Recently, a paper was published on arxiv, which provides a new interpretation of the mathematical principles of Transformer. The content is very long and the knowledge is a lot. It is recommended to read the original text.
In 2017, "Attention is all you need" published by Vaswani et al. became an important milestone in the development of neural network architecture. The core contribution of this paper is the self-attention mechanism, which is the innovation that distinguishes Transformers from traditional architectures and plays an important role in its excellent practical performance. In fact, this innovation has become a key catalyst for advances in artificial intelligence in areas such as computer vision and natural language processing, and also played a key role in the emergence of large language models effect. Therefore, understanding Transformers, and in particular the mechanisms by which self-attention processes data, is a crucial but largely understudied area. 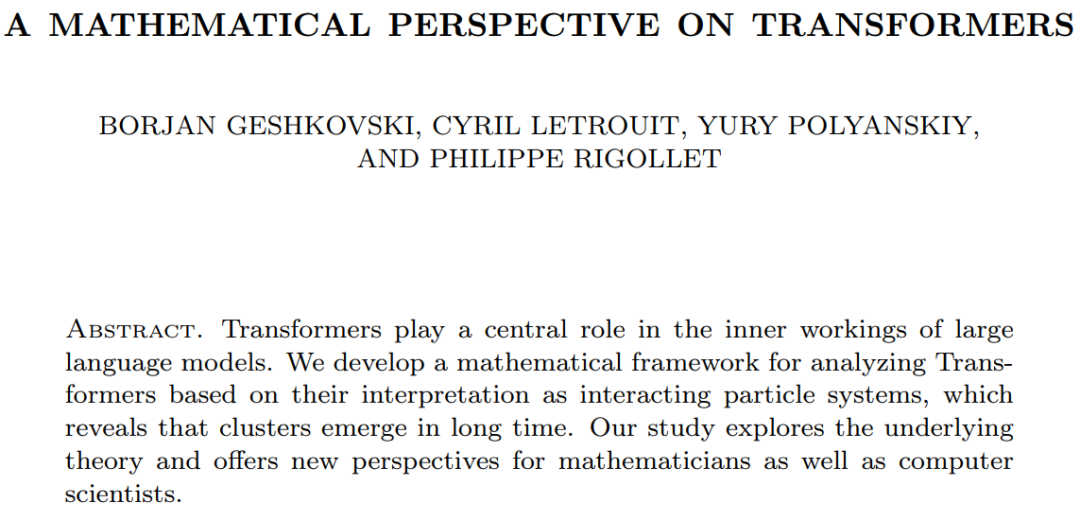
Paper address: https://arxiv.org/pdf/2312.10794.pdfDeep Neural Network ( DNNs) have a common feature: the input data is processed layer by layer in order, forming a time-discrete dynamic system (for specific content, please refer to "Deep Learning" published by MIT, also known as "Flower Book" in China). This perspective has been successfully used to model residual networks onto time-continuous dynamic systems, which are called neural ordinary differential equations (neural ODEs). In the divine constant differential equation, the input image
will evolve according to the given time-varying velocity field
at the time interval (0, T). Therefore, DNN can be regarded as a flow map (Flow Map)
from one
to another
. There is a strong similarity between flow maps even in velocity fields under the constraints of classic DNN architectures. 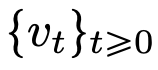
Researchers found that Transformers are actually flow mappings on
, that is, mappings between d-dimensional probability measure spaces (the space of probability measures). In order to implement this flow mapping that converts between metric spaces, Transformers need to establish a mean-field interacting particle system. 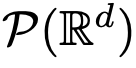
Specifically, each particle (which can be understood as a token in the context of deep learning) follows the flow of the vector field, and the flow depends on the empirical measurement of all particles ( empirical measure). In turn, the equations determine the evolution of empirical measurements of particles, a process that can last for a long time and require sustained attention.
The researchers' main observation was that particles tend to eventually clump together. This phenomenon is particularly evident in learning tasks such as one-way derivation (i.e., predicting the next word in a sequence). The output metric encodes the probability distribution of the next token, and a small number of possible results can be filtered out based on the clustering results.
The research results of this article show that the limit distribution is actually a point mass without diversity or randomness, but this is inconsistent with the actual observation results. This apparent paradox is resolved by the fact that the particles exist in variable states for long periods of time. As can be seen from Figures 2 and 4, Transformers have two different time scales: in the first stage, all tokens quickly form several clusters, while in the second stage (much slower than the first stage), through During the pairwise merging process of clusters, all tokens eventually collapse into one point.
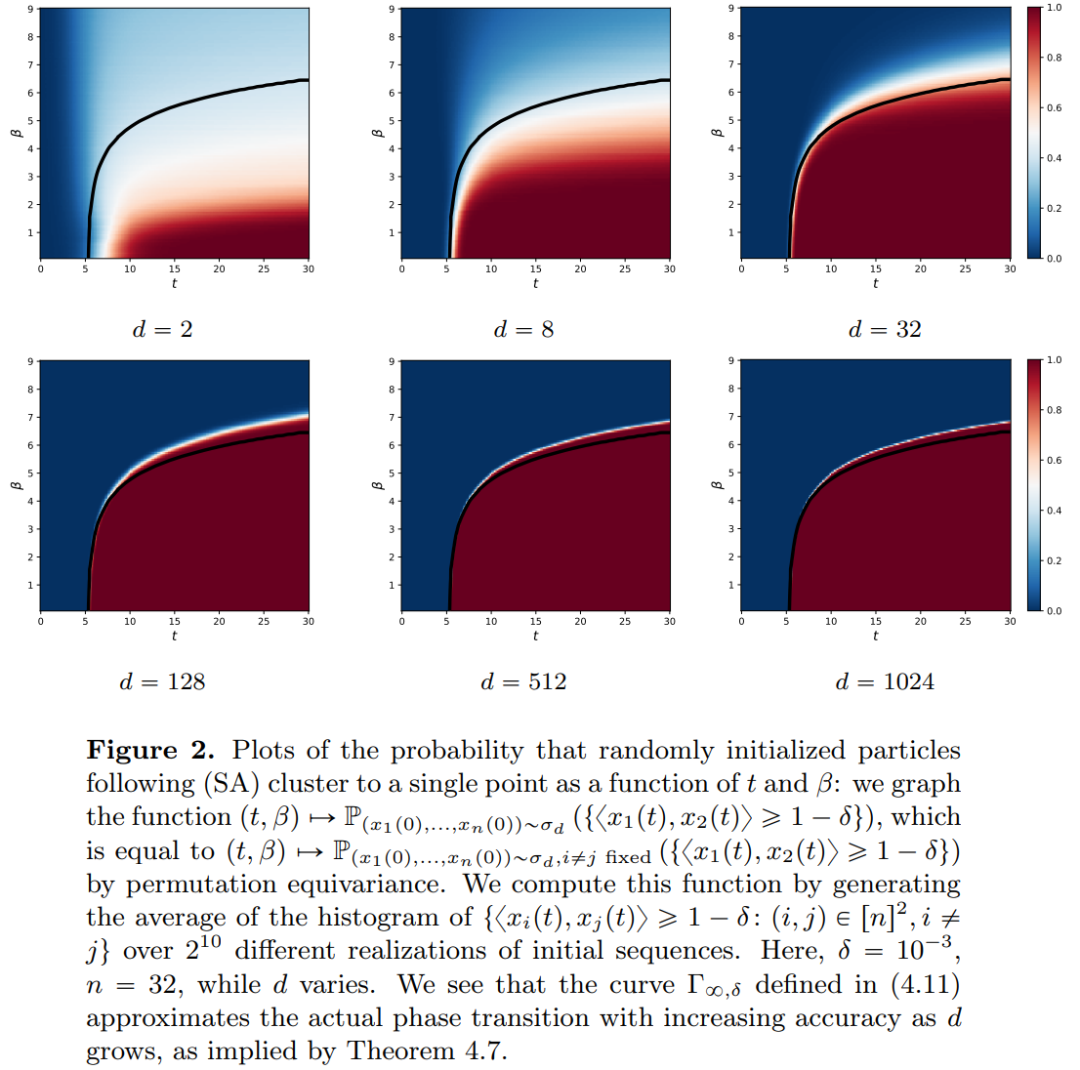
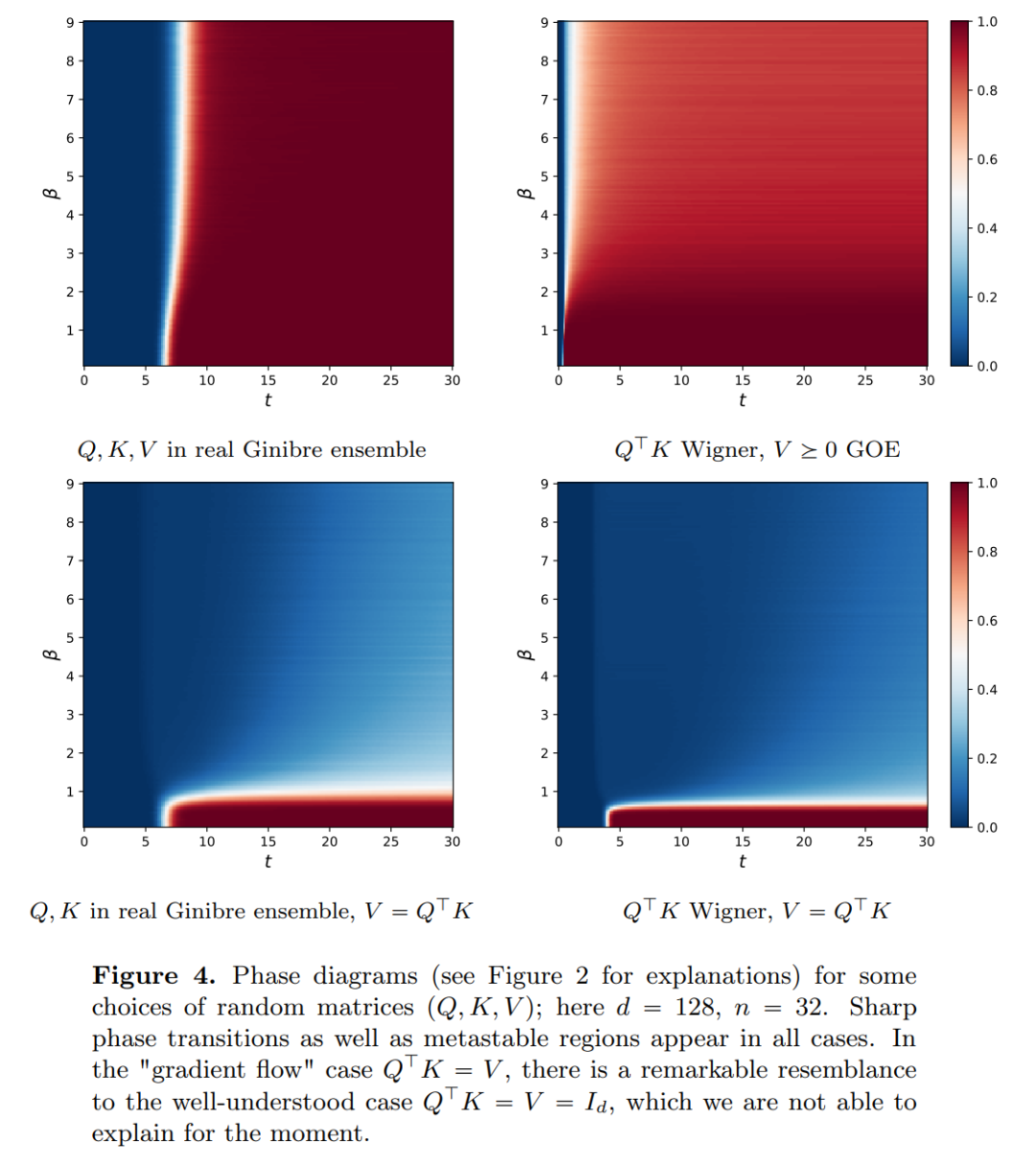
The goal of this article is twofold. On the one hand, This article aims to provide a general and easy-to-understand framework for studying Transformers from a mathematical perspective. In particular, the structure of these systems of interacting particles allows researchers to make concrete connections to established topics in mathematics, including nonlinear transport equations, Wasserstein gradient flows, models of collective behavior, and optimal configurations of points on a sphere. On the other hand, this paper describes several promising research directions, with a special focus on clustering phenomena over long time spans. The main outcome measures proposed by the researchers are new, and they also raise open questions throughout the paper that they consider interesting. The main contributions of this article are divided into three parts. 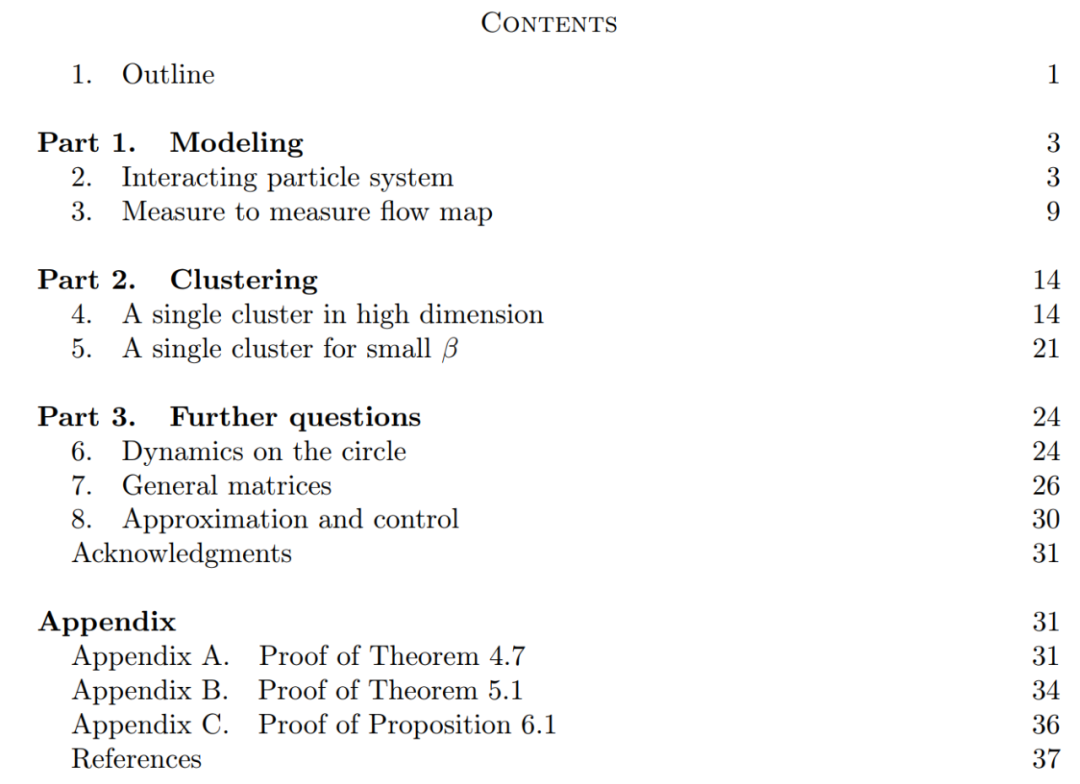
Part 1: Modeling. This article defines an ideal model of the Transformer architecture that treats the number of layers as a continuous-time variable. This approach to abstraction is not new and is similar to the approach taken by classic architectures such as ResNets. The model in this article only focuses on two key components of the Transformer architecture: self-attention mechanism and layer normalization. Layer normalization effectively limits particles to the space of the unit sphere, while the self-attention mechanism achieves nonlinear coupling between particles through empirical measurements. In turn, the empirical measure evolves according to a continuity partial differential equation. This article also introduces a simpler and easier-to-use alternative model for self-attention, a Wasserstein gradient flow of an energy function, and there are already mature research methods for the optimal configuration of points on the sphere of the energy function. 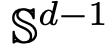
Part 2: Clustering. In this part, the researchers propose new mathematical results on token clustering over a longer time span. As Theorem 4.1 shows, in high-dimensional space, a group of n particles randomly initialized on the unit ball will gather into a point at
. The researchers' precise description of the shrinkage rate of the particle clusters complements this result. Specifically, the researchers plotted histograms of the distances between all particles, as well as the time points when all particles were about to complete clustering (see Section 4 of the original article). The researchers also obtained clustering results without assuming a large dimension d (see Section 5 of the original article). 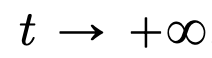
Part 3: Looking ahead. This article proposes potential lines of future research by posing primarily questions in the form of open-ended questions and substantiating them through numerical observations. The researchers first focus on the case of dimension d = 2 (see Section 6 of the original article) and draw out the connection with the Kuramoto oscillator. It is then briefly shown how difficult problems related to spherical optimization can be solved by making simple and natural modifications to the model (see Section 7 of the original article). The following chapters explore the interacting particle systems that make it possible to adjust parameters in the Transformer architecture, which may later lead to practical applications.
The above is the detailed content of Revealed new version: Mathematical principles of Transformer that you have never seen before. For more information, please follow other related articles on the PHP Chinese website!