Grid search process for optimizing svm parameters
SVM is a classic supervised learning algorithm commonly used for classification and regression problems. The core idea is to separate different categories of data by finding an optimal hyperplane. In order to further optimize the performance of the SVM model, grid search is often used for parameter optimization. Grid search tries different parameter combinations to find the optimal parameter combination to improve the performance of the model. This process can effectively help us adjust the hyperparameters of the model to achieve better prediction results.
The detailed process of SVM grid search will be introduced below.
First of all, we first understand that there are two key parameters in the SVM algorithm: C and gamma.
1.C parameter
The C parameter is the penalty coefficient of SVM. The smaller the value, the higher the tolerance of the model to misclassification. , tend to choose larger intervals rather than pursue perfect classification. The larger C is, the less tolerance the model has for misclassification, and it tends to choose a smaller interval in order to pursue higher classification accuracy.
2.gamma parameter
gamma is a parameter of the kernel function, which controls the distribution of data points in high-dimensional space. The larger the gamma, the better the model fits the training set, but the poorer its generalization ability for unknown data. The smaller the gamma, the better the model's generalization ability for unknown data, but it may lead to overfitting the training data.
SVM grid search is an exhaustive parameter search method. It tests different parameter combinations to find the optimal parameter combination to improve the performance of the model. The process of SVM grid search is as follows:
1. Define the parameter search range
First you need to clarify the parameter range to be searched. For the C and gamma parameters, a range can be defined, such as [0.1,1,10]. This range can be adjusted according to actual conditions.
2. Construct parameter combinations
Combine the defined parameter ranges to obtain all possible parameter combinations. For example, for the C and gamma parameter ranges [0.1,1,10], there are 9 combinations, namely (0.1,0.1), (0.1,1), (0.1,10), (1,0.1), (1 ,1),(1,10),(10,0.1),(10,1),(10,10).
3. Training model and evaluating performance
For each parameter combination, use the cross-validation method for model training and performance evaluation. Divide the training data into K subsets, use K-1 subsets for training each time, and the remaining subset is used to verify model performance. Cross-validation can help reduce the risk of overfitting and improve the reliability of the model.
4. Select the optimal parameters
Based on the results of cross-validation, select the parameter combination with the best performance as the optimal parameter. Indicators such as accuracy, precision, recall, and F1 value are usually used to evaluate model performance.
5. Use optimal parameters for prediction
Use the selected optimal parameter combination to train the model and make predictions. The optimal parameter combination can improve the performance of the model and improve the model's generalization ability to unknown data.
The following is a sample code for implementing SVM grid search using Python. We will use the scikit-learn library to build SVM models and perform grid searches. It is assumed here that we have imported the necessary libraries and datasets.
# 导入必要的库 from sklearn.model_selection import GridSearchCV from sklearn.svm import SVC # 定义要搜索的参数范围 param_grid = {'C': [0.1, 1, 10], 'gamma': [0.1, 1, 10]} # 初始化SVM模型 svm = SVC() # 构建网格搜索对象 grid_search = GridSearchCV(svm, param_grid, cv=5) # 进行网格搜索 grid_search.fit(X_train, y_train) # 输出最优参数和最优得分 print("Best parameters: {}".format(grid_search.best_params_)) print("Best cross-validation score: {:.2f}".format(grid_search.best_score_))
Code explanation:
1) First define the parameter range param_grid to be searched, in which C and gamma have values of 0.1, 1 and 10 respectively.
2) Then initialize the SVM model svm.
3) Then use GridSearchCV to build the grid search object grid_search. Among them, the cv parameter specifies the cross-validation method used, and 5-fold cross-validation is selected here.
4) Finally, call the fit method to perform a grid search to obtain the optimal parameters and optimal score.
It should be noted that the data sets X_train and y_train here should have been preprocessed. If preprocessing is required, you can use the preprocessing functions in the scikit-learn library, such as StandardScaler for standardization.
In addition, you can add other parameters to GridSearchCV, such as n_jobs to specify the number of CPU cores used, verbose to specify the level of output detailed information, etc.
In short, SVM grid search is a commonly used parameter optimization method. It tests different parameter combinations to find the optimal parameter combination to improve the performance of the model. When performing grid search, you need to pay attention to issues such as data preprocessing, computational cost, selection of parameter ranges, and selection of cross-validation to ensure the reliability and accuracy of the results.
The above is the detailed content of Grid search process for optimizing svm parameters. For more information, please follow other related articles on the PHP Chinese website!

Hot AI Tools

Undresser.AI Undress
AI-powered app for creating realistic nude photos

AI Clothes Remover
Online AI tool for removing clothes from photos.

Undress AI Tool
Undress images for free

Clothoff.io
AI clothes remover

AI Hentai Generator
Generate AI Hentai for free.

Hot Article

Hot Tools

Notepad++7.3.1
Easy-to-use and free code editor

SublimeText3 Chinese version
Chinese version, very easy to use

Zend Studio 13.0.1
Powerful PHP integrated development environment

Dreamweaver CS6
Visual web development tools

SublimeText3 Mac version
God-level code editing software (SublimeText3)

Hot Topics
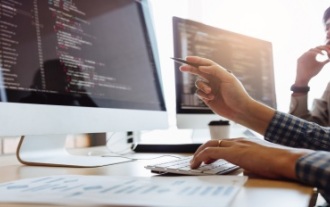
Image annotation is the process of associating labels or descriptive information with images to give deeper meaning and explanation to the image content. This process is critical to machine learning, which helps train vision models to more accurately identify individual elements in images. By adding annotations to images, the computer can understand the semantics and context behind the images, thereby improving the ability to understand and analyze the image content. Image annotation has a wide range of applications, covering many fields, such as computer vision, natural language processing, and graph vision models. It has a wide range of applications, such as assisting vehicles in identifying obstacles on the road, and helping in the detection and diagnosis of diseases through medical image recognition. . This article mainly recommends some better open source and free image annotation tools. 1.Makesens
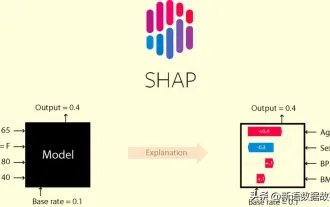
In the fields of machine learning and data science, model interpretability has always been a focus of researchers and practitioners. With the widespread application of complex models such as deep learning and ensemble methods, understanding the model's decision-making process has become particularly important. Explainable AI|XAI helps build trust and confidence in machine learning models by increasing the transparency of the model. Improving model transparency can be achieved through methods such as the widespread use of multiple complex models, as well as the decision-making processes used to explain the models. These methods include feature importance analysis, model prediction interval estimation, local interpretability algorithms, etc. Feature importance analysis can explain the decision-making process of a model by evaluating the degree of influence of the model on the input features. Model prediction interval estimate
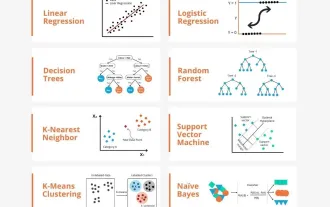
In layman’s terms, a machine learning model is a mathematical function that maps input data to a predicted output. More specifically, a machine learning model is a mathematical function that adjusts model parameters by learning from training data to minimize the error between the predicted output and the true label. There are many models in machine learning, such as logistic regression models, decision tree models, support vector machine models, etc. Each model has its applicable data types and problem types. At the same time, there are many commonalities between different models, or there is a hidden path for model evolution. Taking the connectionist perceptron as an example, by increasing the number of hidden layers of the perceptron, we can transform it into a deep neural network. If a kernel function is added to the perceptron, it can be converted into an SVM. this one
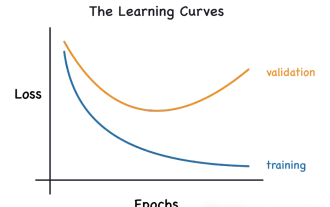
This article will introduce how to effectively identify overfitting and underfitting in machine learning models through learning curves. Underfitting and overfitting 1. Overfitting If a model is overtrained on the data so that it learns noise from it, then the model is said to be overfitting. An overfitted model learns every example so perfectly that it will misclassify an unseen/new example. For an overfitted model, we will get a perfect/near-perfect training set score and a terrible validation set/test score. Slightly modified: "Cause of overfitting: Use a complex model to solve a simple problem and extract noise from the data. Because a small data set as a training set may not represent the correct representation of all data." 2. Underfitting Heru
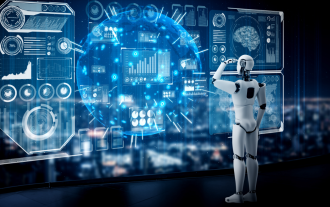
In the 1950s, artificial intelligence (AI) was born. That's when researchers discovered that machines could perform human-like tasks, such as thinking. Later, in the 1960s, the U.S. Department of Defense funded artificial intelligence and established laboratories for further development. Researchers are finding applications for artificial intelligence in many areas, such as space exploration and survival in extreme environments. Space exploration is the study of the universe, which covers the entire universe beyond the earth. Space is classified as an extreme environment because its conditions are different from those on Earth. To survive in space, many factors must be considered and precautions must be taken. Scientists and researchers believe that exploring space and understanding the current state of everything can help understand how the universe works and prepare for potential environmental crises
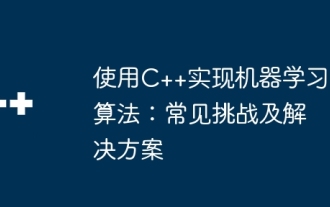
Common challenges faced by machine learning algorithms in C++ include memory management, multi-threading, performance optimization, and maintainability. Solutions include using smart pointers, modern threading libraries, SIMD instructions and third-party libraries, as well as following coding style guidelines and using automation tools. Practical cases show how to use the Eigen library to implement linear regression algorithms, effectively manage memory and use high-performance matrix operations.
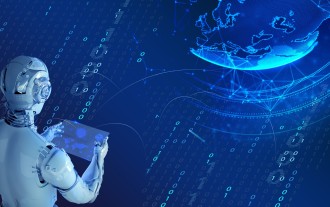
Translator | Reviewed by Li Rui | Chonglou Artificial intelligence (AI) and machine learning (ML) models are becoming increasingly complex today, and the output produced by these models is a black box – unable to be explained to stakeholders. Explainable AI (XAI) aims to solve this problem by enabling stakeholders to understand how these models work, ensuring they understand how these models actually make decisions, and ensuring transparency in AI systems, Trust and accountability to address this issue. This article explores various explainable artificial intelligence (XAI) techniques to illustrate their underlying principles. Several reasons why explainable AI is crucial Trust and transparency: For AI systems to be widely accepted and trusted, users need to understand how decisions are made
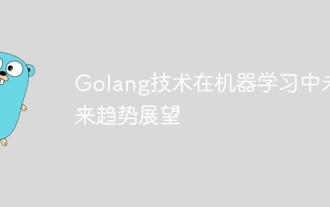
The application potential of Go language in the field of machine learning is huge. Its advantages are: Concurrency: It supports parallel programming and is suitable for computationally intensive operations in machine learning tasks. Efficiency: The garbage collector and language features ensure that the code is efficient, even when processing large data sets. Ease of use: The syntax is concise, making it easy to learn and write machine learning applications.
