Recently, Transformer-based algorithms have been widely used in various computer vision tasks. However, this type of algorithm is prone to over-fitting problems when the amount of training data is small. Existing Vision Transformers usually directly introduce the dropout algorithm commonly used in CNN as a regularizer, which performs random drops on the attention weight map and sets a unified drop probability for the attention layers of different depths. Although Dropout is very simple, there are three main problems with this drop method. First of all, random Drop after softmax normalization will break the probability distribution of attention weights and fail to punish weight peaks, resulting in the model still being overfitted. for local specific information (Figure 1). Secondly, a larger drop probability in the deeper layers of the network will lead to a lack of high-level semantic information, while a smaller drop probability in the shallower layers will lead to overfitting to the underlying detailed features, so a constant drop probability will lead to instability in the training process. Finally, the effectiveness of the structured drop method commonly used in CNN on Vision Transformer is not clear. 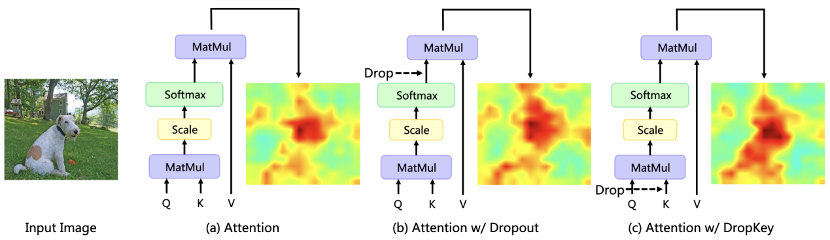
Figure 1 The impact of different regularizers on the attention distribution map Meitu Imaging Research Institute (MT Lab) and the University of Chinese Academy of Sciences published an article at CVPR 2023, proposing a novel and plug-and-play regularizer DropKey. It can effectively alleviate the over-fitting problem in Vision Transformer. 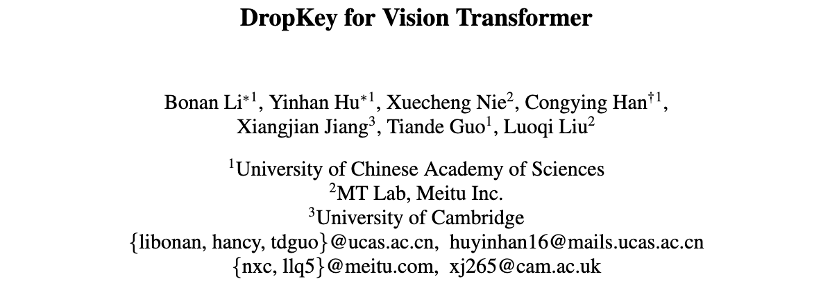
Paper link: https://arxiv.org/abs/2208.02646The following three core issues are discussed in the article Researched: #First, what information should be dropped in the attention layer? Different from directly dropping the attention weight, this method performs the Drop operation before calculating the attention matrix and uses the Key as the base Drop unit. This method theoretically verifies that the regularizer DropKey can penalize high-attention areas and allocate attention weights to other areas of interest, thereby enhancing the model's ability to capture global information. Second, how to set the Drop probability? Compared with all layers sharing the same Drop probability, this paper proposes a novel Drop probability setting method, which gradually attenuates the Drop probability value as the self-attention layer deepens. Third, is it necessary to perform a structured Drop operation like CNN? This method tried a structured drop approach based on block windows and cross windows, and found that this technique was not important for the Vision Transformer. ##Vision Transformer (ViT) is a new technology in recent computer vision models. Paradigm, it is widely used in tasks such as image recognition, image segmentation, human body key point detection and mutual detection of people. Specifically, ViT divides the picture into a fixed number of image blocks, treats each image block as a basic unit, and introduces a multi-head self-attention mechanism to extract feature information containing mutual relationships. However, existing ViT-like methods often suffer from overfitting problems on small data sets, that is, they only use local features of the target to complete specified tasks.
In order to overcome the above problems, this paper proposes a plug-and-play regularizer DropKey that can be implemented with only two lines of code to alleviate the ViT class method The overfitting problem. Different from the existing Dropout, DropKey sets the Key to the drop object and has theoretically and experimentally verified that this change can punish parts with high attention values while encouraging the model to pay more attention to other image patches related to the target, which is helpful. to capture global robust features. In addition, the paper also proposes to set decreasing drop probabilities for ever-deepening attention layers, which can avoid the model from overfitting low-level features while ensuring sufficient high-level features for stable training. In addition, the paper experimentally proves that the structured drop method is not necessary for ViT.
In order to explore the essential causes of over-fitting problems, this study First, the attention mechanism is formalized as a simple optimization objective and its Lagrangian expansion form is analyzed. It was found that when the model is continuously optimized, image patches with a larger proportion of attention in the current iteration will tend to be assigned a larger attention weight in the next iteration. To alleviate this problem, DropKey implicitly assigns an adaptive operator to each attention block by randomly dropping part of the Key to constrain the attention distribution and make it smoother. It is worth noting that compared to other regularizers designed for specific tasks, DropKey does not require any manual design. Since random drops are performed on Key during the training phase, which will lead to inconsistent output expectations in the training and testing phases, this method also proposes to use Monte Carlo methods or fine-tuning techniques to align output expectations. Furthermore, the implementation of this method requires only two lines of code, as shown in Figure 2.
Figure 2 DropKey implementation method Generally speaking, ViT will superimpose multiple attention layers to gradually learn high-dimensional features. Typically, shallower layers extract low-dimensional visual features, while deep layers aim to extract coarse but complex information on the modeling space. Therefore, this study attempts to set a smaller drop probability for deep layers to avoid losing important information of the target object. Specifically, DropKey does not perform random drops with a fixed probability at each layer, but gradually reduces the probability of drops as the number of layers increases. Additionally, the study found that this approach not only works with DropKey but also significantly improves Dropout performance. Although the structured drop method has been studied in detail in CNN, the performance impact of this drop method on ViT has not been studied. To explore whether this strategy will further improve performance, the paper implements two structured forms of DropKey, namely DropKey-Block and DropKey-Cross. Among them, DropKey-Block drops the continuous area in the square window centered on the seed point, and DropKey-Cross drops the cross-shaped continuous area centered on the seed point, as shown in Figure 3. However, the study found that the structured drop approach did not lead to performance improvements.
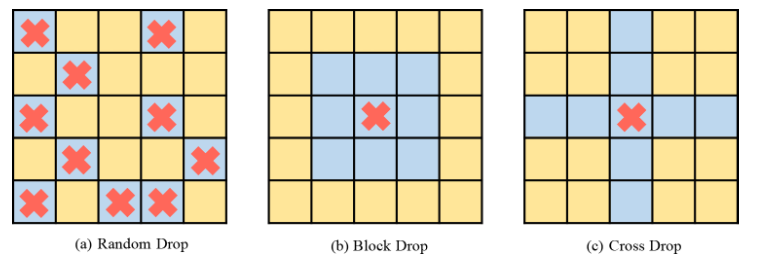
Figure 3 Structured implementation method of DropKey 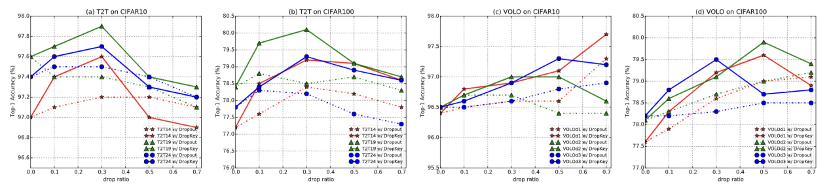
##Figure 4 Performance of DropKey and Dropout on CIFAR10/100 Comparison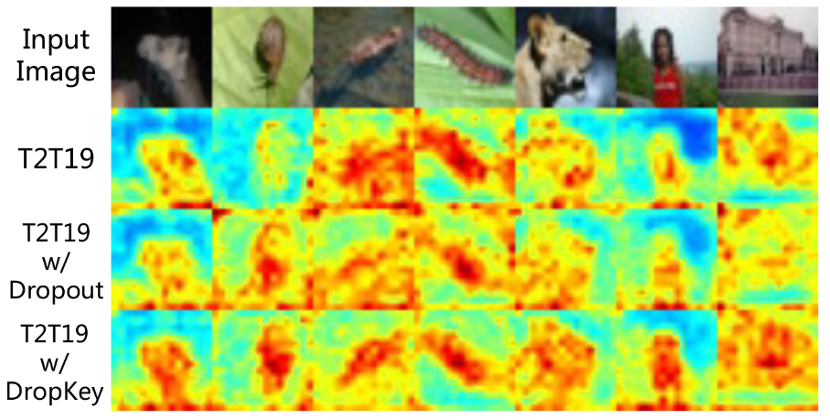
##Figure 5 Comparison of attention map visualization effects of DropKey and Dropout on CIFAR100
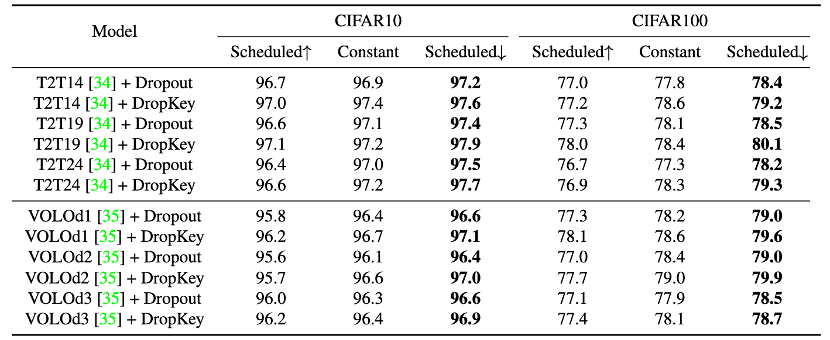
Figure 6 Performance comparison of different drop probability setting strategies
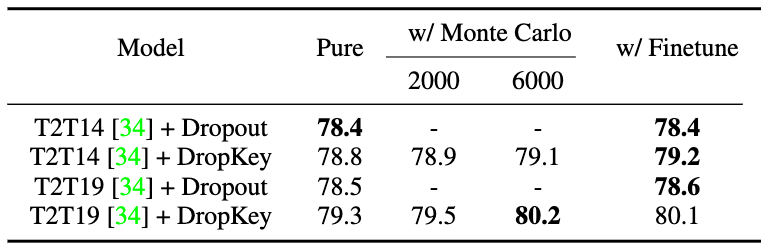
Figure 7 Performance comparison of different output expectation alignment strategies
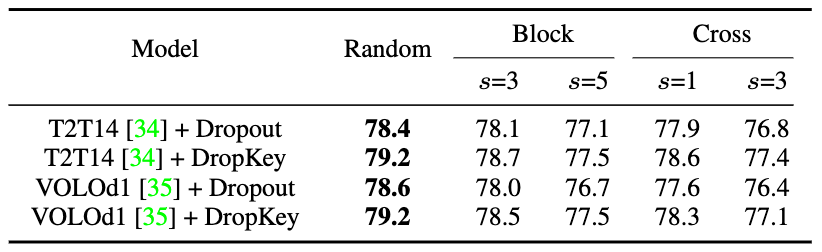
Figure 8 Performance comparison of different structured drop methods
##Figure 9 Performance comparison of DropKey and Dropout on ImageNet
Figure 10 Performance comparison of DropKey and Dropout on COCO

Figure 11 Performance comparison of DropKey and Dropout on HICO-DET
##Figure 12 Performance comparison of DropKey and Dropout on HICO-DET
Figure 13 Visual comparison of attention maps between DropKey and Dropout on HICO-DET#This paper innovatively proposes a regularizer for ViT to alleviate the over-fitting problem of ViT. Compared with existing regularizers, this method can provide smooth attention distribution for the attention layer by simply setting Key as a drop object. In addition, the paper also proposes a novel drop probability setting strategy, which successfully stabilizes the training process while effectively alleviating overfitting. Finally, the paper also explores the impact of structured drop methods on model performance. The above is the detailed content of CVPR 2023|Meitu & National University of Science and Technology jointly proposed the DropKey regularization method: using two lines of code to effectively avoid the visual Transformer overfitting problem. For more information, please follow other related articles on the PHP Chinese website!