Methods for building multimodal RAG systems: using CLIP and LLM
We will discuss ways to build a retrieval-augmented generation (RAG) system using the open source Large Language Multi-Modal. Our focus is to achieve this without relying on LangChain or LLlama index to avoid adding more framework dependencies.
What is RAG
In the field of artificial intelligence, retrieval-augmented generation, The emergence of RAG technology has brought revolutionary improvements to large language models (Large Language Models). The essence of RAG is to enhance the responsiveness of artificial intelligence by allowing models to dynamically retrieve real-time information from external sources. The introduction of this technology enables AI to respond to user needs more specifically. By retrieving and fusing information from external sources, RAG is able to generate more accurate and comprehensive answers, providing users with more valuable content. This improvement in capabilities has brought broader prospects to the application fields of artificial intelligence, including intelligent customer service, intelligent search and knowledge question and answer systems. The emergence of RAG marks the further development of language models, bringing artificial intelligence
This architecture seamlessly combines the dynamic retrieval process with generation capabilities, enabling artificial intelligence to adapt to various fields constantly changing information. Unlike fine-tuning and retraining, RAG provides a cost-effective solution that allows AI to obtain the latest and relevant information without changing the entire model. This combination of capabilities gives RAG an advantage in responding to rapidly changing information environments.
The role of RAG
1. Improve accuracy and reliability:
By Large Language Models (LLMs) direct to reliable sources of knowledge, solving the problem of its unpredictability and reducing the risk of providing false or outdated information, making responses more accurate and reliable.
2. Increase transparency and trust:
Generative AI models like LLM often lack transparency, which makes it difficult for people to trust them. output. RAG addresses concerns about bias, reliability and compliance by providing greater control.
3. Reduce hallucinations:
LLM is prone to hallucinatory reactions - providing coherent but inaccurate or fabricated information. RAG reduces the risk of misleading advice to key sectors by relying on authoritative sources to ensure responsiveness.
4. Cost-effective adaptability:
RAG provides a cost-effective way to improve AI output without the need for Extensive retraining/fine-tuning. Information can be kept up-to-date and relevant by dynamically retrieving specific details as needed, ensuring the adaptability of AI to changing information.
Multimodal modal model
Multimodal involves having multiple inputs and combining them into a single output, taking CLIP as an example : The training data of CLIP is text-image pairs. Through comparative learning, the model can learn the matching relationship of text-image pairs.
This model generates the same (very similar) embedding vectors for different inputs that represent the same thing.
##Multi-mode
Multi-modal large language
GPT4v and Gemini vision explore multi-modal large language that integrates various data types (including images, text, language, audio, etc.) Modal Language Model (MLLM). While large language models (LLMs) like GPT-3, BERT, and RoBERTa perform well on text-based tasks, they face challenges in understanding and processing other data types. To address this limitation, multimodal models combine different modalities to enable a more comprehensive understanding of different data.
Multimodal large language model It goes beyond traditional text-based methods. Taking GPT-4 as an example, these models can seamlessly process various data types, including images and text, to understand the information more comprehensively.
Combined with RAG
Here we will use Clip to embed images and text, storing these embeds in the ChromDB vector database. The large model will then be leveraged to engage in user chat sessions based on the retrieved information.
We will use images from Kaggle and information from Wikipedia to create a flower expert chatbot
First we install the software package:
! pip install -q timm einops wikipedia chromadb open_clip_torch !pip install -q transformers==4.36.0 !pip install -q bitsandbytes==0.41.3 accelerate==0.25.0
The steps to preprocess the data are very simple. Just put the images and text in a folder
可以随意使用任何矢量数据库,这里我们使用ChromaDB。
import chromadb from chromadb.utils.embedding_functions import OpenCLIPEmbeddingFunction from chromadb.utils.data_loaders import ImageLoader from chromadb.config import Settings client = chromadb.PersistentClient(path="DB") embedding_function = OpenCLIPEmbeddingFunction() image_loader = ImageLoader() # must be if you reads from URIs
ChromaDB需要自定义嵌入函数
from chromadb import Documents, EmbeddingFunction, Embeddings class MyEmbeddingFunction(EmbeddingFunction):def __call__(self, input: Documents) -> Embeddings:# embed the documents somehow or imagesreturn embeddings
这里将创建2个集合,一个用于文本,另一个用于图像
collection_images = client.create_collection(name='multimodal_collection_images', embedding_functinotallow=embedding_function, data_loader=image_loader) collection_text = client.create_collection(name='multimodal_collection_text', embedding_functinotallow=embedding_function, ) # Get the Images IMAGE_FOLDER = '/kaggle/working/all_data' image_uris = sorted([os.path.join(IMAGE_FOLDER, image_name) for image_name in os.listdir(IMAGE_FOLDER) if not image_name.endswith('.txt')]) ids = [str(i) for i in range(len(image_uris))] collection_images.add(ids=ids, uris=image_uris) #now we have the images collection
对于Clip,我们可以像这样使用文本检索图像
from matplotlib import pyplot as plt retrieved = collection_images.query(query_texts=["tulip"], include=['data'], n_results=3) for img in retrieved['data'][0]:plt.imshow(img)plt.axis("off")plt.show()
也可以使用图像检索相关的图像
文本集合如下所示
# now the text DB from chromadb.utils import embedding_functions default_ef = embedding_functions.DefaultEmbeddingFunction() text_pth = sorted([os.path.join(IMAGE_FOLDER, image_name) for image_name in os.listdir(IMAGE_FOLDER) if image_name.endswith('.txt')]) list_of_text = [] for text in text_pth:with open(text, 'r') as f:text = f.read()list_of_text.append(text) ids_txt_list = ['id'+str(i) for i in range(len(list_of_text))] ids_txt_list collection_text.add(documents = list_of_text,ids =ids_txt_list )
然后使用上面的文本集合获取嵌入
results = collection_text.query(query_texts=["What is the bellflower?"],n_results=1 ) results
结果如下:
{'ids': [['id0']],'distances': [[0.6072186183744086]],'metadatas': [[None]],'embeddings': None,'documents': [['Campanula () is the type genus of the Campanulaceae family of flowering plants. Campanula are commonly known as bellflowers and take both their common and scientific names from the bell-shaped flowers—campanula is Latin for "little bell".\nThe genus includes over 500 species and several subspecies, distributed across the temperate and subtropical regions of the Northern Hemisphere, with centers of diversity in the Mediterranean region, Balkans, Caucasus and mountains of western Asia. The range also extends into mountains in tropical regions of Asia and Africa.\nThe species include annual, biennial and perennial plants, and vary in habit from dwarf arctic and alpine species under 5 cm high, to large temperate grassland and woodland species growing to 2 metres (6 ft 7 in) tall.']],'uris': None,'data': None}
或使用图片获取文本
query_image = '/kaggle/input/flowers/flowers/rose/00f6e89a2f949f8165d5222955a5a37d.jpg' raw_image = Image.open(query_image) doc = collection_text.query(query_embeddings=embedding_function(query_image), n_results=1, )['documents'][0][0]
上图的结果如下:
A rose is either a woody perennial flowering plant of the genus Rosa (), in the family Rosaceae (), or the flower it bears. There are over three hundred species and tens of thousands of cultivars. They form a group of plants that can be erect shrubs, climbing, or trailing, with stems that are often armed with sharp prickles. Their flowers vary in size and shape and are usually large and showy, in colours ranging from white through yellows and reds. Most species are native to Asia, with smaller numbers native to Europe, North America, and northwestern Africa. Species, cultivars and hybrids are all widely grown for their beauty and often are fragrant. Roses have acquired cultural significance in many societies. Rose plants range in size from compact, miniature roses, to climbers that can reach seven meters in height. Different species hybridize easily, and this has been used in the development of the wide range of garden roses.
这样我们就完成了文本和图像的匹配工作,其实这里都是CLIP的工作,下面我们开始加入LLM。
from huggingface_hub import hf_hub_download hf_hub_download(repo_, filename="configuration_llava.py", local_dir="./", force_download=True) hf_hub_download(repo_, filename="configuration_phi.py", local_dir="./", force_download=True) hf_hub_download(repo_, filename="modeling_llava.py", local_dir="./", force_download=True) hf_hub_download(repo_, filename="modeling_phi.py", local_dir="./", force_download=True) hf_hub_download(repo_, filename="processing_llava.py", local_dir="./", force_download=True)
我们是用visheratin/LLaVA-3b
from modeling_llava import LlavaForConditionalGeneration import torch model = LlavaForConditionalGeneration.from_pretrained("visheratin/LLaVA-3b") model = model.to("cuda")
加载tokenizer
from transformers import AutoTokenizer tokenizer = AutoTokenizer.from_pretrained("visheratin/LLaVA-3b")
然后定义处理器,方便我们以后调用
from processing_llava import LlavaProcessor, OpenCLIPImageProcessor image_processor = OpenCLIPImageProcessor(model.config.preprocess_config) processor = LlavaProcessor(image_processor, tokenizer)
下面就可以直接使用了
question = 'Answer with organized answers: What type of rose is in the picture? Mention some of its characteristics and how to take care of it ?' query_image = '/kaggle/input/flowers/flowers/rose/00f6e89a2f949f8165d5222955a5a37d.jpg' raw_image = Image.open(query_image) doc = collection_text.query(query_embeddings=embedding_function(query_image), n_results=1, )['documents'][0][0] plt.imshow(raw_image) plt.show() imgs = collection_images.query(query_uris=query_image, include=['data'], n_results=3) for img in imgs['data'][0][1:]:plt.imshow(img)plt.axis("off")plt.show()
得到的结果如下:
结果还包含了我们需要的大部分信息
这样我们整合就完成了,最后就是创建聊天模板,
prompt = """system A chat between a curious human and an artificial intelligence assistant. The assistant is an exprt in flowers , and gives helpful, detailed, and polite answers to the human's questions. The assistant does not hallucinate and pays very close attention to the details. user <image> {question} Use the following article as an answer source. Do not write outside its scope unless you find your answer better {article} if you thin your answer is better add it after document. assistant """.format(questinotallow='question', article=doc)</image>
如何创建聊天过程我们这里就不详细介绍了,完整代码在这里:
https://www.php.cn/link/71eee742e4c6e094e6af364597af3f05
The above is the detailed content of Methods for building multimodal RAG systems: using CLIP and LLM. For more information, please follow other related articles on the PHP Chinese website!

Hot AI Tools

Undresser.AI Undress
AI-powered app for creating realistic nude photos

AI Clothes Remover
Online AI tool for removing clothes from photos.

Undress AI Tool
Undress images for free

Clothoff.io
AI clothes remover

AI Hentai Generator
Generate AI Hentai for free.

Hot Article

Hot Tools

Notepad++7.3.1
Easy-to-use and free code editor

SublimeText3 Chinese version
Chinese version, very easy to use

Zend Studio 13.0.1
Powerful PHP integrated development environment

Dreamweaver CS6
Visual web development tools

SublimeText3 Mac version
God-level code editing software (SublimeText3)

Hot Topics


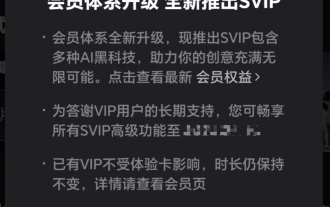
This site reported on June 27 that Jianying is a video editing software developed by FaceMeng Technology, a subsidiary of ByteDance. It relies on the Douyin platform and basically produces short video content for users of the platform. It is compatible with iOS, Android, and Windows. , MacOS and other operating systems. Jianying officially announced the upgrade of its membership system and launched a new SVIP, which includes a variety of AI black technologies, such as intelligent translation, intelligent highlighting, intelligent packaging, digital human synthesis, etc. In terms of price, the monthly fee for clipping SVIP is 79 yuan, the annual fee is 599 yuan (note on this site: equivalent to 49.9 yuan per month), the continuous monthly subscription is 59 yuan per month, and the continuous annual subscription is 499 yuan per year (equivalent to 41.6 yuan per month) . In addition, the cut official also stated that in order to improve the user experience, those who have subscribed to the original VIP
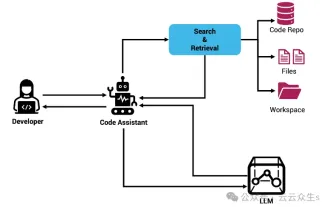
Improve developer productivity, efficiency, and accuracy by incorporating retrieval-enhanced generation and semantic memory into AI coding assistants. Translated from EnhancingAICodingAssistantswithContextUsingRAGandSEM-RAG, author JanakiramMSV. While basic AI programming assistants are naturally helpful, they often fail to provide the most relevant and correct code suggestions because they rely on a general understanding of the software language and the most common patterns of writing software. The code generated by these coding assistants is suitable for solving the problems they are responsible for solving, but often does not conform to the coding standards, conventions and styles of the individual teams. This often results in suggestions that need to be modified or refined in order for the code to be accepted into the application
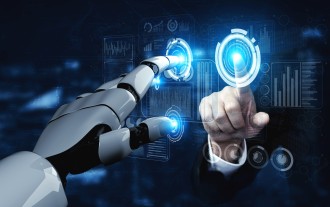
Large Language Models (LLMs) are trained on huge text databases, where they acquire large amounts of real-world knowledge. This knowledge is embedded into their parameters and can then be used when needed. The knowledge of these models is "reified" at the end of training. At the end of pre-training, the model actually stops learning. Align or fine-tune the model to learn how to leverage this knowledge and respond more naturally to user questions. But sometimes model knowledge is not enough, and although the model can access external content through RAG, it is considered beneficial to adapt the model to new domains through fine-tuning. This fine-tuning is performed using input from human annotators or other LLM creations, where the model encounters additional real-world knowledge and integrates it

To learn more about AIGC, please visit: 51CTOAI.x Community https://www.51cto.com/aigc/Translator|Jingyan Reviewer|Chonglou is different from the traditional question bank that can be seen everywhere on the Internet. These questions It requires thinking outside the box. Large Language Models (LLMs) are increasingly important in the fields of data science, generative artificial intelligence (GenAI), and artificial intelligence. These complex algorithms enhance human skills and drive efficiency and innovation in many industries, becoming the key for companies to remain competitive. LLM has a wide range of applications. It can be used in fields such as natural language processing, text generation, speech recognition and recommendation systems. By learning from large amounts of data, LLM is able to generate text
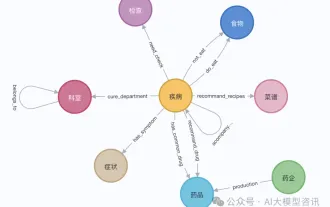
Graph Retrieval Enhanced Generation (GraphRAG) is gradually becoming popular and has become a powerful complement to traditional vector search methods. This method takes advantage of the structural characteristics of graph databases to organize data in the form of nodes and relationships, thereby enhancing the depth and contextual relevance of retrieved information. Graphs have natural advantages in representing and storing diverse and interrelated information, and can easily capture complex relationships and properties between different data types. Vector databases are unable to handle this type of structured information, and they focus more on processing unstructured data represented by high-dimensional vectors. In RAG applications, combining structured graph data and unstructured text vector search allows us to enjoy the advantages of both at the same time, which is what this article will discuss. structure
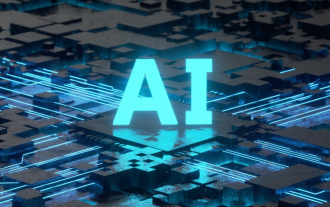
Editor |ScienceAI Question Answering (QA) data set plays a vital role in promoting natural language processing (NLP) research. High-quality QA data sets can not only be used to fine-tune models, but also effectively evaluate the capabilities of large language models (LLM), especially the ability to understand and reason about scientific knowledge. Although there are currently many scientific QA data sets covering medicine, chemistry, biology and other fields, these data sets still have some shortcomings. First, the data form is relatively simple, most of which are multiple-choice questions. They are easy to evaluate, but limit the model's answer selection range and cannot fully test the model's ability to answer scientific questions. In contrast, open-ended Q&A
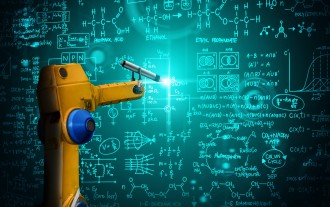
Machine learning is an important branch of artificial intelligence that gives computers the ability to learn from data and improve their capabilities without being explicitly programmed. Machine learning has a wide range of applications in various fields, from image recognition and natural language processing to recommendation systems and fraud detection, and it is changing the way we live. There are many different methods and theories in the field of machine learning, among which the five most influential methods are called the "Five Schools of Machine Learning". The five major schools are the symbolic school, the connectionist school, the evolutionary school, the Bayesian school and the analogy school. 1. Symbolism, also known as symbolism, emphasizes the use of symbols for logical reasoning and expression of knowledge. This school of thought believes that learning is a process of reverse deduction, through existing
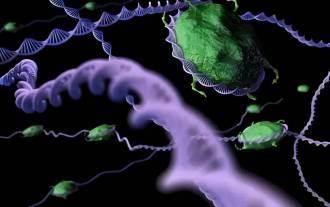
Editor | KX In the field of drug research and development, accurately and effectively predicting the binding affinity of proteins and ligands is crucial for drug screening and optimization. However, current studies do not take into account the important role of molecular surface information in protein-ligand interactions. Based on this, researchers from Xiamen University proposed a novel multi-modal feature extraction (MFE) framework, which for the first time combines information on protein surface, 3D structure and sequence, and uses a cross-attention mechanism to compare different modalities. feature alignment. Experimental results demonstrate that this method achieves state-of-the-art performance in predicting protein-ligand binding affinities. Furthermore, ablation studies demonstrate the effectiveness and necessity of protein surface information and multimodal feature alignment within this framework. Related research begins with "S
