved. Scientists are debating who should test the tools and how best to do so. Devin Singh witnessed a pediatric patient in the emergency room suffer cardiac arrest while waiting for treatment for a long time, which prompted him to explore the application of AI to shorten wait times. Using triage data from SickKids emergency rooms, Singh and colleagues built a series of AI models that provide potential diagnoses and recommend tests. One study showed that these models can speed up doctor visits by 22.3%, speeding up the processing of results by nearly 3 hours per patient requiring a medical test. However, the success of artificial intelligence algorithms in research only verifies this
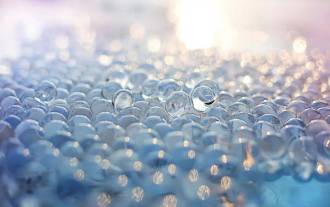
Editor |KX To this day, the structural detail and precision determined by crystallography, from simple metals to large membrane proteins, are unmatched by any other method. However, the biggest challenge, the so-called phase problem, remains retrieving phase information from experimentally determined amplitudes. Researchers at the University of Copenhagen in Denmark have developed a deep learning method called PhAI to solve crystal phase problems. A deep learning neural network trained using millions of artificial crystal structures and their corresponding synthetic diffraction data can generate accurate electron density maps. The study shows that this deep learning-based ab initio structural solution method can solve the phase problem at a resolution of only 2 Angstroms, which is equivalent to only 10% to 20% of the data available at atomic resolution, while traditional ab initio Calculation
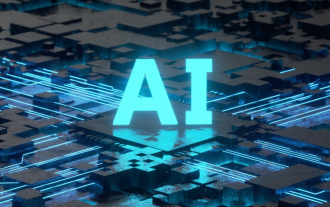
Editor |ScienceAI Question Answering (QA) data set plays a vital role in promoting natural language processing (NLP) research. High-quality QA data sets can not only be used to fine-tune models, but also effectively evaluate the capabilities of large language models (LLM), especially the ability to understand and reason about scientific knowledge. Although there are currently many scientific QA data sets covering medicine, chemistry, biology and other fields, these data sets still have some shortcomings. First, the data form is relatively simple, most of which are multiple-choice questions. They are easy to evaluate, but limit the model's answer selection range and cannot fully test the model's ability to answer scientific questions. In contrast, open-ended Q&A
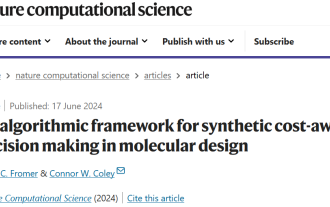
Editor | Ziluo AI’s use in streamlining drug discovery is exploding. Screen billions of candidate molecules for those that may have properties needed to develop new drugs. There are so many variables to consider, from material prices to the risk of error, that weighing the costs of synthesizing the best candidate molecules is no easy task, even if scientists use AI. Here, MIT researchers developed SPARROW, a quantitative decision-making algorithm framework, to automatically identify the best molecular candidates, thereby minimizing synthesis costs while maximizing the likelihood that the candidates have the desired properties. The algorithm also determined the materials and experimental steps needed to synthesize these molecules. SPARROW takes into account the cost of synthesizing a batch of molecules at once, since multiple candidate molecules are often available
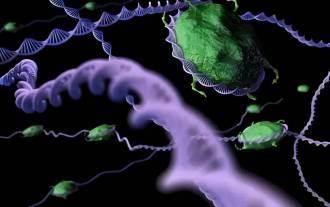
Editor | KX In the field of drug research and development, accurately and effectively predicting the binding affinity of proteins and ligands is crucial for drug screening and optimization. However, current studies do not take into account the important role of molecular surface information in protein-ligand interactions. Based on this, researchers from Xiamen University proposed a novel multi-modal feature extraction (MFE) framework, which for the first time combines information on protein surface, 3D structure and sequence, and uses a cross-attention mechanism to compare different modalities. feature alignment. Experimental results demonstrate that this method achieves state-of-the-art performance in predicting protein-ligand binding affinities. Furthermore, ablation studies demonstrate the effectiveness and necessity of protein surface information and multimodal feature alignment within this framework. Related research begins with "S
