


LLMLingua: Integrate LlamaIndex, compress hints and provide efficient large language model inference services
The emergence of large language models (LLM) has stimulated innovation in multiple fields. However, the increasing complexity of prompts, driven by strategies such as chain-of-thought (CoT) prompts and contextual learning (ICL), poses computational challenges. These lengthy prompts require significant resources for reasoning and therefore require efficient solutions. This article will introduce the integration of LLMLingua with the proprietary LlamaIndex to perform efficient inference
LLMLingua is a paper published by Microsoft researchers at EMNLP 2023. LongLLMLingua is a method to enhance llm's ability to perceive key information in long context scenarios through fast compression.
LLMLingua working with llamindex
LLMLingua emerged as a pioneering solution to verbose prompts in LLM applications. This approach focuses on compressing lengthy prompts while ensuring semantic integrity and increasing inference speed. It combines various compression strategies to provide a subtle way to balance hint length and computational efficiency.
The following are the advantages of integrating LLMLingua with LlamaIndex:
The integration of LLMLingua and LlamaIndex marks an important step for llm in rapid optimization step. LlamaIndex is a specialized repository containing pre-optimized hints tailored for a variety of LLM applications, and through this integration LLMLingua can access a rich set of domain-specific, fine-tuned hints, thereby enhancing its hint compression capabilities.
LLMLingua improves the efficiency of LLM applications through synergy with LlamaIndex’s optimization hint library. Leveraging LLAMA's specialized cues, LLMLingua can fine-tune its compression strategy to ensure domain-specific context is preserved while reducing the length of the cues. This collaboration dramatically speeds up inference while preserving key domain nuances
LLMLingua’s integration with LlamaIndex extends its impact on large-scale LLM applications. By leveraging LLAMA's expert tips, LLMLingua has optimized its compression technology, reducing the computational burden of processing lengthy tips. This integration not only accelerates inference but also ensures the retention of critical domain-specific information.
The workflow of LLMLingua and LlamaIndex
Using LlamaIndex to implement LLMLlingua requires a series of structured steps Process, which includes the use of specialized hint libraries for efficient hint compression and enhanced inference speed
#1. Framework integration
Required first Establish a connection between LLMLingua and LlamaIndex. This includes access rights, API configuration and establishing connections for timely retrieval.
2. Retrieval of pre-optimized hints
LlamaIndex is available as a specialized repository containing content tailored for various LLM applications Pre-optimization tips. LLMLingua can access this repository, retrieve domain-specific hints, and utilize these hints for compression
3. Tip Compression Technology
LLMLingua uses its hint compression method to simplify the retrieved hints. These techniques focus on compressing lengthy prompts while ensuring semantic consistency, thereby increasing inference speed without affecting context or relevance.
4. Fine-tune the compression strategy
LLMLingua fine-tunes its compression strategy based on specialized hints obtained from LlamaIndex. This refinement process ensures that domain-specific nuances are preserved while efficiently reducing prompt length.
5. Execution and reasoning
After using LLMLingua’s customized strategy and combining it with LlamaIndex’s pre-optimization prompts for compression, the obtained prompts can be used for LLM inference tasks. At this stage, we perform compression hints within the LLM framework to enable efficient context-aware reasoning
6. Iterative improvements and enhancements
Code implementation continues to undergo iterative refinement. This process includes improving the compression algorithm, optimizing retrieval of hints from LlamaIndex, and fine-tuning the integration to ensure consistency and enhanced performance of compressed hints and LLM inference.
7. Testing and verification
If necessary, testing and verification can be performed so that the efficiency and effectiveness of the integration of LLMLingua and LlamaIndex can be evaluated . Performance metrics are evaluated to ensure that compression hints maintain semantic integrity and increase inference speed without compromising accuracy.
Code implementation
We will start to delve into the code implementation of LLMLingua and LlamaIndex
Installation Package:
# Install dependency. !pip install llmlingua llama-index openai tiktoken -q # Using the OAI import openai openai.api_key = "<insert_openai_key>"</insert_openai_key>
Get data:
!wget "https://www.dropbox.com/s/f6bmb19xdg0xedm/paul_graham_essay.txt?dl=1" -O paul_graham_essay.txt
Load model:
from llama_index import (VectorStoreIndex,SimpleDirectoryReader,load_index_from_storage,StorageContext, ) # load documents documents = SimpleDirectoryReader(input_files=["paul_graham_essay.txt"] ).load_data()
Vector storage :
index = VectorStoreIndex.from_documents(documents) retriever = index.as_retriever(similarity_top_k=10) question = "Where did the author go for art school?" # Ground-truth Answer answer = "RISD" contexts = retriever.retrieve(question) contexts = retriever.retrieve(question) context_list = [n.get_content() for n in contexts] len(context_list) #Output #10
Original prompt and return
# The response from original prompt from llama_index.llms import OpenAI llm = OpenAI(model="gpt-3.5-turbo-16k") prompt = "\n\n".join(context_list + [question]) response = llm.complete(prompt) print(str(response)) #Output The author went to the Rhode Island School of Design (RISD) for art school.
Set LLMLingua
from llama_index.query_engine import RetrieverQueryEngine from llama_index.response_synthesizers import CompactAndRefine from llama_index.indices.postprocessor import LongLLMLinguaPostprocessor node_postprocessor = LongLLMLinguaPostprocessor(instruction_str="Given the context, please answer the final question",target_token=300,rank_method="longllmlingua",additional_compress_kwargs={"condition_compare": True,"condition_in_question": "after","context_budget": "+100","reorder_context": "sort", # enable document reorder,"dynamic_context_compression_ratio": 0.3,}, )
通过LLMLingua进行压缩
retrieved_nodes = retriever.retrieve(question) synthesizer = CompactAndRefine() from llama_index.indices.query.schema import QueryBundle # postprocess (compress), synthesize new_retrieved_nodes = node_postprocessor.postprocess_nodes(retrieved_nodes, query_bundle=QueryBundle(query_str=question) ) original_contexts = "\n\n".join([n.get_content() for n in retrieved_nodes]) compressed_contexts = "\n\n".join([n.get_content() for n in new_retrieved_nodes]) original_tokens = node_postprocessor._llm_lingua.get_token_length(original_contexts) compressed_tokens = node_postprocessor._llm_lingua.get_token_length(compressed_contexts)
打印2个结果对比:
print(compressed_contexts) print() print("Original Tokens:", original_tokens) print("Compressed Tokens:", compressed_tokens) print("Comressed Ratio:", f"{original_tokens/(compressed_tokens + 1e-5):.2f}x")
打印的结果如下:
next Rtm's advice hadn' included anything that. I wanted to do something completely different, so I decided I'd paint. I wanted to how good I could get if I focused on it. the day after stopped on YC, I painting. I was rusty and it took a while to get back into shape, but it was at least completely engaging.1] I wanted to back RISD, was now broke and RISD was very expensive so decided job for a year and return RISD the fall. I got one at Interleaf, which made software for creating documents. You like Microsoft Word? Exactly That was I low end software tends to high. Interleaf still had a few years to live yet. [] the Accademia wasn't, and my money was running out, end year back to thelot the color class I tookD, but otherwise I was basically myself to do that for in993 I dropped I aroundidence bit then my friend Par did me a big A rent-partment building New York. Did I want it Itt more my place, and York be where the artists. wanted [For when you that ofs you big painting of this type hanging in the apartment of a hedge fund manager, you know he paid millions of dollars for it. That's not always why artists have a signature style, but it's usually why buyers pay a lot for such work. [6] Original Tokens: 10719 Compressed Tokens: 308 Comressed Ratio: 34.80x
验证输出:
response = synthesizer.synthesize(question, new_retrieved_nodes) print(str(response)) #Output #The author went to RISD for art school.
总结
LLMLingua与LlamaIndex的集成证明了协作关系在优化大型语言模型(LLM)应用程序方面的变革潜力。这种协作彻底改变了即时压缩方法和推理效率,为上下文感知、简化的LLM应用程序铺平了道路。
这种集成不仅可以提升推理速度,而且可以保证在压缩提示中保持语义的完整性。通过对基于LlamaIndex特定领域提示的压缩策略进行微调,我们平衡了提示长度的减少和基本上下文的保留,从而提高了LLM推理的准确性
从本质上讲,LLMLingua与LlamaIndex的集成超越了传统的提示压缩方法,为未来大型语言模型应用程序的优化、上下文准确和有效地针对不同领域进行定制奠定了基础。这种协作集成预示着大型语言模型应用程序领域中效率和精细化的新时代的到来。
The above is the detailed content of LLMLingua: Integrate LlamaIndex, compress hints and provide efficient large language model inference services. For more information, please follow other related articles on the PHP Chinese website!

Hot AI Tools

Undresser.AI Undress
AI-powered app for creating realistic nude photos

AI Clothes Remover
Online AI tool for removing clothes from photos.

Undress AI Tool
Undress images for free

Clothoff.io
AI clothes remover

Video Face Swap
Swap faces in any video effortlessly with our completely free AI face swap tool!

Hot Article

Hot Tools

Notepad++7.3.1
Easy-to-use and free code editor

SublimeText3 Chinese version
Chinese version, very easy to use

Zend Studio 13.0.1
Powerful PHP integrated development environment

Dreamweaver CS6
Visual web development tools

SublimeText3 Mac version
God-level code editing software (SublimeText3)

Hot Topics


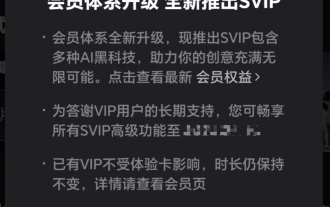
This site reported on June 27 that Jianying is a video editing software developed by FaceMeng Technology, a subsidiary of ByteDance. It relies on the Douyin platform and basically produces short video content for users of the platform. It is compatible with iOS, Android, and Windows. , MacOS and other operating systems. Jianying officially announced the upgrade of its membership system and launched a new SVIP, which includes a variety of AI black technologies, such as intelligent translation, intelligent highlighting, intelligent packaging, digital human synthesis, etc. In terms of price, the monthly fee for clipping SVIP is 79 yuan, the annual fee is 599 yuan (note on this site: equivalent to 49.9 yuan per month), the continuous monthly subscription is 59 yuan per month, and the continuous annual subscription is 499 yuan per year (equivalent to 41.6 yuan per month) . In addition, the cut official also stated that in order to improve the user experience, those who have subscribed to the original VIP
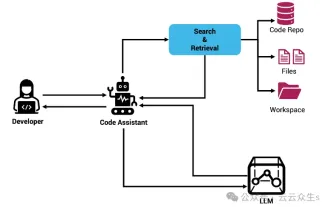
Improve developer productivity, efficiency, and accuracy by incorporating retrieval-enhanced generation and semantic memory into AI coding assistants. Translated from EnhancingAICodingAssistantswithContextUsingRAGandSEM-RAG, author JanakiramMSV. While basic AI programming assistants are naturally helpful, they often fail to provide the most relevant and correct code suggestions because they rely on a general understanding of the software language and the most common patterns of writing software. The code generated by these coding assistants is suitable for solving the problems they are responsible for solving, but often does not conform to the coding standards, conventions and styles of the individual teams. This often results in suggestions that need to be modified or refined in order for the code to be accepted into the application
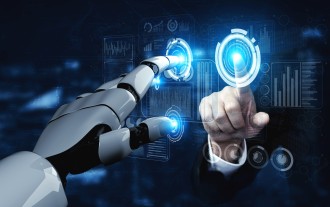
Large Language Models (LLMs) are trained on huge text databases, where they acquire large amounts of real-world knowledge. This knowledge is embedded into their parameters and can then be used when needed. The knowledge of these models is "reified" at the end of training. At the end of pre-training, the model actually stops learning. Align or fine-tune the model to learn how to leverage this knowledge and respond more naturally to user questions. But sometimes model knowledge is not enough, and although the model can access external content through RAG, it is considered beneficial to adapt the model to new domains through fine-tuning. This fine-tuning is performed using input from human annotators or other LLM creations, where the model encounters additional real-world knowledge and integrates it

To learn more about AIGC, please visit: 51CTOAI.x Community https://www.51cto.com/aigc/Translator|Jingyan Reviewer|Chonglou is different from the traditional question bank that can be seen everywhere on the Internet. These questions It requires thinking outside the box. Large Language Models (LLMs) are increasingly important in the fields of data science, generative artificial intelligence (GenAI), and artificial intelligence. These complex algorithms enhance human skills and drive efficiency and innovation in many industries, becoming the key for companies to remain competitive. LLM has a wide range of applications. It can be used in fields such as natural language processing, text generation, speech recognition and recommendation systems. By learning from large amounts of data, LLM is able to generate text
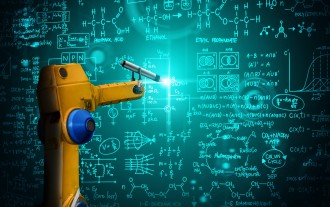
Machine learning is an important branch of artificial intelligence that gives computers the ability to learn from data and improve their capabilities without being explicitly programmed. Machine learning has a wide range of applications in various fields, from image recognition and natural language processing to recommendation systems and fraud detection, and it is changing the way we live. There are many different methods and theories in the field of machine learning, among which the five most influential methods are called the "Five Schools of Machine Learning". The five major schools are the symbolic school, the connectionist school, the evolutionary school, the Bayesian school and the analogy school. 1. Symbolism, also known as symbolism, emphasizes the use of symbols for logical reasoning and expression of knowledge. This school of thought believes that learning is a process of reverse deduction, through existing
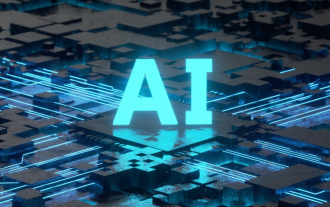
Editor |ScienceAI Question Answering (QA) data set plays a vital role in promoting natural language processing (NLP) research. High-quality QA data sets can not only be used to fine-tune models, but also effectively evaluate the capabilities of large language models (LLM), especially the ability to understand and reason about scientific knowledge. Although there are currently many scientific QA data sets covering medicine, chemistry, biology and other fields, these data sets still have some shortcomings. First, the data form is relatively simple, most of which are multiple-choice questions. They are easy to evaluate, but limit the model's answer selection range and cannot fully test the model's ability to answer scientific questions. In contrast, open-ended Q&A
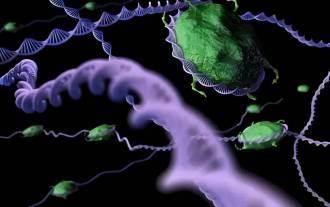
Editor | KX In the field of drug research and development, accurately and effectively predicting the binding affinity of proteins and ligands is crucial for drug screening and optimization. However, current studies do not take into account the important role of molecular surface information in protein-ligand interactions. Based on this, researchers from Xiamen University proposed a novel multi-modal feature extraction (MFE) framework, which for the first time combines information on protein surface, 3D structure and sequence, and uses a cross-attention mechanism to compare different modalities. feature alignment. Experimental results demonstrate that this method achieves state-of-the-art performance in predicting protein-ligand binding affinities. Furthermore, ablation studies demonstrate the effectiveness and necessity of protein surface information and multimodal feature alignment within this framework. Related research begins with "S
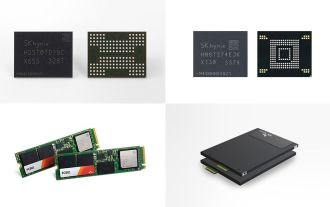
According to news from this site on August 1, SK Hynix released a blog post today (August 1), announcing that it will attend the Global Semiconductor Memory Summit FMS2024 to be held in Santa Clara, California, USA from August 6 to 8, showcasing many new technologies. generation product. Introduction to the Future Memory and Storage Summit (FutureMemoryandStorage), formerly the Flash Memory Summit (FlashMemorySummit) mainly for NAND suppliers, in the context of increasing attention to artificial intelligence technology, this year was renamed the Future Memory and Storage Summit (FutureMemoryandStorage) to invite DRAM and storage vendors and many more players. New product SK hynix launched last year
