Sparse4D v3 is here! Advancing end-to-end 3D detection and tracking
New title: Sparse4D v3: Advancing end-to-end 3D detection and tracking technology
Paper link: https://arxiv.org/pdf/2311.11722.pdf
Needs to be rewritten The content is: Code link: https://github.com/linxuewu/Sparse4D
Rewritten content: The author’s affiliation is Horizon Company
Thesis idea:
In the autonomous driving perception system, 3D detection and tracking are two basic tasks. This article takes a deeper look into this area based on the Sparse4D framework. This article introduces two auxiliary training tasks (temporal instance denoising-Temporal Instance Denoising and quality estimation-Quality Estimation), and proposes decoupled attention (decoupled attention) for structural improvement, thereby significantly improving detection performance. Furthermore, this paper extends the detector to the tracker using a simple method that assigns instance IDs during inference, further highlighting the advantages of query-based algorithms. Extensive experiments on the nuScenes benchmark validate the effectiveness of the proposed improvements. Using ResNet50 as the backbone, mAP, NDS and AMOTA increased by 3.0%, 2.2% and 7.6% respectively, reaching 46.9%, 56.1% and 49.0% respectively. The best model in this article achieved 71.9% NDS and 67.7% AMOTA on the nuScenes test set
Main contribution:
Sparse4D-v3 is a powerful 3D perception framework , which proposes three effective strategies: temporal instance denoising, quality estimation, and decoupled attention
This article extends Sparse4D into an end-to-end tracking model.
This paper demonstrates the effectiveness of nuScenes improvements, achieving state-of-the-art performance in detection and tracking tasks.
Network Design:
First, it is observed that sparse algorithms face greater challenges in convergence compared to dense algorithms, thus affecting the final performance. This problem has been well studied in the field of 2D detection [17, 48, 53], mainly because sparse algorithms use one-to-one positive sample matching. This matching method is unstable in the early stages of training, and compared with one-to-many matching, the number of positive samples is limited, thus reducing the efficiency of decoder training. Furthermore, Sparse4D uses sparse feature sampling instead of global cross-attention, which further hinders the convergence of the encoder due to the scarcity of positive samples. In Sparse4Dv2, dense deep supervision is introduced to partially alleviate these convergence issues faced by image encoders. The main goal of this paper is to enhance model performance by focusing on the stability of decoder training. This paper uses the denoising task as auxiliary supervision and extends the denoising technology from 2D single frame detection to 3D temporal detection. This not only ensures stable positive sample matching, but also significantly increases the number of positive samples. In addition, this paper also introduces a quality assessment task as auxiliary supervision. This makes the output confidence score more reasonable, improves the accuracy of detection result ranking, and thus obtains higher evaluation indicators. In addition, this article improves the structure of the instance self-attention and temporal cross-attention modules in Sparse4D, and introduces a decoupled attention mechanism aimed at reducing feature interference in the attention weight calculation process. By using anchor embeddings and instance features as inputs to the attention calculation, instances with outliers in the attention weights can be reduced. This can more accurately reflect the correlation between target features, thereby achieving correct feature aggregation. This paper uses connections instead of attention mechanisms to significantly reduce this error. This augmentation method has similarities with conditional DETR, but the key difference is that this paper emphasizes attention between queries, while conditional DETR focuses on cross-attention between queries and image features. In addition, this article also involves a unique encoding method
In order to improve the end-to-end capabilities of the perception system, this article studies the method of integrating 3D multi-target tracking tasks into the Sparse4D framework to directly output the target's motion trajectory. Unlike detection-based tracking methods, this paper integrates all tracking functions into the detector by eliminating the need for data association and filtering. Furthermore, unlike existing joint detection and tracking methods, our tracker does not require modification or adjustment of the loss function during training. It does not require providing ground truth IDs, but implements predefined instance-to-track regression. The tracking implementation of this article fully integrates the detector and the tracker, without modifying the training process of the detector, and without additional fine-tuning
This is Figure 1 about the overview of the Sparse4D framework , the input is a multi-view video, and the output is the perceptual result of all frames
Figure 2: Inference efficiency (FPS) - perceptual performance (FPS) on the nuScenes validation data set of different algorithms mAP).
Figure 3: Visualization of attention weights in instance self-attention: 1) The first row shows the attention weights in ordinary self-attention, where the pedestrian in the red circle is shown to be in line with the target vehicle (green box) unexpected correlation. 2) The second row shows the attention weight in decoupled attention, which effectively solves this problem.
The fourth picture shows an example of time series instance denoising. During the training phase, instances consist of two parts: learnable and noisy. Noise instances are composed of temporal and non-temporal elements. This paper adopts a pre-matching method to allocate positive and negative samples, that is, matching anchors with ground truth, while learnable instances are matched with predictions and ground truth. During the testing phase, only green blocks remain. In order to prevent features from spreading between groups, an Attention mask is used. Gray indicates that there is no attention between queries and keys, and green indicates the opposite.
Please see Figure 5: Anchor points Architectures for encoders and attention. This paper independently encodes high-dimensional features of multiple components of anchors and then concatenates them. This approach reduces computational and parameter overhead compared to the original Sparse4D. E and F represent anchor embedding and instance features respectively
Experimental results:
Summary:
This article first proposes a method to enhance the detection performance of Sparse4D. This enhancement mainly includes three aspects: temporal instance denoising, quality estimation and decoupled attention. Subsequently, the article explains the process of extending Sparse4D into an end-to-end tracking model. This article's experiments on nuScenes show that these enhancements significantly improve performance, placing Sparse4Dv3 at the forefront of the field.
Citation:
Lin, X., Pei, Z., Lin, T., Huang, L., & Su, Z. (2023). Sparse4D v3: Advancing End-to-End 3D Detection and Tracking. ArXiv. /abs/2311.11722
The above is the detailed content of Sparse4D v3 is here! Advancing end-to-end 3D detection and tracking. For more information, please follow other related articles on the PHP Chinese website!

Hot AI Tools

Undresser.AI Undress
AI-powered app for creating realistic nude photos

AI Clothes Remover
Online AI tool for removing clothes from photos.

Undress AI Tool
Undress images for free

Clothoff.io
AI clothes remover

Video Face Swap
Swap faces in any video effortlessly with our completely free AI face swap tool!

Hot Article

Hot Tools

Notepad++7.3.1
Easy-to-use and free code editor

SublimeText3 Chinese version
Chinese version, very easy to use

Zend Studio 13.0.1
Powerful PHP integrated development environment

Dreamweaver CS6
Visual web development tools

SublimeText3 Mac version
God-level code editing software (SublimeText3)

Hot Topics


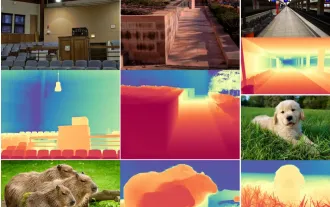
0.What does this article do? We propose DepthFM: a versatile and fast state-of-the-art generative monocular depth estimation model. In addition to traditional depth estimation tasks, DepthFM also demonstrates state-of-the-art capabilities in downstream tasks such as depth inpainting. DepthFM is efficient and can synthesize depth maps within a few inference steps. Let’s read about this work together ~ 1. Paper information title: DepthFM: FastMonocularDepthEstimationwithFlowMatching Author: MingGui, JohannesS.Fischer, UlrichPrestel, PingchuanMa, Dmytr
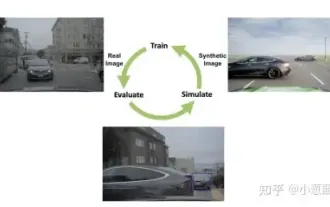
Yesterday during the interview, I was asked whether I had done any long-tail related questions, so I thought I would give a brief summary. The long-tail problem of autonomous driving refers to edge cases in autonomous vehicles, that is, possible scenarios with a low probability of occurrence. The perceived long-tail problem is one of the main reasons currently limiting the operational design domain of single-vehicle intelligent autonomous vehicles. The underlying architecture and most technical issues of autonomous driving have been solved, and the remaining 5% of long-tail problems have gradually become the key to restricting the development of autonomous driving. These problems include a variety of fragmented scenarios, extreme situations, and unpredictable human behavior. The "long tail" of edge scenarios in autonomous driving refers to edge cases in autonomous vehicles (AVs). Edge cases are possible scenarios with a low probability of occurrence. these rare events
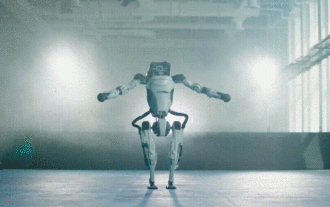
Boston Dynamics Atlas officially enters the era of electric robots! Yesterday, the hydraulic Atlas just "tearfully" withdrew from the stage of history. Today, Boston Dynamics announced that the electric Atlas is on the job. It seems that in the field of commercial humanoid robots, Boston Dynamics is determined to compete with Tesla. After the new video was released, it had already been viewed by more than one million people in just ten hours. The old people leave and new roles appear. This is a historical necessity. There is no doubt that this year is the explosive year of humanoid robots. Netizens commented: The advancement of robots has made this year's opening ceremony look like a human, and the degree of freedom is far greater than that of humans. But is this really not a horror movie? At the beginning of the video, Atlas is lying calmly on the ground, seemingly on his back. What follows is jaw-dropping
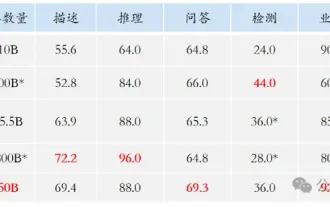
I cry to death. The world is madly building big models. The data on the Internet is not enough. It is not enough at all. The training model looks like "The Hunger Games", and AI researchers around the world are worrying about how to feed these data voracious eaters. This problem is particularly prominent in multi-modal tasks. At a time when nothing could be done, a start-up team from the Department of Renmin University of China used its own new model to become the first in China to make "model-generated data feed itself" a reality. Moreover, it is a two-pronged approach on the understanding side and the generation side. Both sides can generate high-quality, multi-modal new data and provide data feedback to the model itself. What is a model? Awaker 1.0, a large multi-modal model that just appeared on the Zhongguancun Forum. Who is the team? Sophon engine. Founded by Gao Yizhao, a doctoral student at Renmin University’s Hillhouse School of Artificial Intelligence.
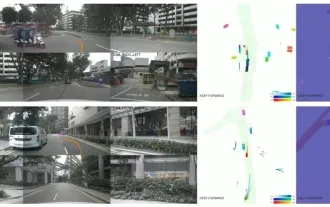
In the past month, due to some well-known reasons, I have had very intensive exchanges with various teachers and classmates in the industry. An inevitable topic in the exchange is naturally end-to-end and the popular Tesla FSDV12. I would like to take this opportunity to sort out some of my thoughts and opinions at this moment for your reference and discussion. How to define an end-to-end autonomous driving system, and what problems should be expected to be solved end-to-end? According to the most traditional definition, an end-to-end system refers to a system that inputs raw information from sensors and directly outputs variables of concern to the task. For example, in image recognition, CNN can be called end-to-end compared to the traditional feature extractor + classifier method. In autonomous driving tasks, input data from various sensors (camera/LiDAR
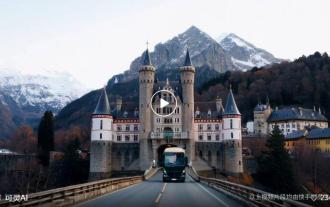
What? Is Zootopia brought into reality by domestic AI? Exposed together with the video is a new large-scale domestic video generation model called "Keling". Sora uses a similar technical route and combines a number of self-developed technological innovations to produce videos that not only have large and reasonable movements, but also simulate the characteristics of the physical world and have strong conceptual combination capabilities and imagination. According to the data, Keling supports the generation of ultra-long videos of up to 2 minutes at 30fps, with resolutions up to 1080p, and supports multiple aspect ratios. Another important point is that Keling is not a demo or video result demonstration released by the laboratory, but a product-level application launched by Kuaishou, a leading player in the short video field. Moreover, the main focus is to be pragmatic, not to write blank checks, and to go online as soon as it is released. The large model of Ke Ling is already available in Kuaiying.
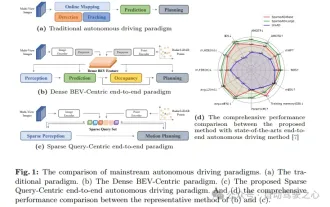
Written in front & starting point The end-to-end paradigm uses a unified framework to achieve multi-tasking in autonomous driving systems. Despite the simplicity and clarity of this paradigm, the performance of end-to-end autonomous driving methods on subtasks still lags far behind single-task methods. At the same time, the dense bird's-eye view (BEV) features widely used in previous end-to-end methods make it difficult to scale to more modalities or tasks. A sparse search-centric end-to-end autonomous driving paradigm (SparseAD) is proposed here, in which sparse search fully represents the entire driving scenario, including space, time, and tasks, without any dense BEV representation. Specifically, a unified sparse architecture is designed for task awareness including detection, tracking, and online mapping. In addition, heavy
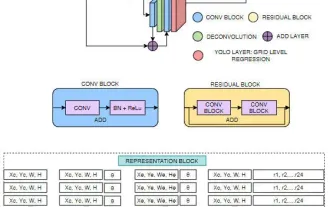
Target detection is a relatively mature problem in autonomous driving systems, among which pedestrian detection is one of the earliest algorithms to be deployed. Very comprehensive research has been carried out in most papers. However, distance perception using fisheye cameras for surround view is relatively less studied. Due to large radial distortion, standard bounding box representation is difficult to implement in fisheye cameras. To alleviate the above description, we explore extended bounding box, ellipse, and general polygon designs into polar/angular representations and define an instance segmentation mIOU metric to analyze these representations. The proposed model fisheyeDetNet with polygonal shape outperforms other models and simultaneously achieves 49.5% mAP on the Valeo fisheye camera dataset for autonomous driving
