


PixelLM, a byte multi-modal large model that efficiently implements pixel-level reasoning without SA dependency
Multi-modal large models have exploded, are you ready to enter practical applications in fine-grained tasks such as image editing, autonomous driving and robotics?
The capabilities of most current models are still limited to generating text descriptions of the overall image or specific areas, and their capabilities in pixel-level understanding (such as object segmentation) are relatively limited.
In response to this problem, some work has begun to explore the use of multi-modal large models to process user segmentation instructions (for example, "Please segment the fruits rich in vitamin C in the picture").
However, the methods on the market all suffer from two major shortcomings:
1) cannot handle tasks involving multiple target objects, which is indispensable in real-world scenarios;
2) Relies on pre-trained image segmentation models like SAM, and the amount of calculation required for one forward propagation of SAM is enough for Llama-7B to generate more than 500 tokens.
In order to solve this problem, the Bytedance Intelligent Creation Team teamed up with researchers from Beijing Jiaotong University and University of Science and Technology Beijing to propose PixelLM, the first large-scale efficient pixel-level inference model that does not rely on SAM.
Before introducing it in detail, let’s experience the actual segmentation effect of several groups of PixelLM:
Compared with previous work, the advantages of PixelLM are:
- It can Proficiently handle any number of open domain targets and diverse complex inference segmentation tasks.
- Avoids additional and costly segmentation models, improving efficiency and migration capabilities to different applications.
Further, in order to support model training and evaluation in this research field, the research team built a data set for multi-objective reasoning segmentation scenarios based on the LVIS data set with the help of GPT-4V. MUSE, which contains more than 200,000 question-answer pairs involving more than 900,000 instance segmentation masks.
In order to achieve the above effects, how was this research conducted?
Principle behind
Picture
As shown in the framework diagram in the paper, the PixelLM architecture is very simple and consists of four main parts. The latter two are the core of PixelLM:
- Pre-trained CLIP-ViT visual encoder
- Large language model
- Lightweight pixel decoder
- Seg Codebook
The Seg codebook contains learnable tokens, which are used to encode target information at different scales of CLIP-ViT. Then, the pixel decoder generates object segmentation results based on these tokens and the image features of CLIP-ViT. Thanks to this design, PixelLM can generate high-quality segmentation results without an external segmentation model, significantly improving model efficiency.
According to the researcher's description, the tokens in the Seg codebook can be divided into L groups, each group contains N tokens, and each group corresponds to a scale from CLIP-ViT visual features.
For the input image, PixelLM extracts L scale features from the image features produced by the CLIP-ViT visual encoder. The last layer covers the global image information and will be used by LLM to understand the image content. .
The tokens of the Seg codebook will be input into the LLM together with the text instructions and the last layer of image features to produce output in the form of autoregression. The output will also include Seg codebook tokens processed by LLM, which will be input into the pixel decoder together with L scale CLIP-ViT features to produce the final segmentation result.
Picture
Picture
So why do we need to set each group to contain N tokens? ? The researchers explained in conjunction with the following figure:
In scenarios involving multiple targets or targets with very complex semantics, although LLM can provide detailed text responses, it may not be fully captured using only a single token. The entire content of the target semantics.
In order to enhance the model's ability in complex reasoning scenarios, the researchers introduced multiple tokens within each scale group and performed a linear fusion operation of one token. Before the token is passed to the decoder, a linear projection layer is used to merge the tokens within each group.
The following figure shows the effect when there are multiple tokens in each group. The attention map is what each token looks like after being processed by the decoder. This visualization shows that multiple tokens provide unique and complementary information, resulting in more effective segmentation output.
Picture
In addition, in order to enhance the model's ability to distinguish multiple targets, PixelLM also designed an additional Target Refinement Loss.
MUSE Dataset
Although the above solutions have been proposed, in order to fully utilize the capabilities of the model, the model still requires appropriate training data. Reviewing the currently available public data sets, we found that the existing data have the following major limitations:
1) Insufficient description of object details;
2) Lack of problems with complex reasoning and multiple target numbers -The answer is correct.
In order to solve these problems, the research team used GPT-4V to build an automated data annotation pipeline, and thus generated the MUSE data set. The figure below shows an example of the prompts used when generating MUSE and the data generated.
Picture
In MUSE, all instance masks are from the LVIS dataset, and additionally detailed text descriptions generated based on the image content are added. MUSE contains 246,000 question-answer pairs, and each question-answer pair involves an average of 3.7 target objects. In addition, the research team conducted an exhaustive statistical analysis of the dataset:
Category statistics: There are more than 1,000 categories in MUSE from the original LVIS dataset, and 900,000 instances with unique descriptions based on The question-answer pairs vary depending on the context. Figure (a) shows the number of instances of each category across all question-answer pairs.
Token number statistics: Figure (b) shows the distribution of the number of tokens described in the instance. Some instance descriptions contain more than 100 tokens. These descriptions are not limited to simple category names; instead, they are enriched with detailed information about each instance, including appearance, properties, and relationships to other objects, through a GPT-4V-based data generation process. The depth and diversity of information in the dataset enhances the generalization ability of the trained model, allowing it to effectively solve open domain problems.
Statistics of the number of targets: Figure (c) shows the statistics of the number of targets in each question-answer pair. The average number of targets is 3.7, and the maximum number of targets can reach 34. This number can cover most target inference scenarios for a single image.
Algorithm Evaluation
The research team evaluated the performance of PixelLM on three benchmarks, including MUSE benchmark, referring segmentation benchmark, and multi-referring segmentation benchmark. In the multi-referring segmentation benchmark, The research team requires the model to continuously segment multiple targets contained in each image in the referring segmentation benchmark in one problem.
At the same time, since PixelLM is the first model to handle complex pixel reasoning tasks involving multiple targets, the research team established four baselines to conduct comparative analysis of the models.
Three of the baselines are based on LISA, the most relevant work on PixelLM, including:
1) Original LISA;
2) LISA_rec: First enter the question into LLAVA-13B to Get the target's text reply, and then use LISA to split the text;
3) LISA_aug: directly add MUSE to the LISA training data.
4) The other is SEEM, a general segmentation model that does not use LLM.
Picture
In most indicators of the three benchmarks, PixelLM's performance is better than other methods, and because PixelLM does not rely on SAM, Its TFLOPs are far lower than models of the same size.
Interested friends can follow the wave first and wait for the code to be open source~
Reference link:
[1]https://www.php.cn/ link/9271858951e6fe9504d1f05ae8576001
[2]https://www.php.cn/link/f1686b4badcf28d33ed632036c7ab0b8
The above is the detailed content of PixelLM, a byte multi-modal large model that efficiently implements pixel-level reasoning without SA dependency. For more information, please follow other related articles on the PHP Chinese website!

Hot AI Tools

Undresser.AI Undress
AI-powered app for creating realistic nude photos

AI Clothes Remover
Online AI tool for removing clothes from photos.

Undress AI Tool
Undress images for free

Clothoff.io
AI clothes remover

AI Hentai Generator
Generate AI Hentai for free.

Hot Article

Hot Tools

Notepad++7.3.1
Easy-to-use and free code editor

SublimeText3 Chinese version
Chinese version, very easy to use

Zend Studio 13.0.1
Powerful PHP integrated development environment

Dreamweaver CS6
Visual web development tools

SublimeText3 Mac version
God-level code editing software (SublimeText3)

Hot Topics


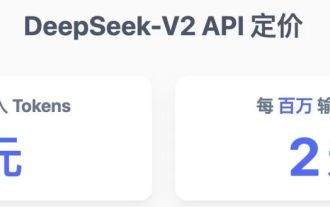
Imagine an artificial intelligence model that not only has the ability to surpass traditional computing, but also achieves more efficient performance at a lower cost. This is not science fiction, DeepSeek-V2[1], the world’s most powerful open source MoE model is here. DeepSeek-V2 is a powerful mixture of experts (MoE) language model with the characteristics of economical training and efficient inference. It consists of 236B parameters, 21B of which are used to activate each marker. Compared with DeepSeek67B, DeepSeek-V2 has stronger performance, while saving 42.5% of training costs, reducing KV cache by 93.3%, and increasing the maximum generation throughput to 5.76 times. DeepSeek is a company exploring general artificial intelligence
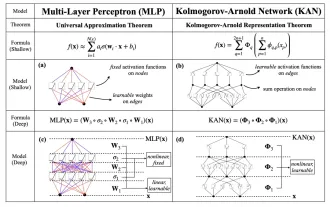
Earlier this month, researchers from MIT and other institutions proposed a very promising alternative to MLP - KAN. KAN outperforms MLP in terms of accuracy and interpretability. And it can outperform MLP running with a larger number of parameters with a very small number of parameters. For example, the authors stated that they used KAN to reproduce DeepMind's results with a smaller network and a higher degree of automation. Specifically, DeepMind's MLP has about 300,000 parameters, while KAN only has about 200 parameters. KAN has a strong mathematical foundation like MLP. MLP is based on the universal approximation theorem, while KAN is based on the Kolmogorov-Arnold representation theorem. As shown in the figure below, KAN has
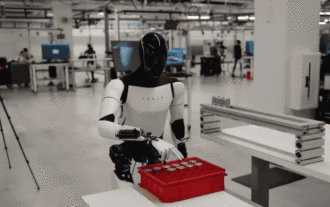
The latest video of Tesla's robot Optimus is released, and it can already work in the factory. At normal speed, it sorts batteries (Tesla's 4680 batteries) like this: The official also released what it looks like at 20x speed - on a small "workstation", picking and picking and picking: This time it is released One of the highlights of the video is that Optimus completes this work in the factory, completely autonomously, without human intervention throughout the process. And from the perspective of Optimus, it can also pick up and place the crooked battery, focusing on automatic error correction: Regarding Optimus's hand, NVIDIA scientist Jim Fan gave a high evaluation: Optimus's hand is the world's five-fingered robot. One of the most dexterous. Its hands are not only tactile
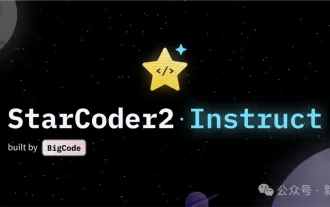
At the forefront of software technology, UIUC Zhang Lingming's group, together with researchers from the BigCode organization, recently announced the StarCoder2-15B-Instruct large code model. This innovative achievement achieved a significant breakthrough in code generation tasks, successfully surpassing CodeLlama-70B-Instruct and reaching the top of the code generation performance list. The unique feature of StarCoder2-15B-Instruct is its pure self-alignment strategy. The entire training process is open, transparent, and completely autonomous and controllable. The model generates thousands of instructions via StarCoder2-15B in response to fine-tuning the StarCoder-15B base model without relying on expensive manual annotation.
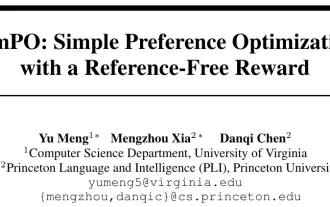
In order to align large language models (LLMs) with human values and intentions, it is critical to learn human feedback to ensure that they are useful, honest, and harmless. In terms of aligning LLM, an effective method is reinforcement learning based on human feedback (RLHF). Although the results of the RLHF method are excellent, there are some optimization challenges involved. This involves training a reward model and then optimizing a policy model to maximize that reward. Recently, some researchers have explored simpler offline algorithms, one of which is direct preference optimization (DPO). DPO learns the policy model directly based on preference data by parameterizing the reward function in RLHF, thus eliminating the need for an explicit reward model. This method is simple and stable
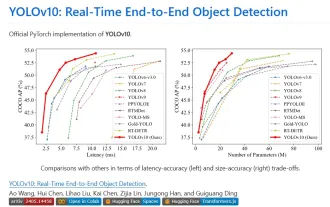
1. Introduction Over the past few years, YOLOs have become the dominant paradigm in the field of real-time object detection due to its effective balance between computational cost and detection performance. Researchers have explored YOLO's architectural design, optimization goals, data expansion strategies, etc., and have made significant progress. At the same time, relying on non-maximum suppression (NMS) for post-processing hinders end-to-end deployment of YOLO and adversely affects inference latency. In YOLOs, the design of various components lacks comprehensive and thorough inspection, resulting in significant computational redundancy and limiting the capabilities of the model. It offers suboptimal efficiency, and relatively large potential for performance improvement. In this work, the goal is to further improve the performance efficiency boundary of YOLO from both post-processing and model architecture. to this end
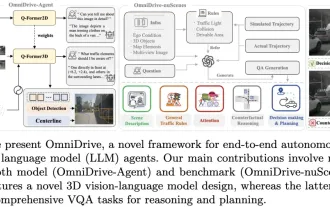
Written above & the author’s personal understanding: This paper is dedicated to solving the key challenges of current multi-modal large language models (MLLMs) in autonomous driving applications, that is, the problem of extending MLLMs from 2D understanding to 3D space. This expansion is particularly important as autonomous vehicles (AVs) need to make accurate decisions about 3D environments. 3D spatial understanding is critical for AVs because it directly impacts the vehicle’s ability to make informed decisions, predict future states, and interact safely with the environment. Current multi-modal large language models (such as LLaVA-1.5) can often only handle lower resolution image inputs (e.g.) due to resolution limitations of the visual encoder, limitations of LLM sequence length. However, autonomous driving applications require
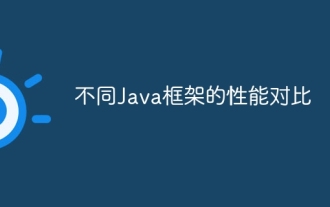
Performance comparison of different Java frameworks: REST API request processing: Vert.x is the best, with a request rate of 2 times SpringBoot and 3 times Dropwizard. Database query: SpringBoot's HibernateORM is better than Vert.x and Dropwizard's ORM. Caching operations: Vert.x's Hazelcast client is superior to SpringBoot and Dropwizard's caching mechanisms. Suitable framework: Choose according to application requirements. Vert.x is suitable for high-performance web services, SpringBoot is suitable for data-intensive applications, and Dropwizard is suitable for microservice architecture.
