


FlashOcc: New ideas for occupancy prediction, new SOTA in accuracy, efficiency and memory usage!
Original title: FlashOcc: Fast and Memory-Efficient Occupancy Prediction via Channel-to-Height Plugin
Paper link: https://arxiv.org/pdf/2311.12058.pdf
Author unit: Dalian University of Technology Houmo AI University of Adelaide
Thesis idea:
In view of being able to alleviate the problem of 3D target detection Occupancy prediction has become a key component of autonomous driving systems due to the ubiquitous long-tail shortcomings and missing capabilities of complex shapes. However, the processing of three-dimensional voxel-level representations inevitably introduces significant overhead in terms of memory and computation, hindering the deployment of occupancy prediction methods to date. Contrary to the trend of making models larger and more complex, this paper argues that an ideal framework should be deployment-friendly across different chips while maintaining high accuracy. To this end, this paper proposes a plug-and-play paradigm, FlashOCC, to consolidate fast and memory-efficient occupancy prediction while maintaining high accuracy. In particular, our FlashOCC makes two improvements based on contemporary voxel-level occupancy prediction methods. First, features are preserved in BEV, enabling the use of efficient 2D convolutional layers for feature extraction. Secondly, channel-to-height transformation is introduced to promote the output logits of BEV to 3D space. This paper applies FlashOCC to various occupancy prediction baselines on the challenging Occ3D-nuScenes benchmark and conducts extensive experiments to verify its effectiveness. Results confirm that our plug-and-play paradigm outperforms previous state-of-the-art methods in terms of accuracy, runtime efficiency, and memory cost, demonstrating its deployment potential. The code will be available for use.
Network design:
Inspired by sub-pixel convolution technology [26], we replace image upsampling with channel rearrangement to achieve channel-to-space Feature transformation. In this study, we aim to achieve channel-to-height feature conversion efficiently. Considering the development of BEV perception tasks, where each pixel in the BEV representation contains information about the corresponding columnar object in the height dimension, we intuitively utilize channel-to-height transformation to flatten the BEV features. Reshape into 3D voxel-level occupancy logits. Therefore, our research focuses on enhancing existing models in a generic and plug-and-play manner rather than developing novel model architectures, as shown in Figure 1(a). Specifically, we directly use 2D convolutions instead of 3D convolutions in contemporary methods, and replace the occupancy logits derived from the 3D convolution outputs with channel-to-height transformations of BEV-level features obtained through 2D convolutions. These models not only achieve the best trade-off between accuracy and time consumption, but also demonstrate excellent deployment compatibility
FlashOcc successfully completed real-time look-around 3D occupancy prediction with extremely high accuracy, representing seminal contributions in this field. Furthermore, it demonstrates enhanced versatility for deployment across different vehicular platforms as it does not require expensive voxel-level feature processing, where view transformers or 3D (deformable) convolution operators are avoided. As shown in Figure 2, the input data of FlashOcc consists of surround images, while the output is dense occupancy prediction results. Although FlashOcc in this article focuses on enhancing existing models in a versatile and plug-and-play manner, it can still be divided into five basic modules: (1) 2D image encoder, responsible for extracting image features from multi-camera images. (2) A view transformation module that helps map 2D perceptual view image features to 3D BEV representations. (3) BEV encoder, responsible for processing BEV feature information. (4) Occupy the prediction module to predict the segmentation label of each voxel. (5) An optional temporal fusion module designed to integrate historical information to improve performance.
Figure 1.(a) illustrates how the proposed FlashOcc can be implemented in a plug-and-play manner. Modern methods use voxel-level 3D features processed by 3D-Conv to predict occupancy. In contrast, our plug-in replacement model is implemented by (1) replacing 3D-Conv with 2D-Conv and (2) replacing the occupancy logits derived from 3D-Conv with channel-to-height transformation. Fast and memory-efficient occupancy prediction of BEV-level features obtained via 2D-Conv. The abbreviation "Conv" stands for convolution. (b) illustrates the trade-off between accuracy and factors such as speed, inference memory consumption, and training duration.
Figure 2. This diagram illustrates the overall architecture of FlashOcc and is best viewed in color with zoom capabilities. The area designated by the dashed box indicates the presence of replaceable modules. The feature shape of each replaceable module is represented by icons representing 2D image, BEV-level, and voxel-level features, respectively. The light blue area corresponds to the optional temporal fusion module, the use of which depends on the activation of the red switch.
Figure 4 shows the architectural comparison between 3D voxel-level representation processing and the plug-in replacement proposed in this article
Experimental results:
#Summary:
This article introduces a plug-and-play method called FlashOCC, designed to achieve fast and memory-efficient occupancy prediction. This method uses 2D convolutions to directly replace the 3D convolutions in voxel-based occupancy methods and combines channel-to-height transformation to reshape flattened BEV features into occupancy logits. FlashOCC has demonstrated its effectiveness and generalizability across a variety of voxel-level occupancy prediction methods. Extensive experiments demonstrate that this method outperforms previous state-of-the-art methods in terms of accuracy, time consumption, memory efficiency, and deployment-friendliness. To the best of our knowledge, FlashOCC is the first method to apply the sub-pixel paradigm (Channel-to-Height) to occupancy tasks, exclusively leveraging BEV-level features and completely avoiding the use of computational 3D (deformable) convolution or transformer modules. The visualization results convincingly demonstrate that FlashOCC successfully retains height information. In future work, this method will be integrated into the perception pipeline of autonomous driving, aiming to achieve efficient on-chip deploymentCitation:
Yu, Z., Shu, C., Deng, J., Lu, K., Liu, Z., Yu, J., Yang, D., Li, H., & Chen, Y. (2023). FlashOcc: Fast and Memory-Efficient Occupancy Prediction via Channel-to-Height Plugin. ArXiv. /abs/2311.12058The above is the detailed content of FlashOcc: New ideas for occupancy prediction, new SOTA in accuracy, efficiency and memory usage!. For more information, please follow other related articles on the PHP Chinese website!

Hot AI Tools

Undresser.AI Undress
AI-powered app for creating realistic nude photos

AI Clothes Remover
Online AI tool for removing clothes from photos.

Undress AI Tool
Undress images for free

Clothoff.io
AI clothes remover

Video Face Swap
Swap faces in any video effortlessly with our completely free AI face swap tool!

Hot Article

Hot Tools

Notepad++7.3.1
Easy-to-use and free code editor

SublimeText3 Chinese version
Chinese version, very easy to use

Zend Studio 13.0.1
Powerful PHP integrated development environment

Dreamweaver CS6
Visual web development tools

SublimeText3 Mac version
God-level code editing software (SublimeText3)

Hot Topics










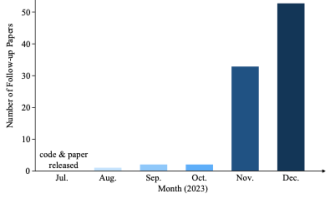
Written above & the author’s personal understanding Three-dimensional Gaussiansplatting (3DGS) is a transformative technology that has emerged in the fields of explicit radiation fields and computer graphics in recent years. This innovative method is characterized by the use of millions of 3D Gaussians, which is very different from the neural radiation field (NeRF) method, which mainly uses an implicit coordinate-based model to map spatial coordinates to pixel values. With its explicit scene representation and differentiable rendering algorithms, 3DGS not only guarantees real-time rendering capabilities, but also introduces an unprecedented level of control and scene editing. This positions 3DGS as a potential game-changer for next-generation 3D reconstruction and representation. To this end, we provide a systematic overview of the latest developments and concerns in the field of 3DGS for the first time.
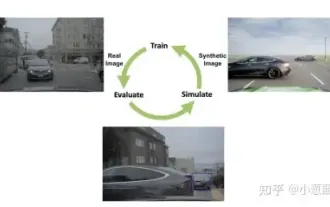
Yesterday during the interview, I was asked whether I had done any long-tail related questions, so I thought I would give a brief summary. The long-tail problem of autonomous driving refers to edge cases in autonomous vehicles, that is, possible scenarios with a low probability of occurrence. The perceived long-tail problem is one of the main reasons currently limiting the operational design domain of single-vehicle intelligent autonomous vehicles. The underlying architecture and most technical issues of autonomous driving have been solved, and the remaining 5% of long-tail problems have gradually become the key to restricting the development of autonomous driving. These problems include a variety of fragmented scenarios, extreme situations, and unpredictable human behavior. The "long tail" of edge scenarios in autonomous driving refers to edge cases in autonomous vehicles (AVs). Edge cases are possible scenarios with a low probability of occurrence. these rare events
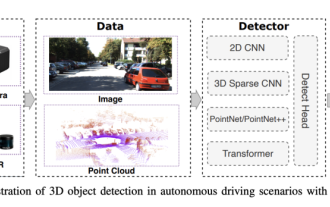
0.Written in front&& Personal understanding that autonomous driving systems rely on advanced perception, decision-making and control technologies, by using various sensors (such as cameras, lidar, radar, etc.) to perceive the surrounding environment, and using algorithms and models for real-time analysis and decision-making. This enables vehicles to recognize road signs, detect and track other vehicles, predict pedestrian behavior, etc., thereby safely operating and adapting to complex traffic environments. This technology is currently attracting widespread attention and is considered an important development area in the future of transportation. one. But what makes autonomous driving difficult is figuring out how to make the car understand what's going on around it. This requires that the three-dimensional object detection algorithm in the autonomous driving system can accurately perceive and describe objects in the surrounding environment, including their locations,

Original title: SIMPL: ASimpleandEfficientMulti-agentMotionPredictionBaselineforAutonomousDriving Paper link: https://arxiv.org/pdf/2402.02519.pdf Code link: https://github.com/HKUST-Aerial-Robotics/SIMPL Author unit: Hong Kong University of Science and Technology DJI Paper idea: This paper proposes a simple and efficient motion prediction baseline (SIMPL) for autonomous vehicles. Compared with traditional agent-cent
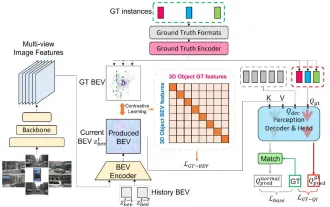
Written above & the author’s personal understanding: At present, in the entire autonomous driving system, the perception module plays a vital role. The autonomous vehicle driving on the road can only obtain accurate perception results through the perception module. The downstream regulation and control module in the autonomous driving system makes timely and correct judgments and behavioral decisions. Currently, cars with autonomous driving functions are usually equipped with a variety of data information sensors including surround-view camera sensors, lidar sensors, and millimeter-wave radar sensors to collect information in different modalities to achieve accurate perception tasks. The BEV perception algorithm based on pure vision is favored by the industry because of its low hardware cost and easy deployment, and its output results can be easily applied to various downstream tasks.
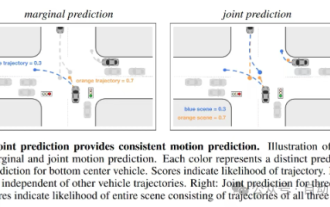
Trajectory prediction plays an important role in autonomous driving. Autonomous driving trajectory prediction refers to predicting the future driving trajectory of the vehicle by analyzing various data during the vehicle's driving process. As the core module of autonomous driving, the quality of trajectory prediction is crucial to downstream planning control. The trajectory prediction task has a rich technology stack and requires familiarity with autonomous driving dynamic/static perception, high-precision maps, lane lines, neural network architecture (CNN&GNN&Transformer) skills, etc. It is very difficult to get started! Many fans hope to get started with trajectory prediction as soon as possible and avoid pitfalls. Today I will take stock of some common problems and introductory learning methods for trajectory prediction! Introductory related knowledge 1. Are the preview papers in order? A: Look at the survey first, p
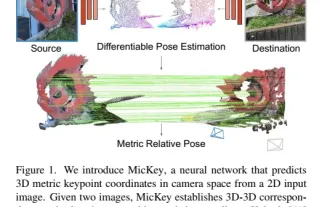
Project link written in front: https://nianticlabs.github.io/mickey/ Given two pictures, the camera pose between them can be estimated by establishing the correspondence between the pictures. Typically, these correspondences are 2D to 2D, and our estimated poses are scale-indeterminate. Some applications, such as instant augmented reality anytime, anywhere, require pose estimation of scale metrics, so they rely on external depth estimators to recover scale. This paper proposes MicKey, a keypoint matching process capable of predicting metric correspondences in 3D camera space. By learning 3D coordinate matching across images, we are able to infer metric relative
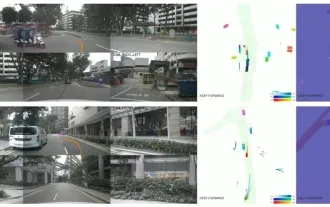
In the past month, due to some well-known reasons, I have had very intensive exchanges with various teachers and classmates in the industry. An inevitable topic in the exchange is naturally end-to-end and the popular Tesla FSDV12. I would like to take this opportunity to sort out some of my thoughts and opinions at this moment for your reference and discussion. How to define an end-to-end autonomous driving system, and what problems should be expected to be solved end-to-end? According to the most traditional definition, an end-to-end system refers to a system that inputs raw information from sensors and directly outputs variables of concern to the task. For example, in image recognition, CNN can be called end-to-end compared to the traditional feature extractor + classifier method. In autonomous driving tasks, input data from various sensors (camera/LiDAR
