Tips and methods for optimizing pandas data analysis
pandas tips and tricks to improve data analysis efficiency
Introduction
In the field of modern data analysis, pandas is a very widely used Python library . It provides efficient, flexible and rich data structures and data processing tools, making data analysis simpler and more efficient. However, to truly realize the potential of pandas, it's crucial to know a few tips and tricks. This article will introduce some pandas techniques to improve the efficiency of data analysis and provide specific code examples.
- Use vectorized operations
In data analysis, it is often necessary to perform various calculations and operations on data, such as addition, subtraction, multiplication and division, average calculation, group statistics, etc. . Using vectorization operations can greatly increase the speed of data processing. Many functions in pandas support vectorization operations, such as addition operation add, subtraction operation sub, multiplication operation mul, division operation div, etc. The following is a simple example:
import pandas as pd df = pd.DataFrame({'A': [1, 2, 3, 4], 'B': [5, 6, 7, 8]}) df['C'] = df['A'] + df['B'] # 使用向量化的加法操作 print(df)
Output:
A B C 0 1 5 6 1 2 6 8 2 3 7 10 3 4 8 12
- Use conditions to select data
When processing data, it is often necessary to based on certain conditions Select the required part from the data set. This function can be easily achieved using conditional selection. Pandas provides a way to select data using conditions, using Boolean indexing. The following is an example:
import pandas as pd df = pd.DataFrame({'A': [1, 2, 3, 4], 'B': [5, 6, 7, 8]}) df_selected = df[df['A'] > 2] # 选择A列中大于2的行 print(df_selected)
Output:
A B 2 3 7 3 4 8
- Using pivot tables for data grouping and aggregation
Pivot tables in pandas are a very Convenient data grouping and aggregation tools. Through pivot tables, you can easily group data according to specified columns and perform aggregate statistics on other columns. Here is an example:
import pandas as pd df = pd.DataFrame({'A': ['foo', 'bar', 'foo', 'bar', 'foo', 'bar'], 'B': ['one', 'one', 'two', 'two', 'two', 'one'], 'C': [1, 2, 3, 4, 5, 6]}) df_pivot = df.pivot_table(values='C', index='A', columns='B', aggfunc='sum') print(df_pivot)
Output:
B one two A bar 7 6 foo 6 8
- Use the apply function for custom operations
Sometimes, some customization of the data is required operation. In pandas, you can use the apply function to achieve this purpose. The apply function can accept a custom function as a parameter and apply it to each row or column of the data set. The following is an example:
import pandas as pd df = pd.DataFrame({'A': [1, 2, 3, 4], 'B': [5, 6, 7, 8]}) def custom_operation(row): return row['A'] + row['B'] df['C'] = df.apply(custom_operation, axis=1) print(df)
Output:
A B C 0 1 5 6 1 2 6 8 2 3 7 10 3 4 8 12
Conclusion
This article introduces several pandas tips and tricks to improve the efficiency of data analysis, including using vectorization operations, utilizing Conditionally select data, use pivot tables for data grouping and aggregation, and use the apply function for custom operations. By mastering these skills, you can perform data analysis work more efficiently and improve work efficiency. Of course, this is only part of the functionality of pandas, and there are many other powerful features waiting for us to explore. I hope this article can inspire readers and play a greater role in daily data analysis work.
The above is the detailed content of Tips and methods for optimizing pandas data analysis. For more information, please follow other related articles on the PHP Chinese website!

Hot AI Tools

Undresser.AI Undress
AI-powered app for creating realistic nude photos

AI Clothes Remover
Online AI tool for removing clothes from photos.

Undress AI Tool
Undress images for free

Clothoff.io
AI clothes remover

AI Hentai Generator
Generate AI Hentai for free.

Hot Article

Hot Tools

Notepad++7.3.1
Easy-to-use and free code editor

SublimeText3 Chinese version
Chinese version, very easy to use

Zend Studio 13.0.1
Powerful PHP integrated development environment

Dreamweaver CS6
Visual web development tools

SublimeText3 Mac version
God-level code editing software (SublimeText3)

Hot Topics


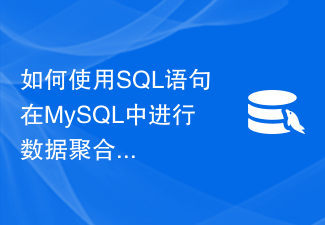
How to use SQL statements for data aggregation and statistics in MySQL? Data aggregation and statistics are very important steps when performing data analysis and statistics. As a powerful relational database management system, MySQL provides a wealth of aggregation and statistical functions, which can easily perform data aggregation and statistical operations. This article will introduce the method of using SQL statements to perform data aggregation and statistics in MySQL, and provide specific code examples. 1. Use the COUNT function for counting. The COUNT function is the most commonly used
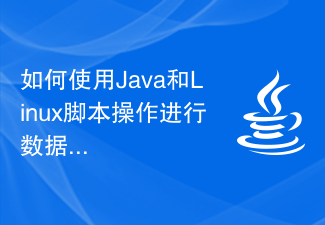
How to use Java and Linux script operations for data cleaning requires specific code examples. Data cleaning is a very important step in the data analysis process. It involves operations such as filtering data, clearing invalid data, and processing missing values. In this article, we will introduce how to use Java and Linux scripts for data cleaning, and provide specific code examples. 1. Use Java for data cleaning. Java is a high-level programming language widely used in software development. It provides a rich class library and powerful functions, which is very suitable for
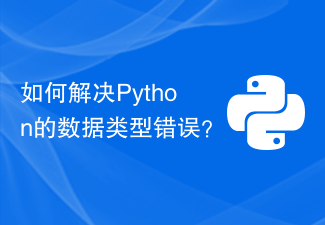
Python is a high-level programming language that is widely used in fields such as data science, machine learning, and artificial intelligence. Due to its easy-to-learn and easy-to-use nature, Python has become one of the most popular programming languages. However, like other programming languages, Python encounters various type errors when processing data. These errors may cause program execution to fail and, if not identified and resolved in time, will waste valuable developer time and resources. This article will introduce ways to solve Python data type errors. 1.Data type
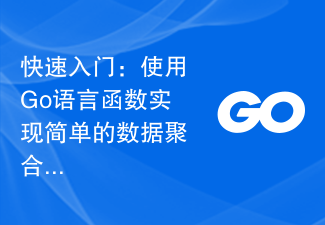
Quick Start: Use Go language functions to implement simple data aggregation functions. In software development, we often encounter situations where we need to aggregate a set of data. Aggregation operations can count, summarize, calculate, etc., to analyze and display data. In the Go language, we can use functions to implement simple data aggregation functions. First, we need to define a data type to represent the data we want to aggregate. Suppose we have a student's grade table, and each student has two fields: name and grade, then we can create the following structure
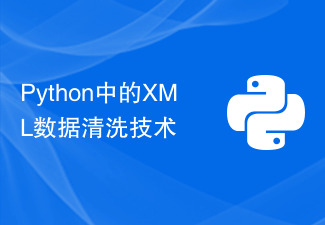
Introduction to XML data cleaning technology in Python: With the rapid development of the Internet, data is generated faster and faster. As a widely used data exchange format, XML (Extensible Markup Language) plays an important role in various fields. However, due to the complexity and diversity of XML data, effective cleaning and processing of large amounts of XML data has become a very challenging task. Fortunately, Python provides some powerful libraries and tools that allow us to easily perform XML data processing.
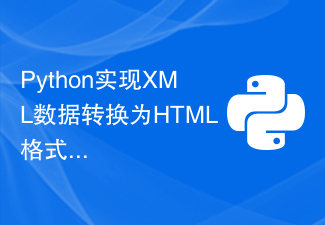
Python implements the conversion of XML data into HTML format. In the process of network development and data processing, XML (Extensible Markup Language) is a common data transmission and storage format. HTML (Hypertext Markup Language) is a standard format for displaying and laying out web pages. In some cases, we need to convert XML data into HTML format for direct display on the web page. This article will introduce how to use Python to implement this conversion process. First, we need to understand some basic XML and HTML
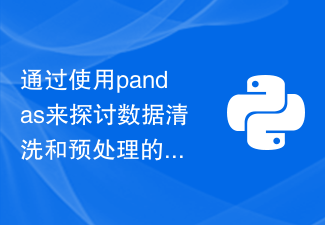
Discussion on methods of data cleaning and preprocessing using pandas Introduction: In data analysis and machine learning, data cleaning and preprocessing are very important steps. As a powerful data processing library in Python, pandas has rich functions and flexible operations, which can help us efficiently clean and preprocess data. This article will explore several commonly used pandas methods and provide corresponding code examples. 1. Data reading First, we need to read the data file. pandas provides many functions
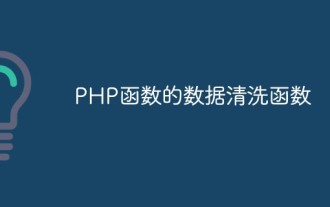
As website and application development becomes more common, it becomes increasingly important to secure user-entered data. In PHP, many data cleaning and validation functions are available to ensure that user-supplied data is correct, safe, and legal. This article will introduce some commonly used PHP functions and how to use them to clean data to reduce security issues. filter_var() The filter_var() function can be used to verify and clean different types of data, such as email, URL, integer, float
