


Decrypting the numpy library: revealing the algorithm principles and working mechanisms behind it
Decrypt the numpy library: Reveal the algorithm principles and working mechanisms behind it
With the rapid development of technology, data science has become an extremely important field. Among them, data processing and analysis are the core links in data science. Moreover, as the amount of data becomes larger and larger, the data processing speed has become an issue that cannot be ignored.
In the field of data science, Python is one of the most commonly used programming languages. As one of the most important data processing libraries in Python, the numpy library is widely used in data science.
This article will focus on the numpy library and reveal the algorithm principles and working mechanisms behind it. At the same time, specific code examples help readers gain a deeper understanding of the usage and application scenarios of numpy.
1. Introduction to numpy
The full name of numpy is Numerical Python, which is a mathematical calculation library based on the Python language. Numpy provides a high-performance, multi-dimensional array data structure, and provides a large number of mathematical functions based on it, which can be used to perform a variety of scientific calculations.
numpy was originally developed by Jim Hugunin, and its core is written in C language. Therefore, numpy not only has the ease of use of Python's high-level programming language, but also has the efficiency of C language.
2. Numpy array
The array in numpy, also called ndarray, is a multi-dimensional array data structure. In numpy, ndarray objects can be one-dimensional or multi-dimensional. Numpy arrays have the following characteristics:
1. Same type: The elements in ndarray must be of the same type.
2. Fixed size: The size of the ndarray object is fixed, that is, when the array is created and the array size is defined, the array size cannot be changed.
3. Support vectorization operations: The vectorization operation in numpy can perform an operation on the entire array without the need to perform the same operation for each element in the array through a loop.
4. Efficiency: Since the bottom layer of numpy is written in C language, its processing efficiency is very high.
The following are some common operations on numpy arrays:
- Creating arrays
Using numpy, you can create arrays through the np.array() function . The np.array() function can receive a Python list or tuple as input and return an ndarray object.
Sample code:
import numpy as np arr = np.array([1, 2, 3]) print(arr)
Output result:
[1 2 3]
- The shape and size of the array
The shape attribute can be used in numpy Get the shape of the array, you can also use the ndarray.size property to get the number of elements in the array.
Sample code:
import numpy as np arr = np.array([[1, 2, 3], [4, 5, 6]]) print(arr.shape) print(arr.size)
Output result:
(2, 3) 6
- Array access
In numpy, arrays can be accessed through indexing elements in . For multidimensional arrays, you can use commas to separate indices.
Sample code:
import numpy as np arr = np.array([[1, 2, 3], [4, 5, 6]]) print(arr[0,1])
Output result:
2
3. Algorithm principle and working mechanism in numpy
The core algorithm and mechanism of numpy library are divided into It is divided into two parts: data structure and C language implementation. The data structure refers to the ndarray object in numpy, which is a multi-dimensional array implemented in C language. The core algorithm implemented in C language is the efficiency guarantee of numpy.
The C language implementation in numpy works in the Python interpreter. When the user calls a function in the numpy library, the Python interpreter will pass the data and function to the numpy library. In the numpy library, the C language code will pass the data structure ndarray to the corresponding algorithm and mathematics library.
Since many core functions in the numpy library are implemented in C language, the numpy library is much more efficient than pure Python code when processing large-scale data. This is because Python is an interpreted language and the code needs to be parsed and compiled during execution. The C language is a compiled language, so during the execution process, the C language code is directly converted into machine code, making it more efficient.
Another important reason why the numpy library relies on C language is that C language has a rich mathematical operation library and underlying hardware support. This allows calculations in the numpy library to be hardware accelerated and more efficient. The efficiency of the numpy library is one of the reasons why Python is used in the field of data science.
4. Application scenarios of numpy
The numpy library is widely used in the field of data science. The following are some common application scenarios of the numpy library in the field of data science:
- Mathematical calculation
The numpy library provides many mathematical functions that can be used to perform various Various scientific calculations, such as matrix multiplication, matrix addition, convolution and Fourier transform, etc.
- Data processing
The numpy library provides many functions for processing data, such as array sorting, filtering, deleting duplicate values, etc.
- Statistics and Modeling
The numpy library has many functions for statistical analysis and modeling, such as linear regression, normal distribution, etc.
- Data Visualization
Arrays in the numpy library can be used as input data for data visualization libraries such as matplotlib to draw graphics.
5. Summary
The numpy library is one of the most important data processing and analysis libraries in Python. It is implemented based on C language and provides efficient multi-dimensional array data structures and various mathematical, processing, statistical and modeling functions.
Through the introduction of this article, we can have a more comprehensive understanding of the algorithm principles and working mechanisms behind the numpy library. At the same time, we can also have a deeper understanding of the usage scenarios and application methods of the numpy library.
The above is the detailed content of Decrypting the numpy library: revealing the algorithm principles and working mechanisms behind it. For more information, please follow other related articles on the PHP Chinese website!

Hot AI Tools

Undresser.AI Undress
AI-powered app for creating realistic nude photos

AI Clothes Remover
Online AI tool for removing clothes from photos.

Undress AI Tool
Undress images for free

Clothoff.io
AI clothes remover

Video Face Swap
Swap faces in any video effortlessly with our completely free AI face swap tool!

Hot Article

Hot Tools

Notepad++7.3.1
Easy-to-use and free code editor

SublimeText3 Chinese version
Chinese version, very easy to use

Zend Studio 13.0.1
Powerful PHP integrated development environment

Dreamweaver CS6
Visual web development tools

SublimeText3 Mac version
God-level code editing software (SublimeText3)

Hot Topics


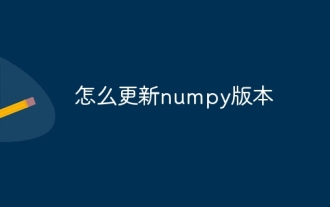
How to update the numpy version: 1. Use the "pip install --upgrade numpy" command; 2. If you are using the Python 3.x version, use the "pip3 install --upgrade numpy" command, which will download and install it, overwriting the current NumPy Version; 3. If you are using conda to manage the Python environment, use the "conda install --update numpy" command to update.
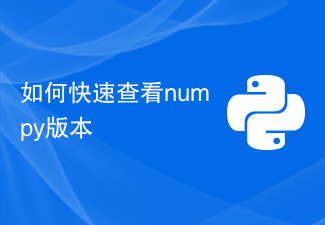
Numpy is an important mathematics library in Python. It provides efficient array operations and scientific calculation functions and is widely used in data analysis, machine learning, deep learning and other fields. When using numpy, we often need to check the version number of numpy to determine the functions supported by the current environment. This article will introduce how to quickly check the numpy version and provide specific code examples. Method 1: Use the __version__ attribute that comes with numpy. The numpy module comes with a __
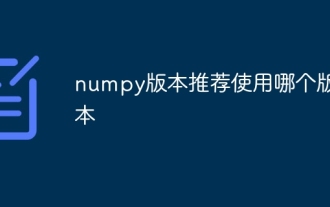
It is recommended to use the latest version of NumPy1.21.2. The reason is: Currently, the latest stable version of NumPy is 1.21.2. Generally, it is recommended to use the latest version of NumPy, as it contains the latest features and performance optimizations, and fixes some issues and bugs in previous versions.
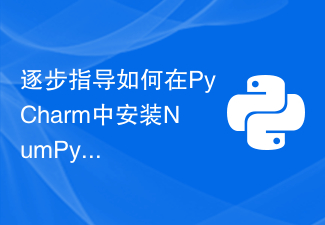
Teach you step by step to install NumPy in PyCharm and make full use of its powerful functions. Preface: NumPy is one of the basic libraries for scientific computing in Python. It provides high-performance multi-dimensional array objects and various functions required to perform basic operations on arrays. function. It is an important part of most data science and machine learning projects. This article will introduce you to how to install NumPy in PyCharm, and demonstrate its powerful features through specific code examples. Step 1: Install PyCharm First, we
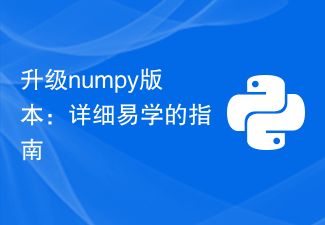
How to upgrade numpy version: Easy-to-follow tutorial, requires concrete code examples Introduction: NumPy is an important Python library used for scientific computing. It provides a powerful multidimensional array object and a series of related functions that can be used to perform efficient numerical operations. As new versions are released, newer features and bug fixes are constantly available to us. This article will describe how to upgrade your installed NumPy library to get the latest features and resolve known issues. Step 1: Check the current NumPy version at the beginning
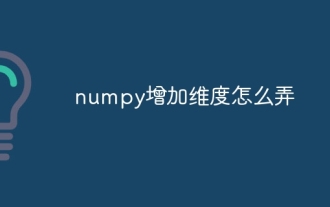
How to add dimensions in numpy: 1. Use "np.newaxis" to add dimensions. "np.newaxis" is a special index value used to insert a new dimension at a specified position. You can use np.newaxis at the corresponding position. To increase the dimension; 2. Use "np.expand_dims()" to increase the dimension. The "np.expand_dims()" function can insert a new dimension at the specified position to increase the dimension of the array.
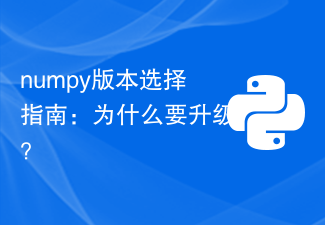
With the rapid development of fields such as data science, machine learning, and deep learning, Python has become a mainstream language for data analysis and modeling. In Python, NumPy (short for NumericalPython) is a very important library because it provides a set of efficient multi-dimensional array objects and is the basis for many other libraries such as pandas, SciPy and scikit-learn. In the process of using NumPy, you are likely to encounter compatibility issues between different versions, then
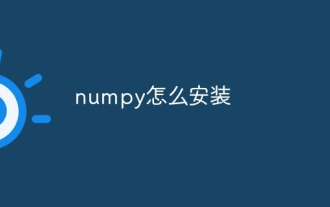
Numpy can be installed using pip, conda, source code and Anaconda. Detailed introduction: 1. pip, enter pip install numpy in the command line; 2. conda, enter conda install numpy in the command line; 3. Source code, unzip the source code package or enter the source code directory, enter in the command line python setup.py build python setup.py install.
