


Launched a free personalized academic paper recommendation system - the 'arXiv customized platform' of the top visual teams of German universities
Generate one image in 10 milliseconds and 6,000 images in 1 minute. What is the concept?
In the picture below, you can deeply feel the super power of AI.
Picture
Even, when you continue to add new elements to the prompts generated by the two-dimensional lady pictures, each The change of pictures in this style also flashes in an instant.
Pictures
Such an amazing real-time picture generation speed is the result of StreamDiffusion proposed by researchers from UC Berkeley, University of Tsukuba, Japan, etc. bring results.
This new solution is a diffusion model process that enables real-time interactive image generation at over 100fps.
Picture
Paper address: https://arxiv.org/abs/2312.12491
After being open sourced, StreamDiffusion directly dominated the GitHub rankings, garnering 3.7k stars.
Picture
StreamDiffusion innovatively uses a batch processing strategy instead of sequence denoising, which is about 1.5 times faster than traditional methods . Moreover, the new residual classifier-free guidance (RCFG) algorithm proposed by the author can be 2.05 times faster than the traditional classifier-free guidance.
The most noteworthy thing is that the new method can achieve an image-to-image generation speed of 91.07fps on the RTX 4090.
Picture
#In the future, StreamDiffusion will quickly generate in different scenarios such as the metaverse, video game graphics rendering, and live video streaming. Able to meet the high throughput requirements of these applications.
In particular, real-time image generation can provide powerful editing and creative capabilities for those who work in game development and video rendering.
Picture
Designed specifically for real-time image generation
Currently, in various fields, diffusion models The application needs a diffusion pipeline with high throughput and low latency to ensure the efficiency of human-computer interaction
A typical example is to use the diffusion model to create the virtual character VTuber - able to Respond fluidly to user input.
Picture
In order to improve high throughput and real-time interaction capabilities, the current research direction is mainly focused on reducing denoising iterations The number of iterations, for example, is reduced from 50 iterations to a few, or even once.
A common strategy is to refine the multi-step diffusion model into several steps and reconstruct the diffusion process using ODEs. To improve efficiency, diffusion models have also been quantified.
In the latest paper, researchers started from the orthogonal direction and introduced StreamDiffusion, a real-time diffusion pipeline designed for high throughput of interactive image generation. design.
Existing model design work can be integrated with StreamDiffusion while also using N-step denoising diffusion models to maintain high throughput and provide users with more flexible options
Picture
Real-time image generation|First and second columns: examples of AI-assisted real-time drawing, third column: real-time rendering from 3D avatars 2D illustration. Columns 4 and 5: Live camera filters. Real-time image generation | The first and second columns show examples of AI-assisted real-time drawing, and the third column shows the process of generating 2D illustrations by rendering 3D avatars in real time. The fourth and fifth columns show the effect of real-time camera filters
How is it implemented?
StreamDiffusion Architecture
StreamDiffusion is a new diffusion pipeline designed to increase throughput.
It consists of several key parts:
Streaming batch processing strategy, residual classifier-free guidance (RCFG), input and output queue, random Model acceleration tools for Stochastic Similarity Filter, precomputation programs, and micro-autoencoders.
Batch denoising
In the diffusion model, the denoising steps are performed in sequence, which leads to the U-Net Processing time,increases proportionally to the number of steps.
However, in order to generate high-fidelity images, the number of steps has to be increased.
In order to solve the problem of high-latency generation in interactive diffusion, researchers proposed a method called Stream Batch.
As shown in the figure below, in the latest methods, instead of waiting for a single image to be completely denoised before processing the next input image, it accepts after each denoising step Next input image.
This forms a denoising batch, and the denoising steps for each image are staggered.
By concatenating these interleaved denoising steps into a batch, researchers can use U-Net to efficiently process batches of consecutive inputs.
The input image encoded at time step t is generated and decoded at time step t n, where n is the number of denoising steps.
Picture
Residual Classifier Free Guided (RCFG)
Common Classifier-free guidance (CFG) is a method that performs vector calculations between the unconditional or negative conditional term and the original conditional term. An algorithm to enhance the effect of the original condition.
Picture
This can bring benefits such as enhancing the effect of the prompt.
However, in order to compute negative conditional residual noise, each input latent variable needs to be paired with a negative conditional embedding and passed to U-Net at each inference time.
To solve this problem, the author introduces an innovative residual classifier-free bootstrapping (RCFG)
This method utilizes virtual residual Noise is used to approximate the negative condition, so that we only need to calculate the negative condition noise in the initial stage of the process, thereby significantly reducing the additional U-Net inference calculation cost when embedding negative conditions
Input and output queue
#Convert the input image into a pipeline-manageable tensor data format, and in turn, convert the decoded tensor back to the output image, both Requires non-negligible additional processing time.
To avoid adding these image processing times to the neural network inference process, we separate image pre- and post-processing into different threads, thereby enabling parallel processing.
In addition, by using input tensor queues, it is also possible to cope with temporary interruptions in input images due to device failures or communication errors, allowing for smooth streaming.
picture
Stochastic Similarity Filter
The following figure shows the core diffusion inference pipeline, including VAE and U-Net.
Improves the speed of the inference pipeline and enables real-time image generation by introducing denoising batching and pre-computed hint embedding cache, sampled noise cache and scheduler value cache.
Stochastic Similarity Filtering (SSF) is designed to save GPU power consumption and can dynamically close the diffusion model pipeline, thereby achieving fast and efficient real-time inference.
Picture
Precomputation
The U-Net architecture requires both input potential Variables also require conditional embedding.
Normally, conditional embedding is derived from "hint embedding" and remains unchanged between different frames.
To optimize this, the researchers pre-compute hint embeddings and store them in cache. In interactive or streaming mode, this precomputed hint embedding cache is recalled.
In U-Net, the calculation of keys and values for each frame is implemented based on pre-computed hint embeddings
Therefore, The researchers modified U-Net to store these key and value pairs so that they can be reused. Whenever the input prompt is updated, the researchers recompute and update these key and value pairs within U-Net.
Model Acceleration and Tiny Autoencoders
To optimize speed, we configured the system to use a static batch size and a fixed input size (height and width).
This approach ensures that the computation graph and memory allocation are optimized for the specific input size, resulting in faster processing.
However, this means that if you need to process images of different shapes (i.e. different heights and widths), use different batch sizes (including the batch size for the denoising step).
Experimental evaluation
Quantitative evaluation of denoising batches
## Figure 8 shows batch denoising and original sequential U- Efficiency comparison of Net loop
When implementing the batch denoising strategy, the researchers found significant improvements in processing time. This reduces the time in half compared to traditional U-Net loops with sequential denoising steps.
Even with the neural module acceleration tool TensorRT applied, the streaming batch processing proposed by the researchers can still significantly improve the efficiency of the original sequential diffusion pipeline in different denoising steps.
Picture
Additionally, the researchers compared the latest method with the AutoPipeline-ForImage2Image pipeline developed by Huggingface Diffusers.
The average inference time comparison is shown in Table 1. The latest pipeline shows that the speed has been greatly improved.
When using TensorRT, StreamDiffusion is able to achieve a 13x speedup when running 10 denoising steps. When only a single denoising step is involved, the speed increase can reach 59.6 times
Even without TensorRT, StreamDiffusion is 29.7 times faster than AutoPipeline when using single-step denoising. An 8.3x improvement when using 10-step denoising.
Picture
Table 2 compares the inference time of the flow diffusion pipeline using RCFG and conventional CFG.
In the case of single-step denoising, the inference time of Onetime-Negative RCFG and traditional CFG is almost the same.
So the inference time of One-time RCFG and traditional CFG during single-step denoising is almost the same. However, as the number of denoising steps increases, the inference speed improvement from traditional CFG to RCFG becomes more obvious.
In the fifth step of denoising, Self-Negative RCFG is 2.05 times faster than traditional CFG, and Onetime-Negative RCFG is 1.79 times faster than traditional CFG.
Picture
Picture
After this, the researchers carried out the Energy consumption was comprehensively assessed. The results of this process can be seen in Figures 6 and 7
These figures demonstrate the application of SSF (setting the threshold eta to 0.98) to the input video to contain periodic static Comparative analysis of GPU usage patterns in characteristic scenes shows that when the input images are mainly static images and have a high degree of similarity, using SSF can significantly reduce GPU usage.
Picture
Ablation study
Different modules perform different denoising steps The impact on average inference time is shown in Table 3. As can be seen, the reduction of different modules is verified in the image-to-image generation process.
Pictures
Qualitative results
are demonstrated in Figure 10 using the remaining Alignment process for fast conditional adjustment of generated images without classifier guidance (RCFG)
The generated images, without using any form of CFG, show weak alignment hints, especially in Aspects such as color changes or adding non-existent elements were not implemented efficiently.
In contrast, the use of CFG or RCFG enhances the ability to modify the original image, such as changing hair color, adding body patterns, and even including objects like glasses. Notably, the use of RCFG can enhance the impact of cues compared with standard CFG.
Picture
Finally, the quality of the standard text-to-image generation results is shown in Figure 11.
Using the sd-turbo model, you can generate high-quality images like the one shown in Figure 11 in just one step.
When using the flow diffusion pipeline and sd-turbo model proposed by the researcher in the environment of GPU: RTX 4090, CPU: Core i9-13900K, OS: Ubuntu 22.04.3 LTS When generating images, it is feasible to produce such high quality images at over 100fps.
Picture
Netizens got started, and a large wave of two-dimensional ladies came
Picture
Project address: https://github.com/cumulo-autumn/StreamDiffusion
Many netizens have begun to generate their own two-dimensional wives.
Pictures
There are also real-time animations.
Pictures
10x speed hand-drawn generation.
Picture
Picture
##Picture
Those who are interested in children's shoes, why not do it yourself.
Reference:
https://www.php.cn/link/f9d8bf6b7414e900118caa579ea1b7be
https://www.php.cn/link/75a6e5993aefba4f6cb07254637a6133
The above is the detailed content of Launched a free personalized academic paper recommendation system - the 'arXiv customized platform' of the top visual teams of German universities. For more information, please follow other related articles on the PHP Chinese website!

Hot AI Tools

Undresser.AI Undress
AI-powered app for creating realistic nude photos

AI Clothes Remover
Online AI tool for removing clothes from photos.

Undress AI Tool
Undress images for free

Clothoff.io
AI clothes remover

AI Hentai Generator
Generate AI Hentai for free.

Hot Article

Hot Tools

Notepad++7.3.1
Easy-to-use and free code editor

SublimeText3 Chinese version
Chinese version, very easy to use

Zend Studio 13.0.1
Powerful PHP integrated development environment

Dreamweaver CS6
Visual web development tools

SublimeText3 Mac version
God-level code editing software (SublimeText3)

Hot Topics


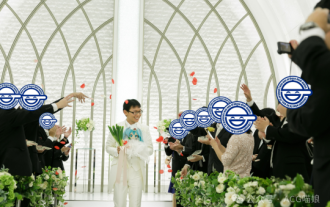
On March 31, 2020, Hatsune Miku officially "divorced" the Japanese otaku who once spent millions to marry her. It has been almost 4 years since then. In fact, when the two got married, many people were not optimistic about the couple. After all, it was very outrageous for a person living in the third dimension to marry a paper person from the second dimension. However, in the face of the criticism from netizens, the Japanese otaku Kondo Akihiko did not back down. In the end, he held a wedding with Hatsune Miku. Judging from the photos Kondo Akihiko posted from time to time after his marriage, his life with Hatsune Miku It was quite good, but unfortunately their marriage did not last too long. As the Gatebox copyright of the first-generation Hatsune model expired, Kondo Akihiko's wife Hatsune Miku also
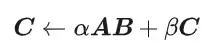
General Matrix Multiplication (GEMM) is a vital part of many applications and algorithms, and is also one of the important indicators for evaluating computer hardware performance. In-depth research and optimization of the implementation of GEMM can help us better understand high-performance computing and the relationship between software and hardware systems. In computer science, effective optimization of GEMM can increase computing speed and save resources, which is crucial to improving the overall performance of a computer system. An in-depth understanding of the working principle and optimization method of GEMM will help us better utilize the potential of modern computing hardware and provide more efficient solutions for various complex computing tasks. By optimizing the performance of GEMM
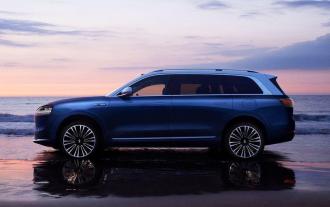
On July 29, at the roll-off ceremony of AITO Wenjie's 400,000th new car, Yu Chengdong, Huawei's Managing Director, Chairman of Terminal BG, and Chairman of Smart Car Solutions BU, attended and delivered a speech and announced that Wenjie series models will be launched this year In August, Huawei Qiankun ADS 3.0 version was launched, and it is planned to successively push upgrades from August to September. The Xiangjie S9, which will be released on August 6, will debut Huawei’s ADS3.0 intelligent driving system. With the assistance of lidar, Huawei Qiankun ADS3.0 version will greatly improve its intelligent driving capabilities, have end-to-end integrated capabilities, and adopt a new end-to-end architecture of GOD (general obstacle identification)/PDP (predictive decision-making and control) , providing the NCA function of smart driving from parking space to parking space, and upgrading CAS3.0
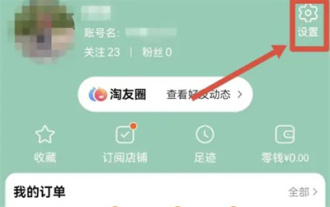
In the process of using Taobao, we will often be recommended by some friends we may know. Here is an introduction to how to turn off this function. Friends who are interested should take a look. After opening the "Taobao" APP on your mobile phone, click "My Taobao" in the lower right corner of the page to enter the personal center page, and then click the "Settings" function in the upper right corner to enter the settings page. 2. After coming to the settings page, find "Privacy" and click on this item to enter. 3. There is a "Recommend friends to me" on the privacy page. When it shows that the current status is "on", click on it to close it. 4. Finally, in the pop-up window, there will be a switch button behind "Recommend friends to me". Click on it to set the button to gray.
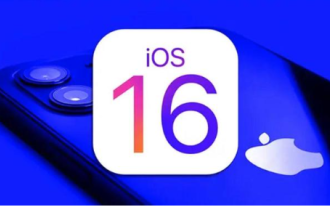
The best version of the Apple 16 system is iOS16.1.4. The best version of the iOS16 system may vary from person to person. The additions and improvements in daily use experience have also been praised by many users. Which version of the Apple 16 system is the best? Answer: iOS16.1.4 The best version of the iOS 16 system may vary from person to person. According to public information, iOS16, launched in 2022, is considered a very stable and performant version, and users are quite satisfied with its overall experience. In addition, the addition of new features and improvements in daily use experience in iOS16 have also been well received by many users. Especially in terms of updated battery life, signal performance and heating control, user feedback has been relatively positive. However, considering iPhone14
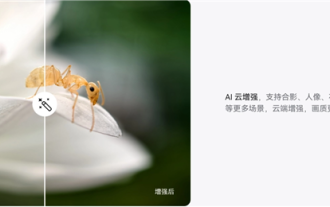
On April 11, Huawei officially announced the HarmonyOS 4.2 100-machine upgrade plan for the first time. This time, more than 180 devices will participate in the upgrade, covering mobile phones, tablets, watches, headphones, smart screens and other devices. In the past month, with the steady progress of the HarmonyOS4.2 100-machine upgrade plan, many popular models including Huawei Pocket2, Huawei MateX5 series, nova12 series, Huawei Pura series, etc. have also started to upgrade and adapt, which means that there will be More Huawei model users can enjoy the common and often new experience brought by HarmonyOS. Judging from user feedback, the experience of Huawei Mate60 series models has improved in all aspects after upgrading HarmonyOS4.2. Especially Huawei M
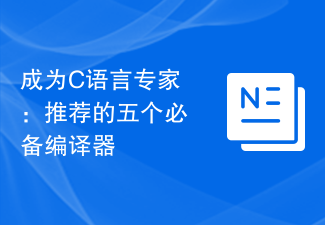
From Beginner to Expert: Five Essential C Compiler Recommendations With the development of computer science, more and more people are interested in programming languages. As a high-level language widely used in system-level programming, C language has always been loved by programmers. In order to write efficient and stable code, it is important to choose a C language compiler that suits you. This article will introduce five essential C language compilers for beginners and experts to choose from. GCCGCC, the GNU compiler collection, is one of the most commonly used C language compilers
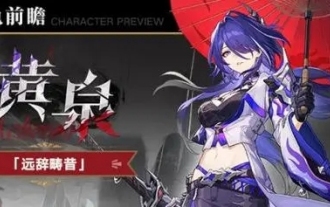
Huang Quan's light cone can effectively increase the character's critical hit damage and attack power in battle. The light cones recommended by Huang Quan are: Walking on the Passing Shore, Good Night and Sleeping Face, Rain Keeps Falling, Just Wait, and Determination Like Beads of Sweat. Shine, below the editor will bring you recommendations for the Underworld Light Cone of the Collapsed Star Dome Railway. Huangquan Light Cone Recommendation 1. Walking on the Passing Bank 1. Huangquan's special weapon can increase the explosive damage. Attacking the enemy can put the enemy into a bubble negative state, which increases the damage caused. The damage of the finishing move is additionally increased. There are both negative states and The damage is increased, it has to be said that it is a special weapon. 2. The exclusive light cone is very unique among many ethereal light cones. It directly increases direct damage, has high damage and improves the critical damage attribute. 3. Not only that, the light cone also provides a negative status effect, which can cause Huangquan itself to react.
