The purpose of Test-Time Adaptation is to adapt the source domain model to the test data in the inference phase, and has achieved excellent results in adapting to unknown image damage fields. However, many current methods lack consideration of the test data flow in real-world scenarios, for example:
- The test data flow should be time-varying distribution ( Rather than a fixed distribution in traditional domain adaptation)
- The test data stream may have local class correlations (rather than completely independent and identically distributed sampling)
- The test data stream still shows global category imbalance for a long time
Recently, South China University of Technology, A* The STAR and CUHK-Shenzhen teams have proven through a large number of experiments that test data flows in these real scenarios will bring huge challenges to existing methods. The team believes that the failure of state-of-the-art methods is first caused by indiscriminately adjusting the normalization layer based on imbalanced test data. To this end, the research teamproposed an innovative Balanced BatchNorm Layer(Balanced BatchNorm Layer) to replace the conventional inference phase Batch normalization layer. At the same time, they found that relying solely on self-training (ST) to learn in unknown test data streams can easily lead to over-adaptation (pseudo-label category imbalance, target domain is not a fixed domain), resulting in poor performance in a changing domain. . Therefore, the team recommends regularizing model updates through anchored loss (Anchored Loss), thereby improving self-reliance under continuous domain transfer Training helps to significantly improve the robustness of the model. In the end, the model TRIBE stably achieved state-of-the-art performance under four data sets and multiple real-world test data stream settings, and significantly surpassed existing advanced methods. Research paper has been accepted by AAAI 2024. 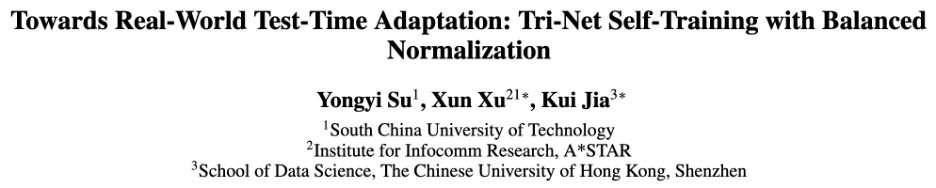
Paper link: https://arxiv.org/abs/2309.14949Code link: https://github.com/Gorilla-Lab- SCUT/TRIBEThe success of deep neural networks relies on generalizing the trained model to i.i.d. assumptions in the test domain . However, in practical applications, the robustness of out-of-distribution test data, such as visual damage caused by different lighting conditions or severe weather, is a concern. Recent research shows that this data loss can seriously affect the performance of pre-trained models. Importantly, the corruption (distribution) of test data is often unknown and sometimes unpredictable before deployment.
Therefore, adjusting the pre-trained model to adapt to the test data distribution in the inference phase is a worthy new topic, namely test-time domain adaptation (TTA). Previously, TTA was mainly implemented through distribution alignment (TTAC, TTT), self-supervised training (AdaContrast) and self-training (Conjugate PL), which have brought significant and robust improvements in a variety of visual damage test data.
Existing test-time domain adaptation (TTA) methods are usually based on some strict test data assumptions, such as stable class distribution, samples obey independent and identically distributed sampling, and fixed domain offset. These assumptions have inspired many researchers to explore real-world test data flows, such as CoTTA, NOTE, SAR, and RoTTA.
Recently, research on real-world TTA, such as SAR (ICLR 2023) and RoTTA (CVPR 2023), has mainly focused on the challenges posed by local class imbalance and continuous domain shift to TTA. Local class imbalance usually results from the fact that the test data is not sampled independently and identically distributedly. Direct indiscriminate domain adaptation will lead to biased distribution estimates.
Recent research has proposed exponentially updated batch normalized statistics (RoTTA) or instance-level discriminative updated batch normalized statistics (NOTE) to solve this challenge. The research goal is to transcend the challenge of local class imbalance, considering that the overall distribution of test data may be severely imbalanced and the distribution of classes may also change over time. A diagram of a more challenging scenario can be seen in Figure 1 below.
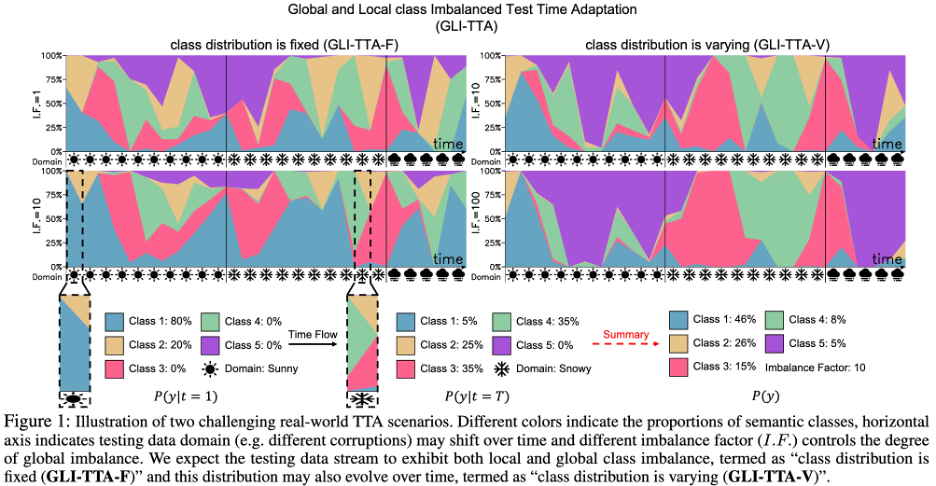
Since the class prevalence in the test data is unknown before the inference stage, and the model may be biased towards the majority class through blind test time adjustments, this renders existing TTA methods ineffective. Based on empirical observations, this problem becomes particularly prominent for methods that rely on the current batch of data to estimate global statistics for updating the normalization layer (BN, PL, TENT, CoTTA, etc.). 1. The current batch of data will be affected by local category imbalance, resulting in a biased overall distribution estimate;2. Estimate a single global distribution from the entire test data with global class imbalance. The global distribution can easily be biased towards the majority class, causing internal covariate shifts. In order to avoid biased batch normalization (BN), the team proposed a balanced batch normalization layer (Balanced Batch Normalization Layer), which is The distribution of each individual class is modeled and the global distribution is extracted from the class distribution. The balanced batch normalization layer allows obtaining class-balanced estimates of distributions under locally and globally class-imbalanced test data streams. Domain shifts occur frequently in real-world test data over time, such as gradual changes in lighting/weather conditions. This brings another challenge to existing TTA methods, the TTA model may become inconsistent when switching from domain A to domain B due to over-adaptation to domain A.
In order to alleviate over-adaptation to a certain short-term domain, CoTTA randomly restores parameters, and EATA uses fisher information to regularize the parameters. Nonetheless, these methods still do not explicitly address the emerging challenges in the field of test data.
This article introduces an anchor network (Anchor Network) to form a three-network self-training model (Tri-Net Self-Training) based on the two-branch self-training architecture. The anchor network is a frozen source model but allows tuning statistics rather than parameters in the batch normalization layer via test samples. And an anchoring loss is proposed to use the output of the anchor network to regularize the output of the teacher model to avoid the network from over-adapting to the local distribution.
The final model combines a three-net self-training model and a balanced batch normalization layer (TRI-net self-training with BalancEd normalization, TRIBE) to perform well in a wider range of adjustable learning rates. Consistently superior performance. It shows substantial performance improvements under four data sets and multiple real-world data streams, demonstrating the unique stability and robustness.
##The paper method is divided into three parts:
- Introducing the TTA protocol in the real world;
- Balanced batch normalization;
- Three-network self-training model.
TTA protocol in the real worldThe author uses a mathematical probability model to model the real-world test data flow with local class imbalance and global class imbalance, as well as the domain distribution that changes over time. As shown in Figure 2 below.
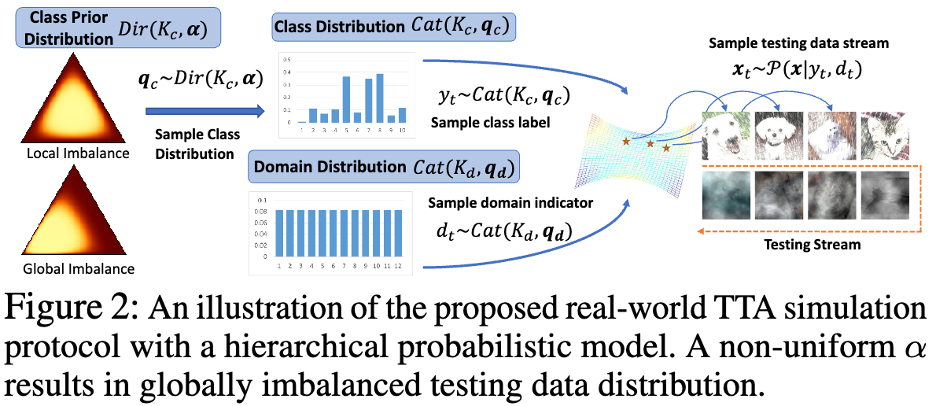
Balanced batch normalizationIn order to correct the unbalanced test data for BN To estimate the bias generated by statistics, the author proposes a balanced batch normalization layer, which maintains a pair of statistics for each semantic class, expressed as:
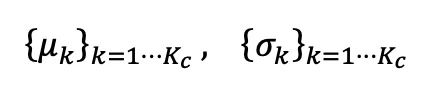
To update category statistics, the author applies an efficient iterative update method with the help of pseudo-label prediction, as shown below:
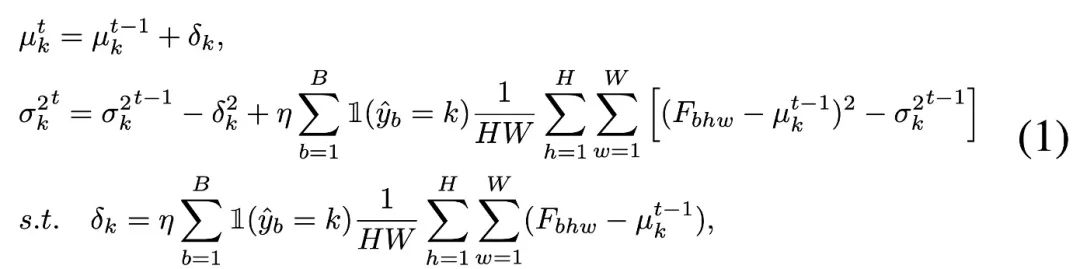
Use pseudo-labels to separately count the sampling points of each category of data, and re-obtain the overall distribution statistics under category balance through the following formula, so as to align the source with category balance A good feature space for data learning.
In some special cases, the author found that when the number of categories is large
or the pseudo-label accuracy is low (accuracy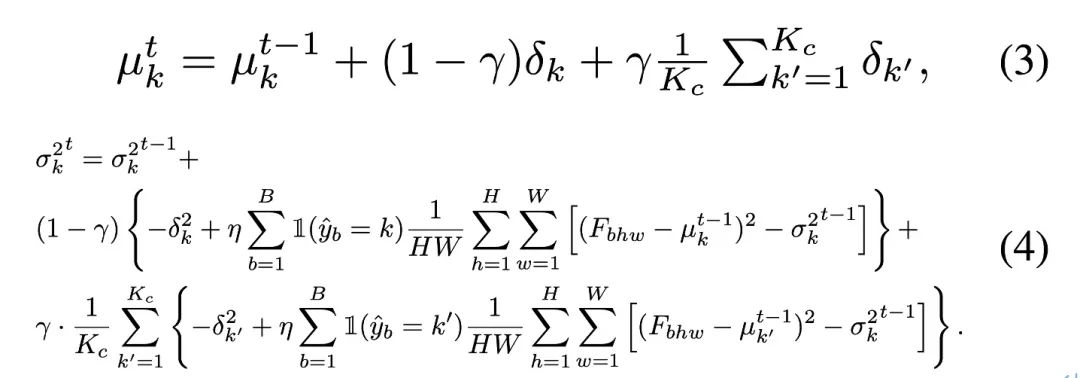
Through further analysis and observation, the author found that when γ=1 When γ = 0, the entire update strategy degenerates into the RobustBN update strategy in RoTTA. When γ = 0, it is a purely category-independent update strategy. Therefore, when γ takes a value of 0 to 1, it can be adapted to various situations. Three network self-training modelThe author is now Based on some student-teacher models, an anchoring network branch is added, and anchoring loss is introduced to constrain the prediction distribution of the teacher network. This design was inspired by TTAC. TTAC points out that relying solely on self-training on the test data stream will easily lead to the accumulation of confirmation bias. This problem is more serious on the real-world test data stream in this article. TTAC uses statistical information collected from the source domain to implement domain alignment regularization, but for the Fully TTA setting, this source domain information is not collectible. At the same time, the author also gained another revelation. The success of unsupervised domain alignment is based on the assumption that the two domain distributions have a relatively high overlap rate. Therefore, the author only adjusted the frozen source domain model of the BN statistic to regularize the teacher model to prevent the teacher model's prediction distribution from deviating too far from the source model's prediction distribution (this destroyed the previous experience of high coincidence rate between the two distributions) observation). A large number of experiments prove that the discoveries and innovations in this article are correct and robust. The following is the expression of anchoring loss: 
The following figure shows the frame diagram of the TRIBE network:
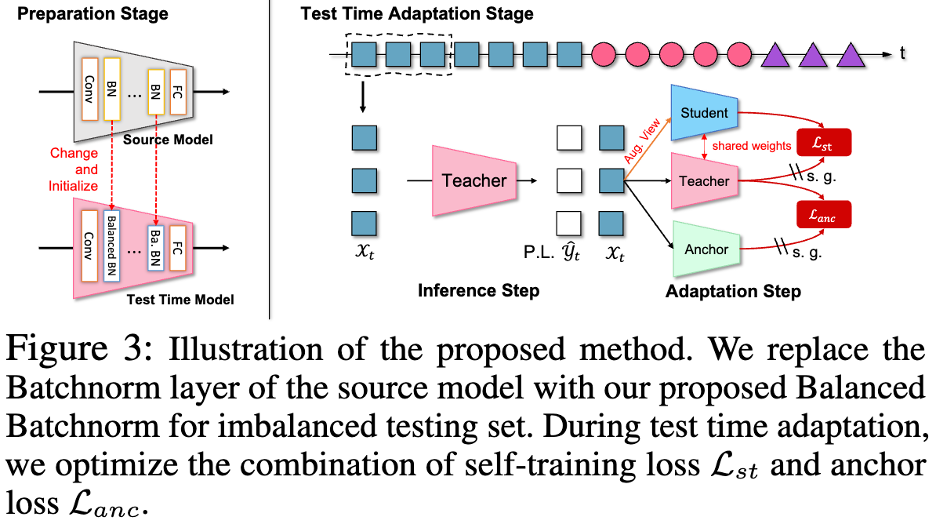
The author of the paper conducted TRIBE on 4 data sets based on two real-world TTA protocols. verified. Two real-world TTA protocols are GLI-TTA-F where the global class distribution is fixed and GLI-TTA-V where the global class distribution is not fixed. 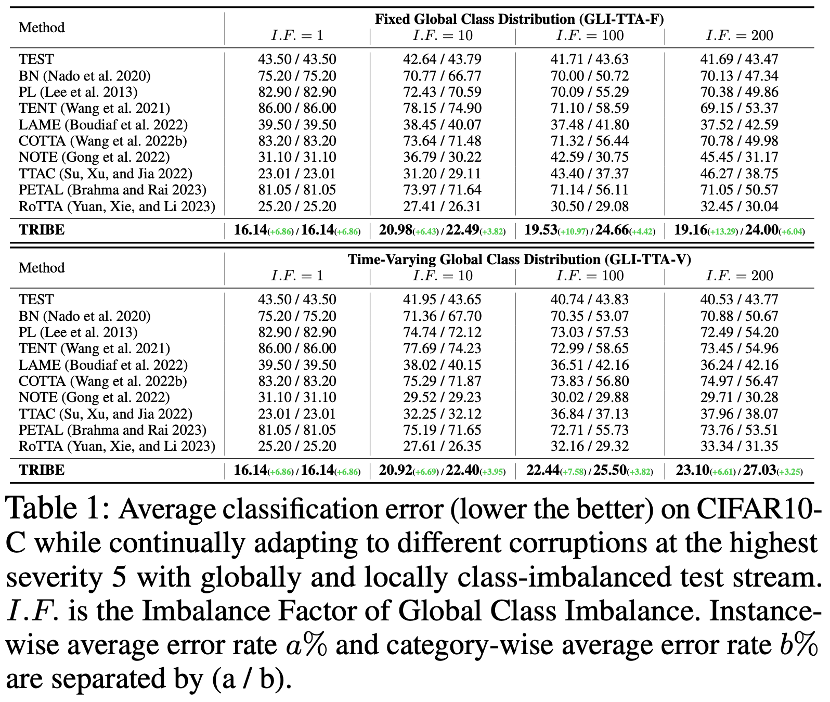
The above table shows the performance of the two protocols in the CIFAR10-C data set under different imbalance coefficients. The following conclusions can be drawn: 1. Only LAME, TTAC, NOTE, RoTTA and the TRIBE proposed in the paper exceed the TEST baseline, indicating the necessity of a more robust TTA method under real test flows. 2. Global class imbalance has brought great challenges to existing TTA methods. For example, the previous SOTA method RoTTA showed an error rate of 25.20% when I.F.=1 But when I.F.=200, the error rate rises to 32.45%. In comparison, TRIBE can stably demonstrate relatively good performance. 3. The consistency of TRIBE has an absolute advantage, surpassing all previous methods, and surpassing the previous SOTA (under the setting of global class balance (I.F.=1) TTAC) about 7%, and achieved a performance improvement of about 13% under the more difficult global class imbalance (I.F.=200) setting. 4. From I.F.=10 to I.F.=200, other TTA methods show a trend of performance decline as the imbalance increases. TRIBE can maintain relatively stable performance. This is attributed to the introduction of a balanced batch normalization layer that better accounts for severe class imbalance and anchoring loss, which avoids over-adaptation across different domains. For more data set results, please refer to the original paper. In addition, Table 4 shows the detailed modular ablation, with the following observational conclusions: 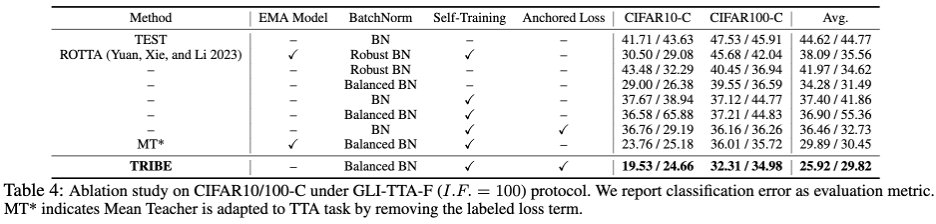
1. Only replacing BN with the balanced batch normalization layer (Balanced BN), without updating any model parameters, and only updating the BN statistics through forward can bring about a performance improvement of 10.24% (44.62 -> 34.28), and It surpasses Robust BN's error rate of 41.97%. 2. Anchored Loss combined with Self-Training, whether under the previous BN structure or the latest Balanced BN structure, has improved performance and surpassed EMA Regularization effect of Model. The rest of this article and the 9-page appendix finally present 17 detailed tabular results, demonstrating the stability, robustness and superiority of TRIBE from multiple dimensions. The appendix also contains a more detailed theoretical derivation and explanation of the balanced batch normalization layer. ##In order to deal with the real world Facing many challenges such as non-i.i.d. test data flow, global class imbalance and continuous domain transfer, the research team deeply explored how to improve the robustness of domain adaptation algorithms at test time. In order to adapt to the unbalanced test data, the author proposed a Balanced Batchnorm Layer to achieve unbiased estimation of statistics, and then proposed a network that includes a student network, a teacher network and an anchor network. Three-layer network structure to standardize TTA based on self-training. But this article still has shortcomings and room for improvement. Since a large number of experiments and starting points are based on classification tasks and BN modules, there is no need for adaptation to other tasks and Transformer-based models. The extent remains unknown. These issues deserve further research and exploration in follow-up work. The above is the detailed content of TRIBE achieves domain adaptation robustness and reaches SOTA's AAAII 2024 in multiple real-life scenarios.. For more information, please follow other related articles on the PHP Chinese website!