


Alibaba's new mPLUG-Owl upgrade has the best of both worlds, and modal collaboration enables MLLM's new SOTA
OpenAI GPT-4V and Google Gemini have demonstrated very strong multi-modal understanding capabilities, promoting the rapid development of multi-modal large models (MLLM), and MLLM has become The hottest research direction in the industry right now.
MLLM achieves excellent instruction following ability in a variety of visual-linguistic open tasks. Although previous research on multimodal learning has shown that different modalities can collaborate and promote each other, existing MLLM research mainly focuses on improving the ability of multimodal tasks and how to balance the benefits of modal collaboration and the impact of modal interference. remains an important issue that needs to be addressed.
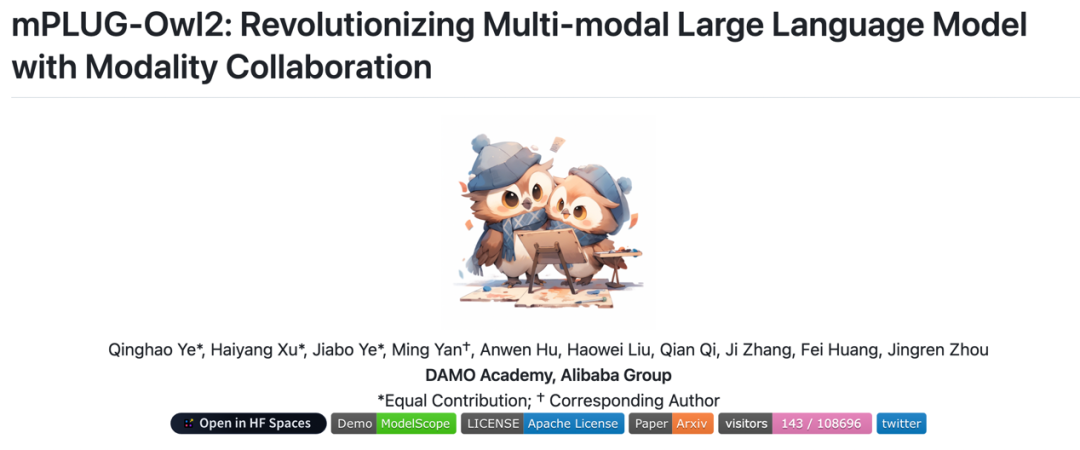
Please click the following link to view the paper: https://arxiv.org/pdf/2311.04257.pdf
-
Please check the following code address: https://github.com/X-PLUG/mPLUG-Owl/tree/main/mPLUG-Owl2
ModelScope experience address: https: //modelscope.cn/studios/damo/mPLUG-Owl2/summary
-
HuggingFace experience address link: https://huggingface.co/spaces/MAGAer13/mPLUG-Owl2
In response to this problem, Alibaba’s multi-modal large model mPLUG-Owl has received a major upgrade. Through modal collaboration, it simultaneously improves the performance of plain text and multi-modality, surpassing LLaVA1.5, MiniGPT4, Qwen-VL and other models, and achieves the best performance in a variety of tasks. Specifically, mPLUG-Owl2 utilizes shared functional modules to promote collaboration between different modalities and introduces a modal adaptation module to retain the characteristics of each modality. With a simple and effective design, mPLUG-Owl2 achieves the best performance in multiple fields including plain text and multi-modal tasks. The study of modal collaboration phenomena also provides inspiration for the future development of multi-modal large models
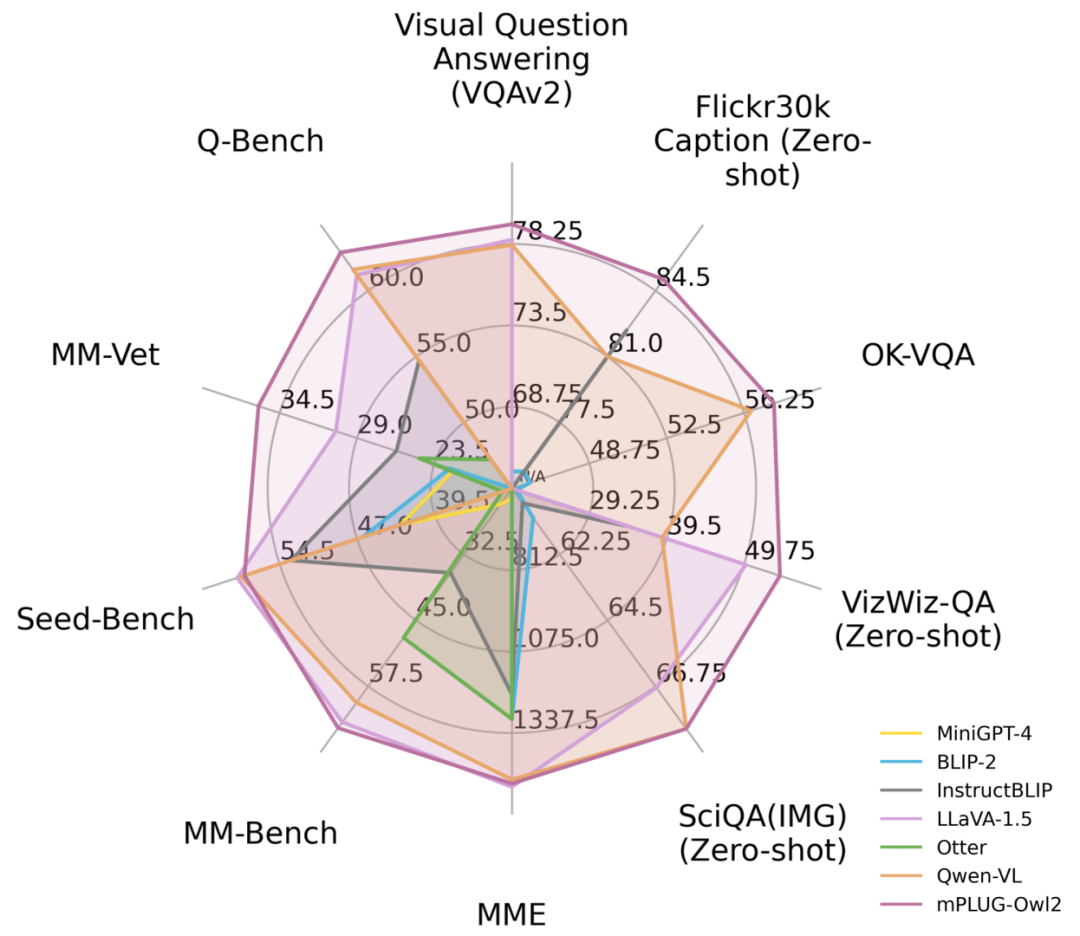
Figure 1 Performance comparison with existing MLLM models
Method introduction In order to achieve the purpose of not changing the original meaning, the content needs to be rewritten into Chinese
mPLUG-Owl2 model mainly consists of three parts:
Visual Encoder: As a visual encoder, ViT-L/14 converts the input image with a resolution of H x W into a sequence of visual tokens of H/14 x W/14 and inputs it into the Visual Abstractor.
Visual Extractor: Extract high-level semantic features by learning a set of available queries while reducing the visual sequence length of the input language model
Language model: LLaMA-2-7B is used as the text decoder, and the modal adaptation module shown in Figure 3 is designed.
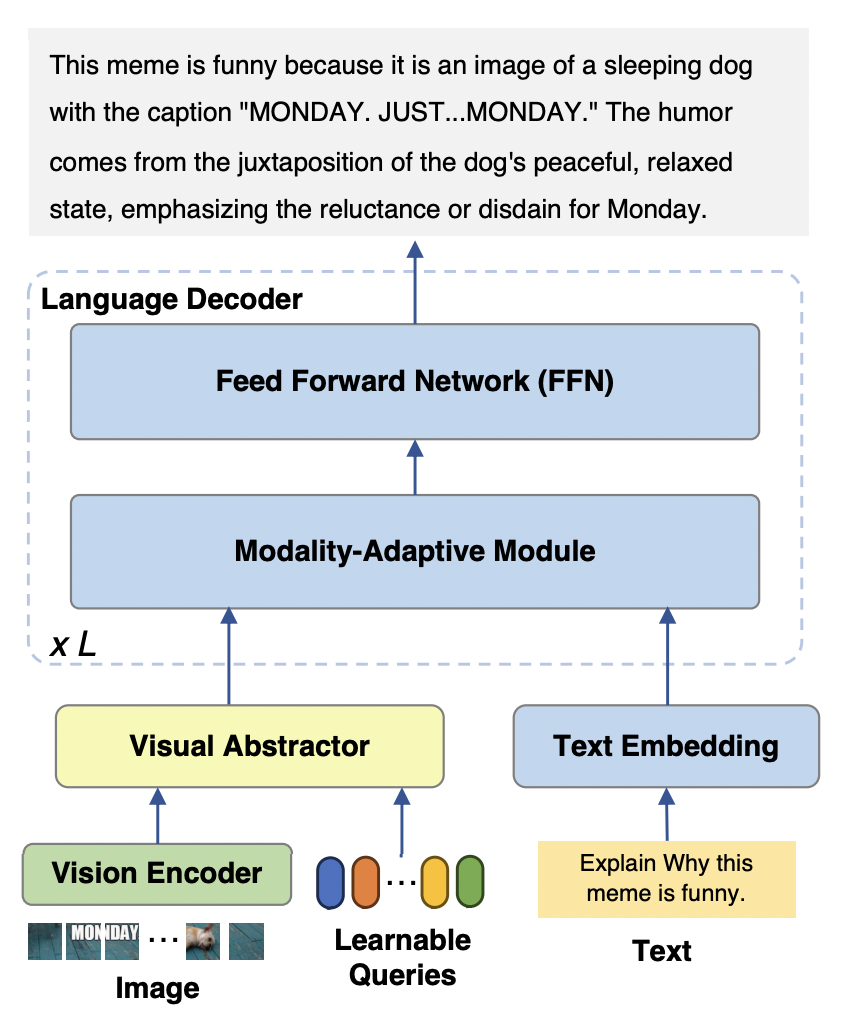
##Figure 2 mPLUG-Owl2 model structure
In order to align the visual and For language modality, existing work usually maps visual features into the semantic space of text. However, this approach ignores the respective characteristics of visual and text information and may affect the performance of the model due to the mismatch of semantic granularity. To solve this problem, this paper proposes a modality-adaptive module (MAM) to map visual and textual features into a shared semantic space, while decoupling visual-linguistic representations to retain the unique properties of each modality. .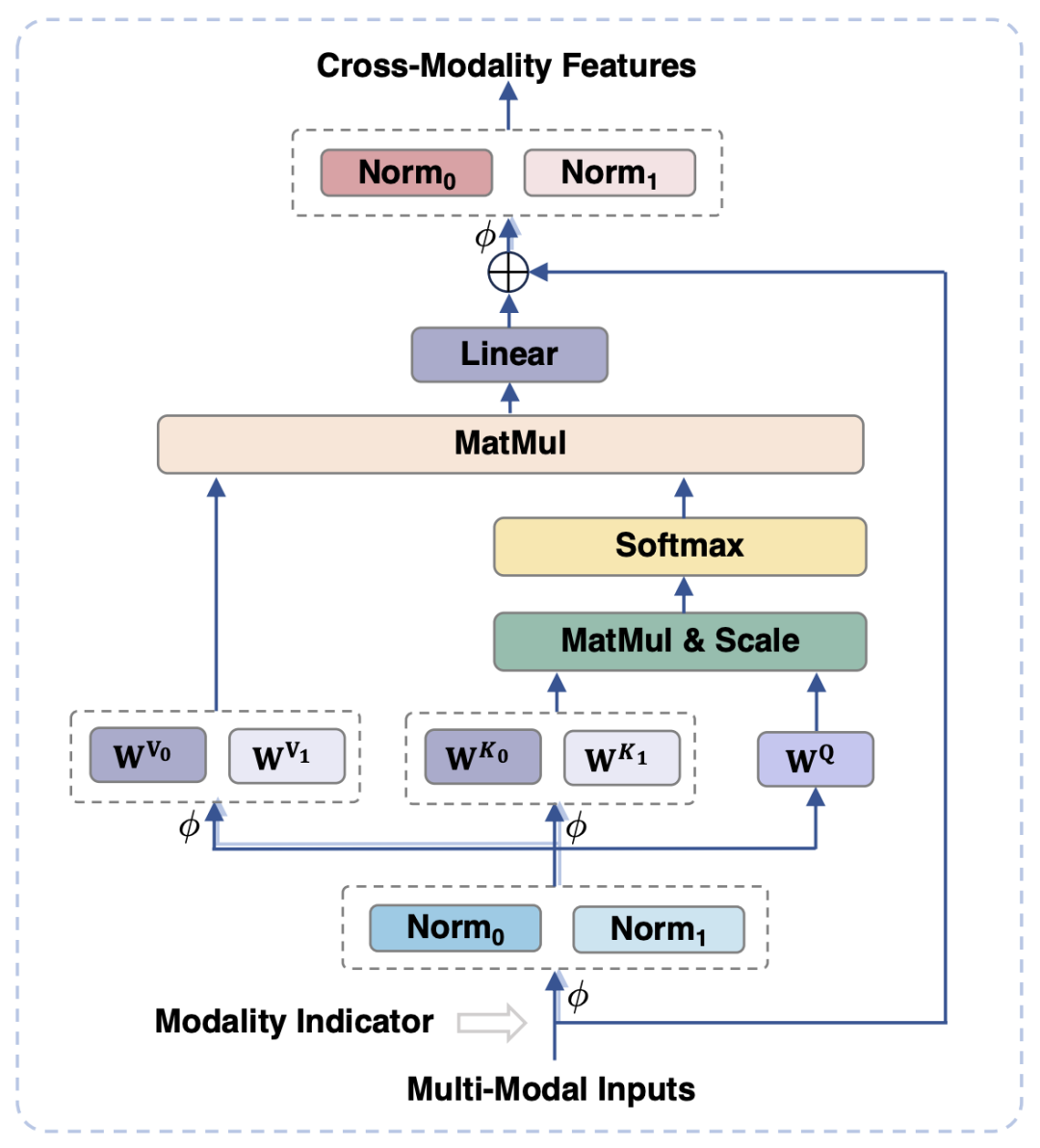
Figure 3 shows the schematic diagram of the modal adaptation module
shown in Figure 3 Yes, compared with the traditional Transformer, the main design of the modal adaptation module is:- In the input and output stages of the module, LayerNorm operations are performed on the visual and language modalities respectively. to adapt to the respective feature distributions of the two modes.
- In the self-attention operation, separate key and value projection matrices are used for visual and language modalities, but a shared query projection matrix is used to decouple the key and value projections. The matrix can avoid interference between the two modalities when the semantic granularity does not match.
- By sharing the same FFN, the two modalities can promote collaboration between each other
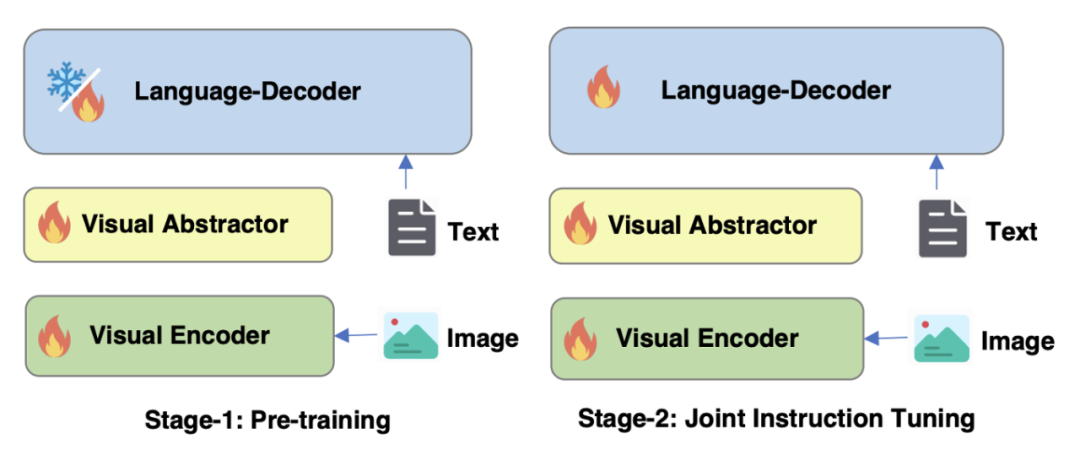
Optimize the training strategy of mPLUG-Owl2 in Figure 4
As shown in Figure 4, the training of mPLUG-Owl2 includes two stages: pre-training and instruction fine-tuning. The pre-training stage is mainly to achieve the alignment of the visual encoder and the language model. At this stage, the Visual Encoder and Visual Abstractor are trainable, and in the language model, only the visual-related model weights added by the Modality Adaptive Module are processed. renew. In the instruction fine-tuning stage, all parameters of the model are fine-tuned based on text and multi-modal instruction data (as shown in Figure 5) to improve the model's instruction following ability.
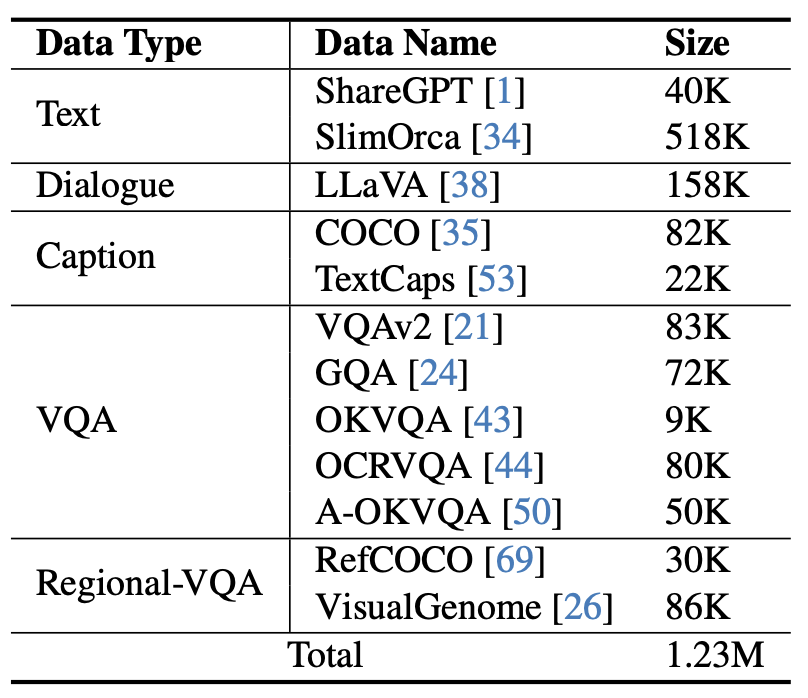
Figure 5 Instruction fine-tuning data used by mPLUG-Owl2
Experiment and results
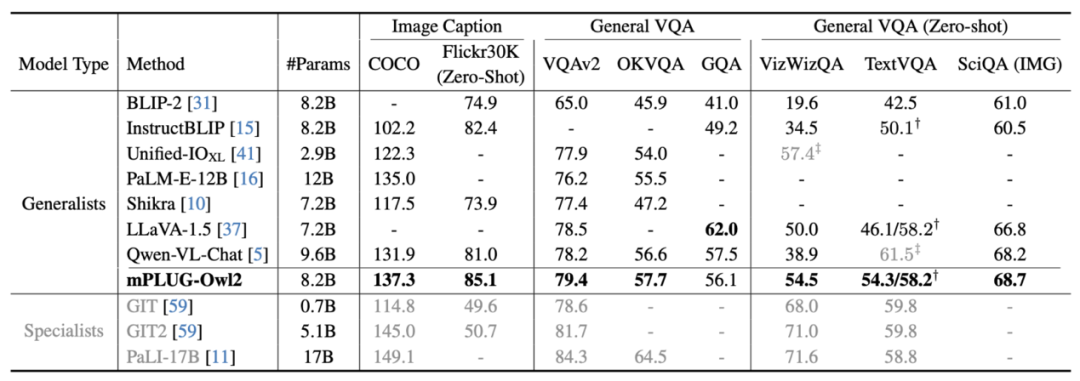
Figure 6 Image description and VQA task performance
Figure 7 MLLM benchmark performance
As shown in Figure 6 and Figure 7, whether it is traditional image description, VQA and other visual-language tasks, or MMBench, Q-Bench, etc. On benchmark data sets for multi-modal large models, mPLUG-Owl2 has achieved better performance than existing work.
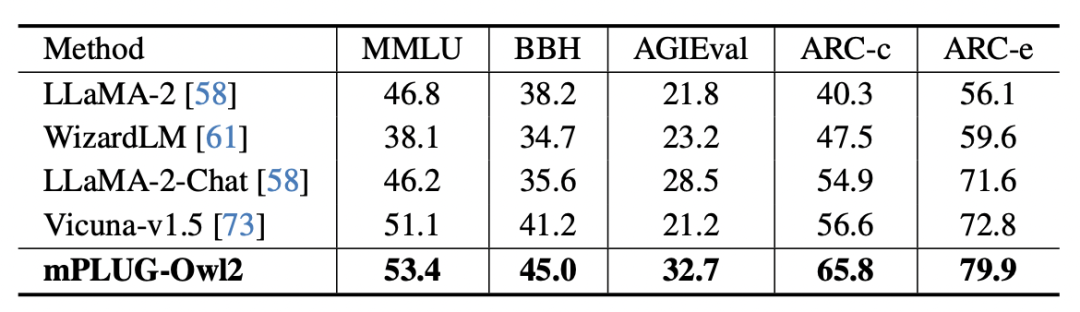
Figure 8 Plain text benchmark performance
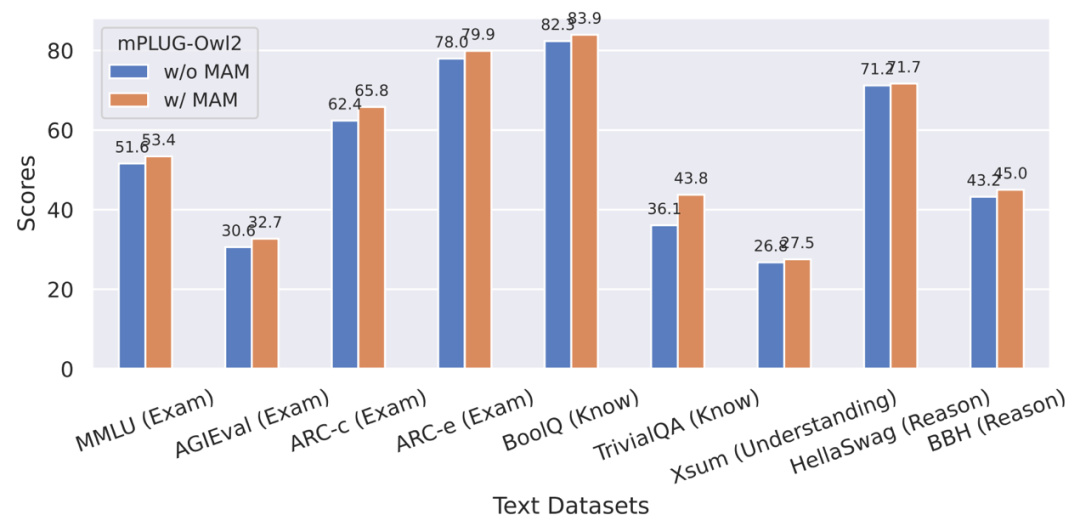
##Figure 9 The impact of modal adaptation module on the performance of plain text tasks
In addition, in order to evaluate the impact of modal collaboration on plain text tasks, the author also tested mPLUG -Owl2 performance in natural language understanding and generation. As shown in Figure 8, mPLUG-Owl2 achieves better performance compared to other instruction-fine-tuned LLMs. Figure 9 shows the performance on the plain text task. It can be seen that since the modal adaptation module promotes modal collaboration, the model's examination and knowledge capabilities have been significantly improved. The author analyzes that this is because multi-modal collaboration enables the model to use visual information to understand concepts that are difficult to describe in language, and enhances the model's reasoning ability through the rich information in the image, and indirectly strengthens the reasoning ability of the text.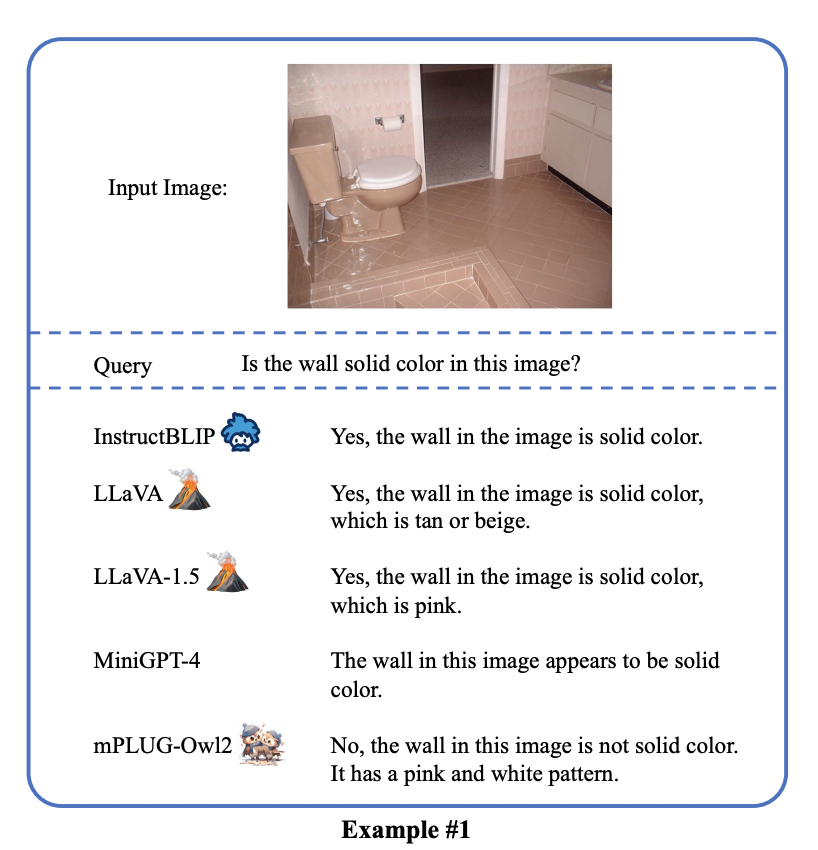
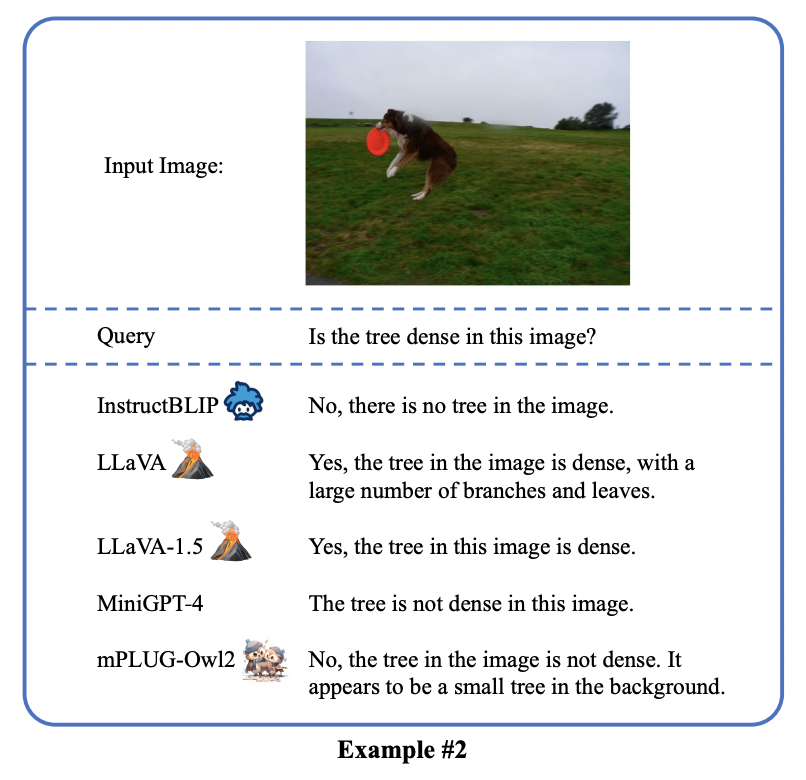
The above is the detailed content of Alibaba's new mPLUG-Owl upgrade has the best of both worlds, and modal collaboration enables MLLM's new SOTA. For more information, please follow other related articles on the PHP Chinese website!

Hot AI Tools

Undresser.AI Undress
AI-powered app for creating realistic nude photos

AI Clothes Remover
Online AI tool for removing clothes from photos.

Undress AI Tool
Undress images for free

Clothoff.io
AI clothes remover

AI Hentai Generator
Generate AI Hentai for free.

Hot Article

Hot Tools

Notepad++7.3.1
Easy-to-use and free code editor

SublimeText3 Chinese version
Chinese version, very easy to use

Zend Studio 13.0.1
Powerful PHP integrated development environment

Dreamweaver CS6
Visual web development tools

SublimeText3 Mac version
God-level code editing software (SublimeText3)

Hot Topics
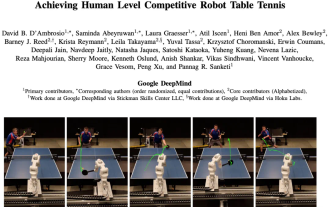
But maybe he can’t defeat the old man in the park? The Paris Olympic Games are in full swing, and table tennis has attracted much attention. At the same time, robots have also made new breakthroughs in playing table tennis. Just now, DeepMind proposed the first learning robot agent that can reach the level of human amateur players in competitive table tennis. Paper address: https://arxiv.org/pdf/2408.03906 How good is the DeepMind robot at playing table tennis? Probably on par with human amateur players: both forehand and backhand: the opponent uses a variety of playing styles, and the robot can also withstand: receiving serves with different spins: However, the intensity of the game does not seem to be as intense as the old man in the park. For robots, table tennis
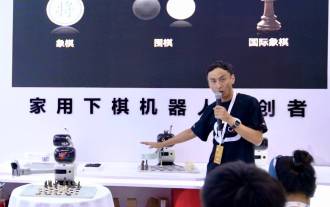
On August 21, the 2024 World Robot Conference was grandly held in Beijing. SenseTime's home robot brand "Yuanluobot SenseRobot" has unveiled its entire family of products, and recently released the Yuanluobot AI chess-playing robot - Chess Professional Edition (hereinafter referred to as "Yuanluobot SenseRobot"), becoming the world's first A chess robot for the home. As the third chess-playing robot product of Yuanluobo, the new Guoxiang robot has undergone a large number of special technical upgrades and innovations in AI and engineering machinery. For the first time, it has realized the ability to pick up three-dimensional chess pieces through mechanical claws on a home robot, and perform human-machine Functions such as chess playing, everyone playing chess, notation review, etc.
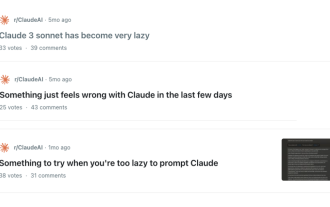
The start of school is about to begin, and it’s not just the students who are about to start the new semester who should take care of themselves, but also the large AI models. Some time ago, Reddit was filled with netizens complaining that Claude was getting lazy. "Its level has dropped a lot, it often pauses, and even the output becomes very short. In the first week of release, it could translate a full 4-page document at once, but now it can't even output half a page!" https:// www.reddit.com/r/ClaudeAI/comments/1by8rw8/something_just_feels_wrong_with_claude_in_the/ in a post titled "Totally disappointed with Claude", full of
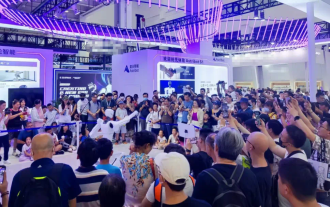
At the World Robot Conference being held in Beijing, the display of humanoid robots has become the absolute focus of the scene. At the Stardust Intelligent booth, the AI robot assistant S1 performed three major performances of dulcimer, martial arts, and calligraphy in one exhibition area, capable of both literary and martial arts. , attracted a large number of professional audiences and media. The elegant playing on the elastic strings allows the S1 to demonstrate fine operation and absolute control with speed, strength and precision. CCTV News conducted a special report on the imitation learning and intelligent control behind "Calligraphy". Company founder Lai Jie explained that behind the silky movements, the hardware side pursues the best force control and the most human-like body indicators (speed, load) etc.), but on the AI side, the real movement data of people is collected, allowing the robot to become stronger when it encounters a strong situation and learn to evolve quickly. And agile

Deep integration of vision and robot learning. When two robot hands work together smoothly to fold clothes, pour tea, and pack shoes, coupled with the 1X humanoid robot NEO that has been making headlines recently, you may have a feeling: we seem to be entering the age of robots. In fact, these silky movements are the product of advanced robotic technology + exquisite frame design + multi-modal large models. We know that useful robots often require complex and exquisite interactions with the environment, and the environment can be represented as constraints in the spatial and temporal domains. For example, if you want a robot to pour tea, the robot first needs to grasp the handle of the teapot and keep it upright without spilling the tea, then move it smoothly until the mouth of the pot is aligned with the mouth of the cup, and then tilt the teapot at a certain angle. . this
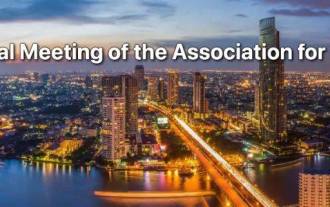
At this ACL conference, contributors have gained a lot. The six-day ACL2024 is being held in Bangkok, Thailand. ACL is the top international conference in the field of computational linguistics and natural language processing. It is organized by the International Association for Computational Linguistics and is held annually. ACL has always ranked first in academic influence in the field of NLP, and it is also a CCF-A recommended conference. This year's ACL conference is the 62nd and has received more than 400 cutting-edge works in the field of NLP. Yesterday afternoon, the conference announced the best paper and other awards. This time, there are 7 Best Paper Awards (two unpublished), 1 Best Theme Paper Award, and 35 Outstanding Paper Awards. The conference also awarded 3 Resource Paper Awards (ResourceAward) and Social Impact Award (
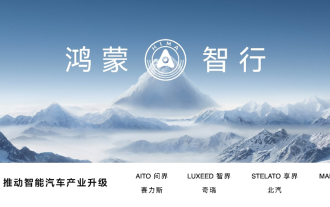
This afternoon, Hongmeng Zhixing officially welcomed new brands and new cars. On August 6, Huawei held the Hongmeng Smart Xingxing S9 and Huawei full-scenario new product launch conference, bringing the panoramic smart flagship sedan Xiangjie S9, the new M7Pro and Huawei novaFlip, MatePad Pro 12.2 inches, the new MatePad Air, Huawei Bisheng With many new all-scenario smart products including the laser printer X1 series, FreeBuds6i, WATCHFIT3 and smart screen S5Pro, from smart travel, smart office to smart wear, Huawei continues to build a full-scenario smart ecosystem to bring consumers a smart experience of the Internet of Everything. Hongmeng Zhixing: In-depth empowerment to promote the upgrading of the smart car industry Huawei joins hands with Chinese automotive industry partners to provide
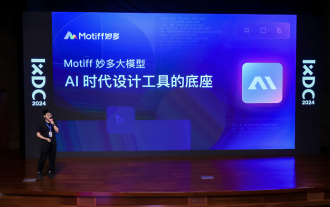
Artificial intelligence is developing faster than you might imagine. Since GPT-4 introduced multimodal technology into the public eye, multimodal large models have entered a stage of rapid development, gradually shifting from pure model research and development to exploration and application in vertical fields, and are deeply integrated with all walks of life. In the field of interface interaction, international technology giants such as Google and Apple have invested in the research and development of large multi-modal UI models, which is regarded as the only way forward for the mobile phone AI revolution. In this context, the first large-scale UI model in China was born. On August 17, at the IXDC2024 International Experience Design Conference, Motiff, a design tool in the AI era, launched its independently developed UI multi-modal model - Motiff Model. This is the world's first UI design tool
