


ReSimAD: How to improve the generalization performance of perceptual models through virtual data
Written before & the author’s personal understanding
Domain changes at the sensor level of autonomous vehicles are a very common phenomenon. For example, autonomous vehicles in different scenarios and locations are in Self-driving vehicles under different lighting and weather conditions, and self-driving vehicles equipped with different sensor equipment, all of the above can be considered as classic differences in the autonomous driving domain. This domain difference creates challenges for autonomous driving, mainly because autonomous driving models that rely on old domain knowledge are difficult to directly deploy to a new domain that has never been seen before without additional cost. Therefore, in this paper, we propose a reconstruction-simulation-awareness (ReSimAD) scheme to provide a new perspective and method for domain migration. Specifically, we use implicit reconstruction technology to obtain old domain knowledge in the driving scene. The purpose of the reconstruction process is to study how to convert domain-related knowledge in the old domain into domain-invariant representations (Domain-invariant Representations). For example, we believe that 3D scene-level mesh representations (3D Mesh Representations) are a domain-invariant representation. Based on the reconstructed results, we further use the simulator to generate a more realistic simulation point cloud of the target domain. This step relies on the reconstructed background information and the sensor solution of the target domain, thus reducing the collection and labeling time in the subsequent sensing process. Cost of new domain data.
We considered different cross-domain settings in the experimental verification part, including Waymo-to-KITTI, Waymo-to-nuScenes, Waymo-to-ONCE, etc. All cross-domain settings adopt zero-shot experimental settings, relying only on the background mesh and simulated sensors of the source domain to simulate target domain samples to improve model generalization capabilities. The results show that ReSimAD can greatly improve the generalization ability of the perception model to the target domain scene, even better than some unsupervised domain adaptation methods.
Paper information
- Paper title: ReSimAD: Zero-Shot 3D Domain Transfer for Autonomous Driving with Source Reconstruction and Target Simulation
- ICLR-2024 accepts
- Papers published by: Shanghai Artificial Intelligence Laboratory, Shanghai Jiao Tong University, Fudan University, Beihang University
- Paper address: https: //arxiv.org/abs/2309.05527
- Code address: simulation data set and perception part, https://github.com/PJLab-ADG/3DTrans#resimad; source domain reconstruction part, https:// github.com/pjlab-ADG/neuralsim; Target domain simulation part, https://github.com/PJLab-ADG/PCSim
Research motivation
Challenge: Although 3D models can help autonomous vehicles recognize their surroundings, existing baseline models are difficult to generalize to new domains (such as different sensor settings or unseen city of). The long-term vision in the field of autonomous driving is to enable models to achieve domain migration at a lower cost, that is, to successfully adapt a model fully trained on the source domain to the target domain scenario, where the source domain and target domain exist respectively. There are two domains with obvious data distribution differences. For example, the source domain is sunny and the target domain is rainy; the source domain is a 64-beam sensor and the target domain is a 32-beam sensor.
Commonly used solution ideas: Faced with the above domain differences, the most common solution is to obtain and annotate data for the target domain scenario. This method can avoid domain differences to a certain extent. The problem of model performance degradation caused by the difference, but there is a huge 1) data collection cost and 2) data labeling cost. Therefore, as shown in the figure below (see the two baseline methods (a) and (b)), in order to alleviate the cost of data collection and data annotation for a new domain, the simulation engine can be used to render some simulated point cloud samples. This is common Solution ideas for sim-to-real research work. Another idea is unsupervised domain adaptation (UDA for 3D). The purpose of this type of work is to study how to achieve approximately fully supervised fine-tuning under the condition of only being exposed to unlabeled target domain data (note that it is real data) If this can be achieved, it will indeed save the cost of labeling the target domain. However, the UDA method still needs to collect a large amount of real target domain data to characterize the data distribution of the target domain.
Figure 1: Comparison of different training paradigms
Our ideas: Different from the above two categories of research ideas, as shown in the figure below (please see (c) baseline process), we are committed to the data simulation-perception integration route that combines virtual and real , where reality in the combination of virtual and real refers to constructing a domain-invariant representation based on massive labeled source domain data. This assumption has practical significance for many scenarios, because after long-term historical data accumulation, We can always think that this kind of labeled source domain data exists; on the other hand, simulation in the combination of virtual and real means: when we build a domain-invariant representation based on the source domain data, This representation can be imported into an existing rendering pipeline to perform simulation of target domain data. Compared with current sim-to-real research work, our method is supported by real scene-level data, including real information such as road structure, uphill and downhill slopes, etc. This information is difficult to obtain solely by relying on the simulation engine itself. After obtaining data in the target domain, we integrate the data into the best current perception model, such as PV-RCNN, for training, and then verify the accuracy of the model in the target domain. Please see the figure below for the overall detailed workflow:
Figure 2 ReSimAD flow chart
The flow chart of ReSimAD is shown in Figure 2, which mainly includesa) Point-to -Mesh implicit reconstruction process, b) Mesh-to-point simulation engine rendering process, c) Zero-sample sensing process.
ReSimAD: Simulation reconstruction-aware paradigm
a) Point-to-mesh implicit reconstruction process: affected by StreetSurf Inspired by , we only use lidar reconstruction to reconstruct real and diverse street scene backgrounds and dynamic traffic flow information. We first designed a pure point cloud SDF reconstruction module (LiDAR-only Implicit Neural Reconstruction, LINR). Its advantage is that it is not affected by some domain differences caused by camera sensing, such as: changes in illumination, changes in weather conditions, etc. . The pure point cloud SDF reconstruction module takes LiDAR rays as input, then predicts depth information, and finally builds a 3D meshes representation of the scene.
Specifically, for the ray emitted from the origin
with direction
, we apply volume rendering to the lidar to train the Signed Distance Field (SDF) network, and the rendering depth D can be formulated is:
where is the sampling depth of the sample point , is the accumulated transmittance (the accumulated transmittance), which is obtained by using the close range model in NeuS of.
Taking inspiration from StreetSurf, the model input for the reconstruction process proposed in this article comes from lidar rays, and the output is the predicted depth. On each sampled lidar beam , we apply a logarithmic L1 loss on
, which is the combined rendering depth of the near and distant models:
However, LINR methods still face some challenges. Due to the inherent sparsity of data acquired by lidar, a single lidar point cloud frame can only capture a portion of the information contained in a standard RGB image. This difference highlights the potential shortcomings of depth rendering in providing the necessary geometric detail for effective training. Therefore, this can lead to a large number of artifacts within the resulting reconstructed mesh. To address this challenge, we propose to splice all frames in a Waymo sequence to increase the density of the point cloud.
Due to the limitation of the vertical field of view of the Top LiDAR in the Waymo dataset, only obtaining point clouds between -17.6° and 2.4° has obvious limitations on the reconstruction of surrounding high-rise buildings. To address this challenge, we introduce a solution that incorporates point clouds from Side LiDAR into sampling sequences for reconstruction. Four blind-filling radars are installed on the front, rear and two sides of the autonomous vehicle, with a vertical field of view reaching [-90°, 30°], which effectively compensates for the shortcomings of the insufficient field of view range of the top lidar. Due to the difference in point cloud density between side lidar and top lidar, we choose to assign a higher sampling weight to side lidar to improve the reconstruction quality of high-rise building scenes.
Reconstruction quality evaluation: Due to the occlusion caused by dynamic objects and the impact of lidar noise, there may be a certain amount of noise in the implicit representation for reconstruction. Therefore, we evaluated the reconstruction accuracy. Because we can obtain massive annotated point cloud data from the old domain, we can obtain the simulated point cloud data of the old domain by re-rendering on the old domain to evaluate the accuracy of the reconstructed mesh. We measure the simulated point cloud and the original real point cloud using root mean square error (RMSE) and chamfer distance (CD):
where for each For a description of the sequence reconstruction scores and some detailed processes, please refer to the original appendix.
b) Mesh-to-point simulation engine rendering process: After obtaining the static background mesh through the above LINR method, we use the Blender Python API to convert the mesh into The data is converted from .ply format to 3D model files in .fbx format, and the background mesh is finally loaded as an asset library into the open source simulator CARLA.
We first obtain Waymo's annotation file to obtain the bounding box category and three-dimensional object size of each traffic participant, and based on this information, we search for traffic participants of the same category in CARLA's digital asset library The closest digital asset in size is imported and used as a traffic participant model. Based on the scene authenticity information available in the CARLA simulator, we developed a detection box extraction tool for each detectable object in the traffic scene. For details, please refer to PCSim Development Tools.
Figure 3 Distribution of object sizes (length, width, height) of traffic participants in different data sets. As can be seen from Figure 3, the distribution diversity of object sizes simulated using this method is very wide, exceeding currently published data sets such as KITTI, nuScenes, Waymo, ONCE, etc.
We use Waymo as the source domain data and reconstruct it on Waymo to obtain a more realistic 3D mesh. At the same time, we use KITTI, nuScenes, and ONCE as target domain scenarios, and verify the zero-shot performance achieved by our method in these target domain scenarios.
We generate 3D scene-level mesh data based on the Waymo data set according to the introduction in the above chapter, and use the above evaluation criteria to determine which 3D meshes are of high quality under the Waymo domain, and select the highest 146 based on the score. meshes to carry out the subsequent target domain simulation process.
Evaluation results
Some visualization examples in the ResimAD data set are shown below:
Evaluation resultsExperimental Settings
Baseline Selection: We compare the proposed ReSimAD with three typical cross-domain baselines: a) Baseline for data simulation using the simulation engine directly; b) Baseline for data simulation by changing sensor parameter settings in the simulation engine; c) Domain Adaptation (UDA) baseline.
- Metric: We Align The current evaluation standards for 3D cross-domain object detection use BEV-based and 3D-based AP as evaluation metrics respectively.
- Parameter settings: For details, please refer to the paper.
Only the main experimental results are shown here, please refer to us for more results thesis.
Adaptation performance of PV-RCNN/PV-RCNN two models under three cross-domain settings
We can observe from the above table: The main difference between UDA and ReSimAD using unsupervised domain adaptation (UDA) technology is that the former uses samples of the target domain real scenes for model domain migration , and the experimental setting of ReSimAD requires that it cannot access any real point cloud data in the target domain. As can be seen from the above table, the cross-domain results obtained by our ReSimAD are comparable to those obtained by the UDA method. This result shows that when the lidar sensor needs to be upgraded for commercial purposes, our method can greatly reduce the cost of data collection and further shorten the retraining and redevelopment cycle of the model due to domain differences.
ReSimAD data is used as the cold start data of the target domain, and the effect that can be achieved on the target domain
Data generated using ReSimAD Another benefit is that high-performance target domain accuracy can be obtained without accessing any real data distribution of the target domain. This process is actually similar to the "cold start" process of the autonomous driving model in new scenarios.
The above table reports the experimental results under the fully supervised target domain. Oracle represents the result of the model being trained on the full amount of labeled target domain data, while SFT represents that the network initialization parameters of the baseline model are provided by the weights trained on the ReSimAD simulation data. The above experimental table shows that the point cloud simulated using our ReSimAD method can obtain higher initialization weight parameters, and its performance exceeds the Oracle experimental setting.
ReSimAD data is used as a general data set, using the performance of AD-PT pre-training method on different downstream tasks
For verification Whether ReSimAD can generate more point cloud data to help 3D pre-training, we designed the following experiments: use AD-PT (a recently proposed method for pre-training backbone networks in autonomous driving scenarios) to pre-train on simulated point clouds Train the 3D backbone and then use downstream real scene data for full parameter fine-tuning.
- We leverage ReSimAD to generate data with a wider distribution of point clouds. In order to make a fair comparison with the pre-training results in AD-PT, the target amount of simulated point cloud data generated by ReSimAD is approximately . In the above table, our baseline detectors are 3D pre-trained on real pre-training data (ONCE dataset) and simulated pre-training data (provided by ReSimAD), using the AD-PT method, and on the KITTI and Waymo datasets Perform downstream fine-tuning. The results in the above table show that using simulation pre-training data of different sizes can continuously improve the performance of the model in the downstream. In addition, it can be seen that the data acquisition cost of pre-training data obtained by ReSimAD is very low, compared with using ONCE for model pre-training, and the pre-training performance obtained by ReSimAD is comparable to the pre-training performance on the ONCE data set. Comparison of.
Visual comparison using ReSimAD to reconstruct simulation vs. using CARLA default simulation
We are based on the Waymo data set Visual comparison of reconstructed mesh vs. reconstruction using VDBFusion
Summary
In this work, we are committed to Study how to experiment with the zero-sample target domain model transfer task. This task requires the model to successfully migrate the pre-trained model in the source domain to the target domain scene without being exposed to any sample data information from the target domain. Different from previous work, we explored for the first time a 3D data generation technology based on implicit reconstruction of the source domain and diversity simulation of the target domain, and verified that this technology can achieve a better model without being exposed to the data distribution of the target domain. The migration performance is even better than some unsupervised domain adaptation (UDA) methods.
Original link: https://mp.weixin.qq.com/s/pmHFDvS7nXy-6AQBhvVzSw
The above is the detailed content of ReSimAD: How to improve the generalization performance of perceptual models through virtual data. For more information, please follow other related articles on the PHP Chinese website!

Hot AI Tools

Undresser.AI Undress
AI-powered app for creating realistic nude photos

AI Clothes Remover
Online AI tool for removing clothes from photos.

Undress AI Tool
Undress images for free

Clothoff.io
AI clothes remover

Video Face Swap
Swap faces in any video effortlessly with our completely free AI face swap tool!

Hot Article

Hot Tools

Notepad++7.3.1
Easy-to-use and free code editor

SublimeText3 Chinese version
Chinese version, very easy to use

Zend Studio 13.0.1
Powerful PHP integrated development environment

Dreamweaver CS6
Visual web development tools

SublimeText3 Mac version
God-level code editing software (SublimeText3)

Hot Topics


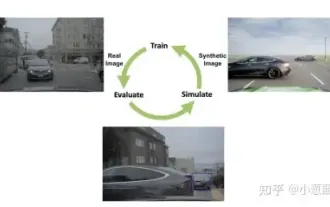
Yesterday during the interview, I was asked whether I had done any long-tail related questions, so I thought I would give a brief summary. The long-tail problem of autonomous driving refers to edge cases in autonomous vehicles, that is, possible scenarios with a low probability of occurrence. The perceived long-tail problem is one of the main reasons currently limiting the operational design domain of single-vehicle intelligent autonomous vehicles. The underlying architecture and most technical issues of autonomous driving have been solved, and the remaining 5% of long-tail problems have gradually become the key to restricting the development of autonomous driving. These problems include a variety of fragmented scenarios, extreme situations, and unpredictable human behavior. The "long tail" of edge scenarios in autonomous driving refers to edge cases in autonomous vehicles (AVs). Edge cases are possible scenarios with a low probability of occurrence. these rare events
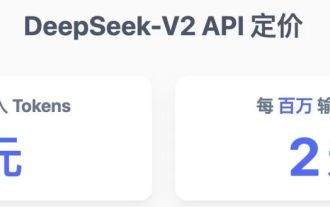
Imagine an artificial intelligence model that not only has the ability to surpass traditional computing, but also achieves more efficient performance at a lower cost. This is not science fiction, DeepSeek-V2[1], the world’s most powerful open source MoE model is here. DeepSeek-V2 is a powerful mixture of experts (MoE) language model with the characteristics of economical training and efficient inference. It consists of 236B parameters, 21B of which are used to activate each marker. Compared with DeepSeek67B, DeepSeek-V2 has stronger performance, while saving 42.5% of training costs, reducing KV cache by 93.3%, and increasing the maximum generation throughput to 5.76 times. DeepSeek is a company exploring general artificial intelligence
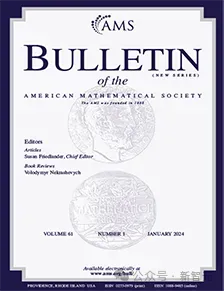
AI is indeed changing mathematics. Recently, Tao Zhexuan, who has been paying close attention to this issue, forwarded the latest issue of "Bulletin of the American Mathematical Society" (Bulletin of the American Mathematical Society). Focusing on the topic "Will machines change mathematics?", many mathematicians expressed their opinions. The whole process was full of sparks, hardcore and exciting. The author has a strong lineup, including Fields Medal winner Akshay Venkatesh, Chinese mathematician Zheng Lejun, NYU computer scientist Ernest Davis and many other well-known scholars in the industry. The world of AI has changed dramatically. You know, many of these articles were submitted a year ago.
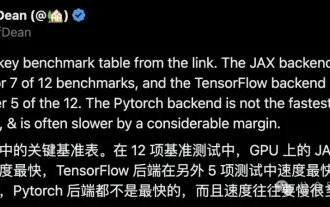
The performance of JAX, promoted by Google, has surpassed that of Pytorch and TensorFlow in recent benchmark tests, ranking first in 7 indicators. And the test was not done on the TPU with the best JAX performance. Although among developers, Pytorch is still more popular than Tensorflow. But in the future, perhaps more large models will be trained and run based on the JAX platform. Models Recently, the Keras team benchmarked three backends (TensorFlow, JAX, PyTorch) with the native PyTorch implementation and Keras2 with TensorFlow. First, they select a set of mainstream
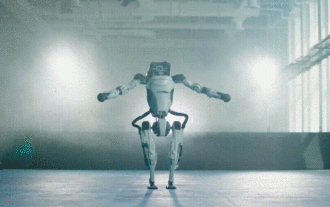
Boston Dynamics Atlas officially enters the era of electric robots! Yesterday, the hydraulic Atlas just "tearfully" withdrew from the stage of history. Today, Boston Dynamics announced that the electric Atlas is on the job. It seems that in the field of commercial humanoid robots, Boston Dynamics is determined to compete with Tesla. After the new video was released, it had already been viewed by more than one million people in just ten hours. The old people leave and new roles appear. This is a historical necessity. There is no doubt that this year is the explosive year of humanoid robots. Netizens commented: The advancement of robots has made this year's opening ceremony look like a human, and the degree of freedom is far greater than that of humans. But is this really not a horror movie? At the beginning of the video, Atlas is lying calmly on the ground, seemingly on his back. What follows is jaw-dropping
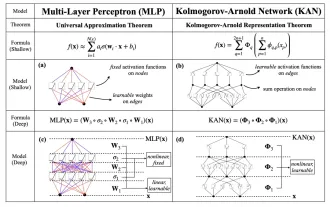
Earlier this month, researchers from MIT and other institutions proposed a very promising alternative to MLP - KAN. KAN outperforms MLP in terms of accuracy and interpretability. And it can outperform MLP running with a larger number of parameters with a very small number of parameters. For example, the authors stated that they used KAN to reproduce DeepMind's results with a smaller network and a higher degree of automation. Specifically, DeepMind's MLP has about 300,000 parameters, while KAN only has about 200 parameters. KAN has a strong mathematical foundation like MLP. MLP is based on the universal approximation theorem, while KAN is based on the Kolmogorov-Arnold representation theorem. As shown in the figure below, KAN has
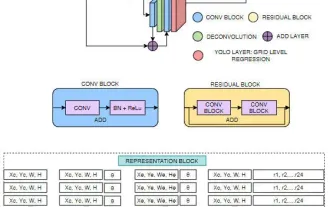
Target detection is a relatively mature problem in autonomous driving systems, among which pedestrian detection is one of the earliest algorithms to be deployed. Very comprehensive research has been carried out in most papers. However, distance perception using fisheye cameras for surround view is relatively less studied. Due to large radial distortion, standard bounding box representation is difficult to implement in fisheye cameras. To alleviate the above description, we explore extended bounding box, ellipse, and general polygon designs into polar/angular representations and define an instance segmentation mIOU metric to analyze these representations. The proposed model fisheyeDetNet with polygonal shape outperforms other models and simultaneously achieves 49.5% mAP on the Valeo fisheye camera dataset for autonomous driving
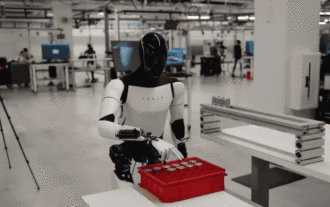
The latest video of Tesla's robot Optimus is released, and it can already work in the factory. At normal speed, it sorts batteries (Tesla's 4680 batteries) like this: The official also released what it looks like at 20x speed - on a small "workstation", picking and picking and picking: This time it is released One of the highlights of the video is that Optimus completes this work in the factory, completely autonomously, without human intervention throughout the process. And from the perspective of Optimus, it can also pick up and place the crooked battery, focusing on automatic error correction: Regarding Optimus's hand, NVIDIA scientist Jim Fan gave a high evaluation: Optimus's hand is the world's five-fingered robot. One of the most dexterous. Its hands are not only tactile
