


RoSA: A new method for efficient fine-tuning of large model parameters
As language models scale to unprecedented scale, comprehensive fine-tuning of downstream tasks becomes prohibitively expensive. In order to solve this problem, researchers began to pay attention to and adopt the PEFT method. The main idea of the PEFT method is to limit the scope of fine-tuning to a small set of parameters to reduce computational costs while still achieving state-of-the-art performance on natural language understanding tasks. In this way, researchers can save computing resources while maintaining high performance, bringing new research hotspots to the field of natural language processing.
RoSA is a new PEFT technology. Through experiments on a set of benchmark tests, it was found that when using the same parameter budget, RoSA performs better than previous low-rank adaptation (LoRA) and pure sparse fine-tuning methods.
This article will delve into RoSA principles, methods and results, explaining how its performance marks meaningful progress. For those who want to effectively fine-tune large language models, RoSA provides a new solution that is superior to previous solutions.
Demand for efficient fine-tuning of parameters
NLP has been replaced by transformer-based language models such as GPT- 4 Complete changes. These models learn powerful language representations by pre-training on large text corpora. They then transfer these representations to downstream language tasks through a simple process.
As model size grows from billions to trillions of parameters, fine-tuning brings a huge computational burden. For example, for a model like GPT-4 with 1.76 trillion parameters, fine-tuning can cost millions of dollars. This makes deployment in real applications very impractical.
PEFT method improves efficiency and accuracy by limiting the parameter range of fine-tuning. Recently, a variety of PEFT technologies have emerged that trade off efficiency and accuracy.
LoRA
#One prominent PEFT method is low-rank adaptation (LoRA). LoRA was launched in 2021 by researchers from Meta and MIT. This approach is motivated by their observation that the transformer exhibits low-rank structure in its head matrix. LoRA is proposed to take advantage of this low-rank structure to reduce computational complexity and improve model efficiency and speed.
LoRA only fine-tunes the first k singular vectors, while other parameters remain unchanged. This only requires O(k) extra parameters to tune, instead of O(n).
By leveraging this low-rank structure, LoRA can capture meaningful signals needed for generalization to downstream tasks and limit fine-tuning to these top singular vectors, enabling optimization and inference More effective.
Experiments show that LoRA can match fully fine-tuned performance on the GLUE benchmark while using more than 100 times fewer parameters. However, as the model size continues to expand, obtaining strong performance through LoRA requires increasing rank k, reducing the computational savings compared to full fine-tuning.
Before RoSA, LoRA represented the state-of-the-art in PEFT methods, with only modest improvements using techniques such as different matrix factorization or adding a small number of additional fine-tuning parameters.
Robust Adaptation (RoSA)
Robust Adaptation (RoSA) introduces a new parameter-efficient fine-tuning method. RoSA is inspired by robust principal component analysis (robust PCA), rather than relying solely on low-rank structures.
In traditional principal component analysis, the data matrix X is decomposed into matrix. Robust PCA goes a step further and decomposes X into a clean low-rank L and a "contaminated/corrupted" sparse S.
RoSA draws inspiration from this and decomposes the fine-tuning of the language model into:
A low-rank adaptive (L) matrix similar to LoRA , fine-tuned to approximate the dominant task-related signal
A highly sparse fine-tuning (S) matrix containing a very small number of large, selectively fine-tuned parameters that encode L miss the residual signal.
Explicitly modeling the residual sparse component allows RoSA to achieve higher accuracy than LoRA alone.
RoSA constructs L by performing a low-rank decomposition of the model’s head matrix. This will encode underlying semantic representations useful for downstream tasks. RoSA then selectively fine-tunes the top m most important parameters of each layer to S, while all other parameters remain unchanged. This step captures residual signals that are not suitable for low-rank fitting.
The number of fine-tuning parameters m is an order of magnitude smaller than the rank k required by LoRA alone. Therefore, combined with the low-rank head matrix in L, RoSA maintains extremely high parameter efficiency.
RoSA also uses some other simple but effective optimizations:
Residual sparse connection: S residuals are added directly to the output of each transformer block before it goes through layer normalization and feedforward sublayers. This can simulate signals missed by L.
Independent Sparse Mask: The metrics selected in S for fine-tuning are generated independently for each transformer layer.
Shared low-rank structure: The same low-rank base U,V matrices are shared between all layers of L, just like in LoRA. This will capture semantic concepts in a consistent subspace.
These architectural choices provide RoSA modeling with the flexibility akin to full fine-tuning while maintaining parameter efficiency for optimization and inference. Utilizing this PEFT method that combines robust low-rank adaptation and highly sparse residuals, RoSA achieves a new technology of accuracy-efficiency trade-off.
Experiments and Results
The researchers evaluated RoSA on a comprehensive benchmark of 12 NLU datasets covering Tasks such as text detection, sentiment analysis, natural language reasoning and robustness testing. They conducted experiments using RoSA based on artificial intelligence assistant LLM, using a 12 billion parameter model.
On every task, RoSA performs significantly better than LoRA when using the same parameters. The total parameters of both methods are approximately 0.3% of the entire model. This means that there are about 4.5 million fine-tuning parameters in both cases for k = 16 for LoRA and m = 5120 for RoSA.
RoSA also matches or exceeds the performance of pure sparse fine-tuned baselines.
On the ANLI benchmark, which evaluates robustness to adversarial examples, RoSA scores 55.6, while LoRA scores 52.7. This demonstrates improvements in generalization and calibration.
For the sentiment analysis tasks SST-2 and IMDB, the accuracy of RoSA reaches 91.2% and 96.9%, while the accuracy of LoRA reaches 90.1% and 95.3%.
On WIC, a challenging word sense disambiguation test, RoSA has an F1 score of 93.5, while LoRA has an F1 score of 91.7.
Across all 12 datasets, RoSA generally shows better performance than LoRA under matching parameter budgets.
Notably, RoSA is able to achieve these gains without requiring any task-specific tuning or specialization. This makes RoSA suitable for use as a universal PEFT solution.
Summary
As the size of language models continues to grow rapidly, reducing the computational requirements for fine-tuning them is an urgent problem that needs to be solved. Parameter-efficient adaptive training techniques like LoRA have shown initial success but face inherent limitations of low-rank approximation.
RoSA organically combines robust low-rank decomposition and highly sparse residual fine-tuning to provide a convincing new solution. It greatly improves the performance of PEFT by considering signals that escape low-rank fitting through selective sparse residuals. Empirical evaluation shows significant improvements over LoRA and uncontrolled sparsity baselines on different NLU task sets.
RoSA is conceptually simple but highly performant, and can further advance cross-research on parameter efficiency, adaptive representation, and continuous learning to expand language intelligence.
The above is the detailed content of RoSA: A new method for efficient fine-tuning of large model parameters. For more information, please follow other related articles on the PHP Chinese website!

Hot AI Tools

Undresser.AI Undress
AI-powered app for creating realistic nude photos

AI Clothes Remover
Online AI tool for removing clothes from photos.

Undress AI Tool
Undress images for free

Clothoff.io
AI clothes remover

AI Hentai Generator
Generate AI Hentai for free.

Hot Article

Hot Tools

Notepad++7.3.1
Easy-to-use and free code editor

SublimeText3 Chinese version
Chinese version, very easy to use

Zend Studio 13.0.1
Powerful PHP integrated development environment

Dreamweaver CS6
Visual web development tools

SublimeText3 Mac version
God-level code editing software (SublimeText3)

Hot Topics


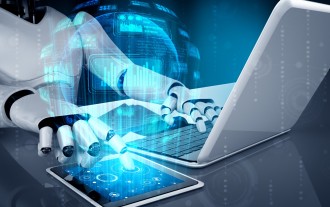
If you have been paying attention to the architecture of large language models, you may have seen the term "SwiGLU" in the latest models and research papers. SwiGLU can be said to be the most commonly used activation function in large language models. We will introduce it in detail in this article. SwiGLU is actually an activation function proposed by Google in 2020, which combines the characteristics of SWISH and GLU. The full Chinese name of SwiGLU is "bidirectional gated linear unit". It optimizes and combines two activation functions, SWISH and GLU, to improve the nonlinear expression ability of the model. SWISH is a very common activation function that is widely used in large language models, while GLU has shown good performance in natural language processing tasks.
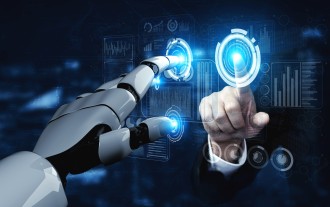
Large Language Models (LLMs) are trained on huge text databases, where they acquire large amounts of real-world knowledge. This knowledge is embedded into their parameters and can then be used when needed. The knowledge of these models is "reified" at the end of training. At the end of pre-training, the model actually stops learning. Align or fine-tune the model to learn how to leverage this knowledge and respond more naturally to user questions. But sometimes model knowledge is not enough, and although the model can access external content through RAG, it is considered beneficial to adapt the model to new domains through fine-tuning. This fine-tuning is performed using input from human annotators or other LLM creations, where the model encounters additional real-world knowledge and integrates it
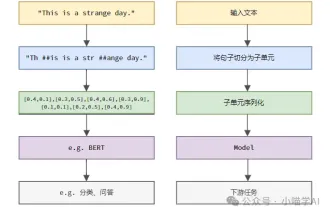
Language models reason about text, which is usually in the form of strings, but the input to the model can only be numbers, so the text needs to be converted into numerical form. Tokenization is a basic task of natural language processing. It can divide a continuous text sequence (such as sentences, paragraphs, etc.) into a character sequence (such as words, phrases, characters, punctuation, etc.) according to specific needs. The units in it Called a token or word. According to the specific process shown in the figure below, the text sentences are first divided into units, then the single elements are digitized (mapped into vectors), then these vectors are input to the model for encoding, and finally output to downstream tasks to further obtain the final result. Text segmentation can be divided into Toke according to the granularity of text segmentation.
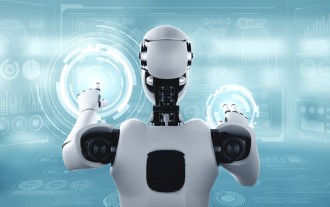
As the performance of open source large-scale language models continues to improve, performance in writing and analyzing code, recommendations, text summarization, and question-answering (QA) pairs has all improved. But when it comes to QA, LLM often falls short on issues related to untrained data, and many internal documents are kept within the company to ensure compliance, trade secrets, or privacy. When these documents are queried, LLM can hallucinate and produce irrelevant, fabricated, or inconsistent content. One possible technique to handle this challenge is Retrieval Augmented Generation (RAG). It involves the process of enhancing responses by referencing authoritative knowledge bases beyond the training data source to improve the quality and accuracy of the generation. The RAG system includes a retrieval system for retrieving relevant document fragments from the corpus
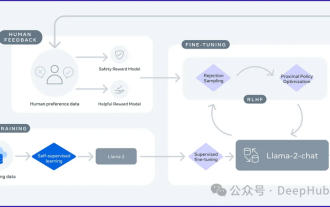
2024 is a year of rapid development for large language models (LLM). In the training of LLM, alignment methods are an important technical means, including supervised fine-tuning (SFT) and reinforcement learning with human feedback that relies on human preferences (RLHF). These methods have played a crucial role in the development of LLM, but alignment methods require a large amount of manually annotated data. Faced with this challenge, fine-tuning has become a vibrant area of research, with researchers actively working to develop methods that can effectively exploit human data. Therefore, the development of alignment methods will promote further breakthroughs in LLM technology. The University of California recently conducted a study introducing a new technology called SPIN (SelfPlayfInetuNing). S
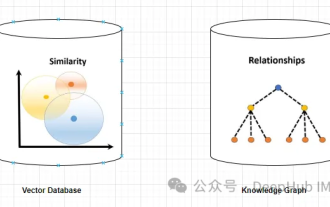
Hallucinations are a common problem when working with large language models (LLMs). Although LLM can generate smooth and coherent text, the information it generates is often inaccurate or inconsistent. In order to prevent LLM from hallucinations, external knowledge sources, such as databases or knowledge graphs, can be used to provide factual information. In this way, LLM can rely on these reliable data sources, resulting in more accurate and reliable text content. Vector Database and Knowledge Graph Vector Database A vector database is a set of high-dimensional vectors that represent entities or concepts. They can be used to measure the similarity or correlation between different entities or concepts, calculated through their vector representations. A vector database can tell you, based on vector distance, that "Paris" and "France" are closer than "Paris" and
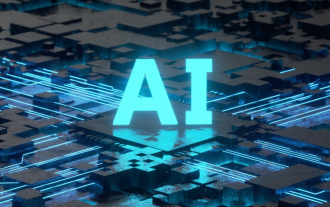
Editor |ScienceAI Question Answering (QA) data set plays a vital role in promoting natural language processing (NLP) research. High-quality QA data sets can not only be used to fine-tune models, but also effectively evaluate the capabilities of large language models (LLM), especially the ability to understand and reason about scientific knowledge. Although there are currently many scientific QA data sets covering medicine, chemistry, biology and other fields, these data sets still have some shortcomings. First, the data form is relatively simple, most of which are multiple-choice questions. They are easy to evaluate, but limit the model's answer selection range and cannot fully test the model's ability to answer scientific questions. In contrast, open-ended Q&A
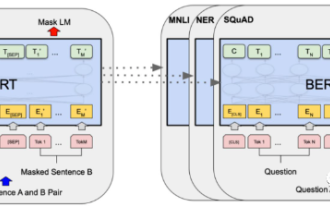
In 2018, Google released BERT. Once it was released, it defeated the State-of-the-art (Sota) results of 11 NLP tasks in one fell swoop, becoming a new milestone in the NLP world. The structure of BERT is shown in the figure below. On the left is the BERT model preset. The training process, on the right is the fine-tuning process for specific tasks. Among them, the fine-tuning stage is for fine-tuning when it is subsequently used in some downstream tasks, such as text classification, part-of-speech tagging, question and answer systems, etc. BERT can be fine-tuned on different tasks without adjusting the structure. Through the task design of "pre-trained language model + downstream task fine-tuning", it brings powerful model effects. Since then, "pre-training language model + downstream task fine-tuning" has become the mainstream training in the NLP field.
