Microsoft Orca-2 13B small language model beats 70B alternatives
#Microsoft recently released a new research paper on its next-generation Orca-2 AI model. Proving that the power of artificial intelligence not only applies to the largest and most complex systems, but also thrives in more compact and accessible frameworks. Microsoft has taken a bold step in this direction with the launch of Orca-2, a language model that challenges the idea that bigger always means better popular concepts. This new development is particularly interesting for those who are passionate about artificial intelligence and seek to push the boundaries of what these systems are capable of. Microsoft's research paper titled "Orca-2: Teaching small language models how to reason" provides an insight into how to train small models like Orca-2 to improve their
reasoning capabilitiesAn interesting exploration. Orca-2 has only 13 billion parameters, proving that training quality can significantly affect the model's inference capabilities. This is a crucial insight for anyone interested in the potential of small models to perform complex tasks that were once considered the exclusive domain of larger models. Microsoft explains more: "Orca 2 is our latest step in exploring the capabilities of small LMs (approximately 10 billion parameters or less). With Orca 2, we continue to demonstrate that improved training signals and methods can Enables smaller language models to achieve enhanced inference capabilities typically only found in larger language models. One of the most striking aspects of Orca-2 is its ability to outperform on inference tasks Through models with up to
70 billion parameters. This is a testament to Microsoft's innovative approach and is especially important for those working under computational constraints or looking for more efficient AI solutions. Orca-2 The benchmark results highlight the model's inference capabilities, a key element of high-level language understanding.
Orca-2 Small Language ModelOrca-2 comes in two sizes (7 billion and 13 billion parameters); both were created by fine-tuning the corresponding LLAMA 2 base model on custom high-quality synthetic data. We are making the Orca 2 weights publicly available to encourage the development, evaluation, and alignment of small LMs Research.
Microsoft Orca-2
To underscore their commitment to collaborative advancements in artificial intelligence, Microsoft has made model weights for Orca-2
available to the open source community. This enables Enthusiasts and researchers alike can take advantage of this state-of-the-art technology, integrate it into their own projects, and contribute to the collective advancement of artificial intelligence.
This research paper goes beyond traditional imitation learning, and introduces alternative training methods that give Orca-2 multiple inference strategies. These methods enable the model to adapt to different tasks, indicating a more sophisticated approach to AI training. For those who delve into the complexities of artificial intelligence Said, this represents an opportunity to explore new training paradigms that could redefine the way we teach machines to think. Orca-2 was conducted on a carefully constructed
synthetic datasettraining, achieving significant baseline performance. This means that the model has been honed through strategic data use, ensuring its effectiveness and adaptability in real-world applications. For practitioners, this means that a model not only functions Robust, yet also versatile in handling a variety of scenarios.
Orca-2’s licensing terms are tailored to emphasize its research-oriented nature. This is something to consider when planning to use the model important factor as it supports a research-focused development environment and guides the application of Orca-2 in a variety of projects.Microsoft also provides detailed instructions for setting up Orca-2 on your local computer. This enables users to tailor the model to their specific needs and gain a deeper understanding of its inner workings. Whether you are a developer, researcher or AI enthusiast, this level of customization is essential for exploring the full capabilities of Orca-2 priceless.
Microsoft’s Orca-2 represents a major advance in compact language models, providing enhanced inference capabilities and challenging the dominance of large models. Whether through open source collaboration, innovative training technology, or research initiatives, partnering with Orca-2 puts you at the forefront of a transformative period in AI development. Microsoft's Orca-2 not only broadens the horizons of what small models can do, but also invites you to take an active role in this exciting field.
The above is the detailed content of Microsoft Orca-2 13B small language model beats 70B alternatives. For more information, please follow other related articles on the PHP Chinese website!

Hot AI Tools

Undresser.AI Undress
AI-powered app for creating realistic nude photos

AI Clothes Remover
Online AI tool for removing clothes from photos.

Undress AI Tool
Undress images for free

Clothoff.io
AI clothes remover

AI Hentai Generator
Generate AI Hentai for free.

Hot Article

Hot Tools

Notepad++7.3.1
Easy-to-use and free code editor

SublimeText3 Chinese version
Chinese version, very easy to use

Zend Studio 13.0.1
Powerful PHP integrated development environment

Dreamweaver CS6
Visual web development tools

SublimeText3 Mac version
God-level code editing software (SublimeText3)

Hot Topics
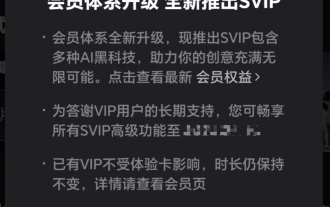
This site reported on June 27 that Jianying is a video editing software developed by FaceMeng Technology, a subsidiary of ByteDance. It relies on the Douyin platform and basically produces short video content for users of the platform. It is compatible with iOS, Android, and Windows. , MacOS and other operating systems. Jianying officially announced the upgrade of its membership system and launched a new SVIP, which includes a variety of AI black technologies, such as intelligent translation, intelligent highlighting, intelligent packaging, digital human synthesis, etc. In terms of price, the monthly fee for clipping SVIP is 79 yuan, the annual fee is 599 yuan (note on this site: equivalent to 49.9 yuan per month), the continuous monthly subscription is 59 yuan per month, and the continuous annual subscription is 499 yuan per year (equivalent to 41.6 yuan per month) . In addition, the cut official also stated that in order to improve the user experience, those who have subscribed to the original VIP
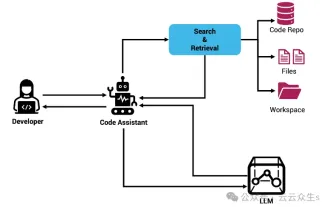
Improve developer productivity, efficiency, and accuracy by incorporating retrieval-enhanced generation and semantic memory into AI coding assistants. Translated from EnhancingAICodingAssistantswithContextUsingRAGandSEM-RAG, author JanakiramMSV. While basic AI programming assistants are naturally helpful, they often fail to provide the most relevant and correct code suggestions because they rely on a general understanding of the software language and the most common patterns of writing software. The code generated by these coding assistants is suitable for solving the problems they are responsible for solving, but often does not conform to the coding standards, conventions and styles of the individual teams. This often results in suggestions that need to be modified or refined in order for the code to be accepted into the application
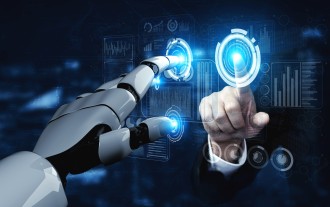
Large Language Models (LLMs) are trained on huge text databases, where they acquire large amounts of real-world knowledge. This knowledge is embedded into their parameters and can then be used when needed. The knowledge of these models is "reified" at the end of training. At the end of pre-training, the model actually stops learning. Align or fine-tune the model to learn how to leverage this knowledge and respond more naturally to user questions. But sometimes model knowledge is not enough, and although the model can access external content through RAG, it is considered beneficial to adapt the model to new domains through fine-tuning. This fine-tuning is performed using input from human annotators or other LLM creations, where the model encounters additional real-world knowledge and integrates it
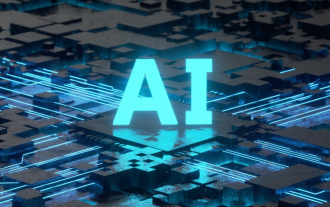
Editor |ScienceAI Question Answering (QA) data set plays a vital role in promoting natural language processing (NLP) research. High-quality QA data sets can not only be used to fine-tune models, but also effectively evaluate the capabilities of large language models (LLM), especially the ability to understand and reason about scientific knowledge. Although there are currently many scientific QA data sets covering medicine, chemistry, biology and other fields, these data sets still have some shortcomings. First, the data form is relatively simple, most of which are multiple-choice questions. They are easy to evaluate, but limit the model's answer selection range and cannot fully test the model's ability to answer scientific questions. In contrast, open-ended Q&A
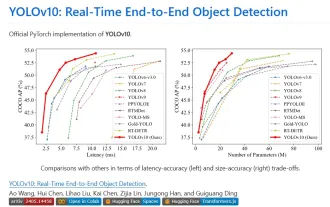
1. Introduction Over the past few years, YOLOs have become the dominant paradigm in the field of real-time object detection due to its effective balance between computational cost and detection performance. Researchers have explored YOLO's architectural design, optimization goals, data expansion strategies, etc., and have made significant progress. At the same time, relying on non-maximum suppression (NMS) for post-processing hinders end-to-end deployment of YOLO and adversely affects inference latency. In YOLOs, the design of various components lacks comprehensive and thorough inspection, resulting in significant computational redundancy and limiting the capabilities of the model. It offers suboptimal efficiency, and relatively large potential for performance improvement. In this work, the goal is to further improve the performance efficiency boundary of YOLO from both post-processing and model architecture. to this end
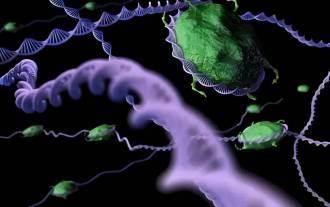
Editor | KX In the field of drug research and development, accurately and effectively predicting the binding affinity of proteins and ligands is crucial for drug screening and optimization. However, current studies do not take into account the important role of molecular surface information in protein-ligand interactions. Based on this, researchers from Xiamen University proposed a novel multi-modal feature extraction (MFE) framework, which for the first time combines information on protein surface, 3D structure and sequence, and uses a cross-attention mechanism to compare different modalities. feature alignment. Experimental results demonstrate that this method achieves state-of-the-art performance in predicting protein-ligand binding affinities. Furthermore, ablation studies demonstrate the effectiveness and necessity of protein surface information and multimodal feature alignment within this framework. Related research begins with "S
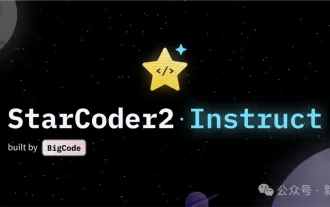
At the forefront of software technology, UIUC Zhang Lingming's group, together with researchers from the BigCode organization, recently announced the StarCoder2-15B-Instruct large code model. This innovative achievement achieved a significant breakthrough in code generation tasks, successfully surpassing CodeLlama-70B-Instruct and reaching the top of the code generation performance list. The unique feature of StarCoder2-15B-Instruct is its pure self-alignment strategy. The entire training process is open, transparent, and completely autonomous and controllable. The model generates thousands of instructions via StarCoder2-15B in response to fine-tuning the StarCoder-15B base model without relying on expensive manual annotation.
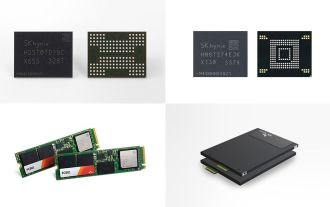
According to news from this site on August 1, SK Hynix released a blog post today (August 1), announcing that it will attend the Global Semiconductor Memory Summit FMS2024 to be held in Santa Clara, California, USA from August 6 to 8, showcasing many new technologies. generation product. Introduction to the Future Memory and Storage Summit (FutureMemoryandStorage), formerly the Flash Memory Summit (FlashMemorySummit) mainly for NAND suppliers, in the context of increasing attention to artificial intelligence technology, this year was renamed the Future Memory and Storage Summit (FutureMemoryandStorage) to invite DRAM and storage vendors and many more players. New product SK hynix launched last year