


Asynchronous coroutine development practice: optimizing the speed and efficiency of big data processing
Asynchronous Coroutine Development Practice: Optimizing the Speed and Efficiency of Big Data Processing
Introduction:
In today's digital era, big data processing has become an important issue in all walks of life. important needs of the industry. However, with the increase in data volume and complexity, traditional methods can no longer meet the speed and efficiency requirements for processing big data. In order to solve this problem, asynchronous coroutine development has gradually emerged in recent years. This article will introduce what asynchronous coroutine development is and how to use asynchronous coroutine development to optimize the speed and efficiency of big data processing, and provide specific code examples.
1. What is asynchronous coroutine development
Asynchronous coroutine development is a concurrent programming method that allows the program to release CPU resources to perform other tasks while waiting for an operation to be completed. Thereby improving the concurrency capability and response performance of the program. Compared with traditional thread or process methods, asynchronous coroutine development is more lightweight, efficient and easy to use.
2. Why use asynchronous coroutines to develop and optimize big data processing
In the process of big data processing, a large number of IO operations are often required, such as reading files, requesting the network, accessing the database, etc. In traditional programming methods, these IO operations are often blocking, which means that the program must wait for the IO operation to complete before continuing to the next step. During this waiting process, CPU resources are idle, resulting in low processing efficiency.
Asynchronous coroutine development solves this problem by converting IO operations into non-blocking methods. When the program encounters an IO operation, it will initiate an asynchronous request and continue to perform subsequent operations instead of waiting for the IO operation to complete. When the IO operation is completed, the program will process the results according to the pre-defined callback function. This method greatly improves the concurrency and response speed of the program.
3. Asynchronous Coroutine Development Practice: Optimizing the Speed and Efficiency of Big Data Processing
The following is a sample code that uses asynchronous coroutine development to process big data:
import asyncio async def process_data(data): # 模拟耗时的数据处理操作 await asyncio.sleep(1) # 处理数据 processed_data = data.upper() return processed_data async def process_big_data(big_data): processed_data_list = [] tasks = [] for data in big_data: # 创建协程任务 task = asyncio.create_task(process_data(data)) tasks.append(task) # 并发执行协程任务 processed_data_list = await asyncio.gather(*tasks) return processed_data_list async def main(): # 构造大数据 big_data = ['data1', 'data2', 'data3', ...] # 处理大数据 processed_data_list = await process_big_data(big_data) # 输出处理结果 print(processed_data_list) if __name__ == '__main__': asyncio.run(main())
Above In the code, the process_data
function simulates a time-consuming data processing operation and returns the processing result using the await
keyword. The process_big_data
function creates multiple coroutine tasks and uses the asyncio.gather
function to execute these tasks concurrently. Finally, the main
function is responsible for constructing big data, calling the process_big_data
function to process the data, and output the processing results.
By using asynchronous coroutine development, the above code can execute the processing of big data concurrently, making full use of CPU resources and improving the speed and efficiency of data processing. Moreover, because asynchronous coroutine development is based on event loops, it is more lightweight than multi-threading or multi-process, avoiding the overhead of thread switching and context switching.
Conclusion:
Asynchronous coroutine development is an important means to optimize big data processing. By using asynchronous coroutine development, big data processing tasks can be executed concurrently, making full use of CPU resources and improving the speed and efficiency of data processing. This article introduces the concepts and principles of asynchronous coroutine development and provides a specific code example, hoping to help readers better understand asynchronous coroutine development and apply it to actual big data processing.
The above is the detailed content of Asynchronous coroutine development practice: optimizing the speed and efficiency of big data processing. For more information, please follow other related articles on the PHP Chinese website!

Hot AI Tools

Undresser.AI Undress
AI-powered app for creating realistic nude photos

AI Clothes Remover
Online AI tool for removing clothes from photos.

Undress AI Tool
Undress images for free

Clothoff.io
AI clothes remover

Video Face Swap
Swap faces in any video effortlessly with our completely free AI face swap tool!

Hot Article

Hot Tools

Notepad++7.3.1
Easy-to-use and free code editor

SublimeText3 Chinese version
Chinese version, very easy to use

Zend Studio 13.0.1
Powerful PHP integrated development environment

Dreamweaver CS6
Visual web development tools

SublimeText3 Mac version
God-level code editing software (SublimeText3)

Hot Topics


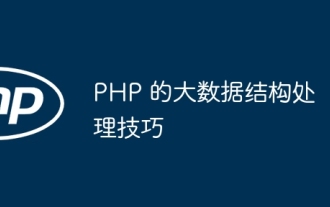
Big data structure processing skills: Chunking: Break down the data set and process it in chunks to reduce memory consumption. Generator: Generate data items one by one without loading the entire data set, suitable for unlimited data sets. Streaming: Read files or query results line by line, suitable for large files or remote data. External storage: For very large data sets, store the data in a database or NoSQL.
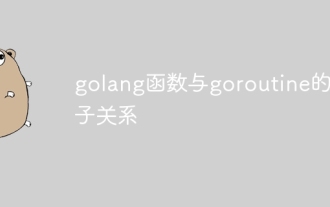
There is a parent-child relationship between functions and goroutines in Go. The parent goroutine creates the child goroutine, and the child goroutine can access the variables of the parent goroutine but not vice versa. Create a child goroutine using the go keyword, and the child goroutine is executed through an anonymous function or a named function. A parent goroutine can wait for child goroutines to complete via sync.WaitGroup to ensure that the program does not exit before all child goroutines have completed.
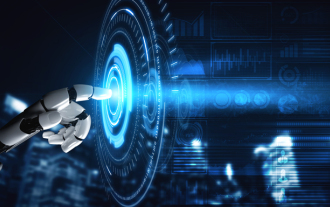
AEC/O (Architecture, Engineering & Construction/Operation) refers to the comprehensive services that provide architectural design, engineering design, construction and operation in the construction industry. In 2024, the AEC/O industry faces changing challenges amid technological advancements. This year is expected to see the integration of advanced technologies, heralding a paradigm shift in design, construction and operations. In response to these changes, industries are redefining work processes, adjusting priorities, and enhancing collaboration to adapt to the needs of a rapidly changing world. The following five major trends in the AEC/O industry will become key themes in 2024, recommending it move towards a more integrated, responsive and sustainable future: integrated supply chain, smart manufacturing
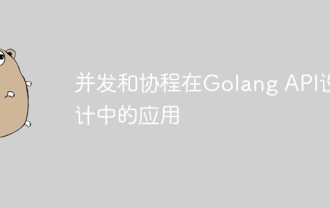
Concurrency and coroutines are used in GoAPI design for: High-performance processing: Processing multiple requests simultaneously to improve performance. Asynchronous processing: Use coroutines to process tasks (such as sending emails) asynchronously, releasing the main thread. Stream processing: Use coroutines to efficiently process data streams (such as database reads).
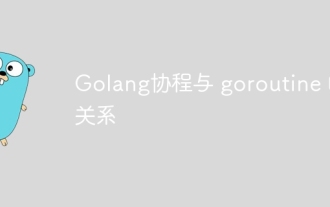
Coroutine is an abstract concept for executing tasks concurrently, and goroutine is a lightweight thread function in the Go language that implements the concept of coroutine. The two are closely related, but goroutine resource consumption is lower and managed by the Go scheduler. Goroutine is widely used in actual combat, such as concurrently processing web requests and improving program performance.
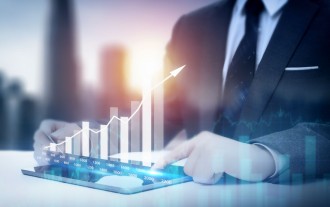
1. Background of the Construction of 58 Portraits Platform First of all, I would like to share with you the background of the construction of the 58 Portrait Platform. 1. The traditional thinking of the traditional profiling platform is no longer enough. Building a user profiling platform relies on data warehouse modeling capabilities to integrate data from multiple business lines to build accurate user portraits; it also requires data mining to understand user behavior, interests and needs, and provide algorithms. side capabilities; finally, it also needs to have data platform capabilities to efficiently store, query and share user profile data and provide profile services. The main difference between a self-built business profiling platform and a middle-office profiling platform is that the self-built profiling platform serves a single business line and can be customized on demand; the mid-office platform serves multiple business lines, has complex modeling, and provides more general capabilities. 2.58 User portraits of the background of Zhongtai portrait construction
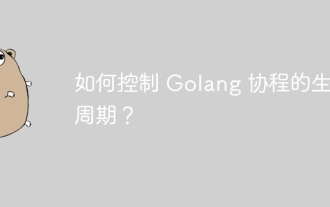
Controlling the life cycle of a Go coroutine can be done in the following ways: Create a coroutine: Use the go keyword to start a new task. Terminate coroutines: wait for all coroutines to complete, use sync.WaitGroup. Use channel closing signals. Use context context.Context.
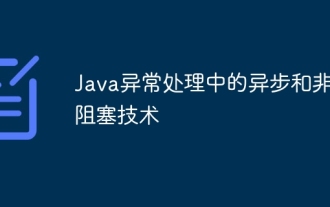
Asynchronous and non-blocking techniques can be used to complement traditional exception handling, allowing the creation of more responsive and efficient Java applications: Asynchronous exception handling: Handling exceptions in another thread or process, allowing the main thread to continue executing, avoiding blocking. Non-blocking exception handling: involves event-driven exception handling when an I/O operation goes wrong, avoiding blocking threads and allowing the event loop to handle exceptions.
