Scale Invariant Features (SIFT) algorithm
The Scale Invariant Feature Transform (SIFT) algorithm is a feature extraction algorithm used in the fields of image processing and computer vision. This algorithm was proposed in 1999 to improve object recognition and matching performance in computer vision systems. The SIFT algorithm is robust and accurate and is widely used in image recognition, three-dimensional reconstruction, target detection, video tracking and other fields. It achieves scale invariance by detecting key points in multiple scale spaces and extracting local feature descriptors around the key points. The main steps of the SIFT algorithm include scale space construction, key point detection, key point positioning, direction assignment and feature descriptor generation. Through these steps, the SIFT algorithm can extract robust and unique features to achieve efficient recognition and matching of images.
The SIFT algorithm has the main feature of being invariant to changes in the scale, rotation and brightness of the image, and can extract unique and stable feature points to achieve efficient matching and recognition. . Its main steps include scale space extreme value detection, key point positioning, direction assignment, key point description and matching, etc. Through scale space extreme value detection, the SIFT algorithm can detect extreme points in images at different scales. In the key point positioning stage, key points with stability and uniqueness are determined through local extreme value detection and edge response elimination. The direction assignment stage assigns the dominant direction to each key point to improve the rotation invariance of feature description. The key point description stage uses the image gradient information around the key points to generate features
1. Scale space extreme value detection
Through the Gaussian difference function The original image undergoes scale space processing in order to detect extreme points with different scales. Then, the DoG operator is used to detect these extreme points, that is, the differences between two adjacent layers of Gaussian images in Gaussian pyramids of different scales and spatial positions are compared to obtain scale-invariant key points.
2. Key point positioning
Next, the SIFT algorithm assigns directions to each key point to ensure invariance to rotation transformation . Direction allocation uses the gradient histogram statistical method to calculate the gradient value and direction of the pixels around each key point, then distribute these values to the gradient histogram, and finally select the largest peak in the histogram as the main point of the key point. direction.
3. Direction allocation
After key point positioning and direction allocation, the SIFT algorithm uses the feature descriptor of the local image block to describe each Regional characteristics of key points. The descriptor is constructed based on pixels around key points to ensure invariance to rotation, scale and brightness changes. Specifically, the SIFT algorithm divides the image blocks around the key point into several sub-regions, then calculates the gradient magnitude and direction of the pixels in each sub-region, and constructs a 128-dimensional feature vector to describe the local characteristics of the key point. .
4. Key point description and matching
Finally, the SIFT algorithm performs image matching by comparing the key point feature vectors in the two images. . Specifically, the algorithm evaluates the similarity between two feature vectors by calculating their Euclidean distance or cosine similarity, thereby achieving feature matching and target recognition.
How does the scale-invariant feature transformation algorithm detect key points in images?
The SIFT algorithm performs scale space processing on the original image through the Gaussian difference function to detect extreme points with different scales. Specifically, the SIFT algorithm realizes the scale transformation of the image by constructing a Gaussian pyramid, that is, continuously convolving and downsampling the original image to obtain a series of Gaussian images with different scales. Then, the scale-invariant key points are obtained by performing a difference operation, that is, the DoG operator, on two adjacent layers of Gaussian images.
Before performing the DoG operator operation, it is necessary to determine the number of layers of the Gaussian pyramid and the scale of each layer of the image. The SIFT algorithm usually divides the Gaussian pyramid into several layers, and the size of each layer's image is half of the previous layer's image. This ensures that the scale change of the image will not affect the detection of key points. For each image layer, the SIFT algorithm also selects multiple scales in order to detect key points at different scales.
After determining the number of layers of the Gaussian pyramid and the scale of the image in each layer, the SIFT algorithm will look for extreme points on each image layer, that is, in each layer of the Gaussian pyramid. Among the 26 pixels around a pixel, find the maximum or minimum value and compare it with the corresponding pixels in the two adjacent layers of Gaussian pyramid to determine whether the point is an extreme point in scale space. This enables the detection of key points with stability and uniqueness in images of different scales. It should be noted that the SIFT algorithm will also perform some screening of the detected extreme points, such as excluding low contrast and edge points.
After determining the location of the key points, the SIFT algorithm will also perform key point positioning and direction allocation to ensure invariance to rotation transformation. Specifically, the SIFT algorithm calculates the gradient value and direction of the pixels around each key point and assigns these values to the gradient histogram. Then, the SIFT algorithm will select the largest peak in the histogram as the main direction of the key point and use it as the direction of the point. This ensures that the key points are rotationally invariant and provides direction information for subsequent feature description.
It should be noted that the detection and positioning of key points in the SIFT algorithm are based on the Gaussian pyramid and DoG operator, so the algorithm has good robustness to changes in the scale of the image. . However, the SIFT algorithm has high computational complexity and requires a large number of image convolution and difference operations. Therefore, certain optimization and acceleration are required in practical applications, such as using integral image and fast filter technologies.
In general, the SIFT algorithm, as an effective feature extraction algorithm, has strong robustness and accuracy, and can effectively handle the scale, rotation and Brightness and other transformations to achieve efficient matching and recognition of images. This algorithm has been widely used in the fields of computer vision and image processing, making important contributions to the development of computer vision systems.
The above is the detailed content of Scale Invariant Features (SIFT) algorithm. For more information, please follow other related articles on the PHP Chinese website!

Hot AI Tools

Undresser.AI Undress
AI-powered app for creating realistic nude photos

AI Clothes Remover
Online AI tool for removing clothes from photos.

Undress AI Tool
Undress images for free

Clothoff.io
AI clothes remover

Video Face Swap
Swap faces in any video effortlessly with our completely free AI face swap tool!

Hot Article

Hot Tools

Notepad++7.3.1
Easy-to-use and free code editor

SublimeText3 Chinese version
Chinese version, very easy to use

Zend Studio 13.0.1
Powerful PHP integrated development environment

Dreamweaver CS6
Visual web development tools

SublimeText3 Mac version
God-level code editing software (SublimeText3)

Hot Topics










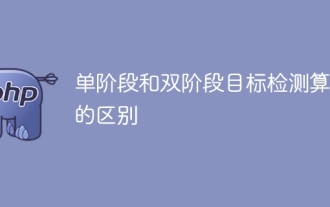
Object detection is an important task in the field of computer vision, used to identify objects in images or videos and locate their locations. This task is usually divided into two categories of algorithms, single-stage and two-stage, which differ in terms of accuracy and robustness. Single-stage target detection algorithm The single-stage target detection algorithm converts target detection into a classification problem. Its advantage is that it is fast and can complete the detection in just one step. However, due to oversimplification, the accuracy is usually not as good as the two-stage object detection algorithm. Common single-stage target detection algorithms include YOLO, SSD and FasterR-CNN. These algorithms generally take the entire image as input and run a classifier to identify the target object. Unlike traditional two-stage target detection algorithms, they do not need to define areas in advance, but directly predict
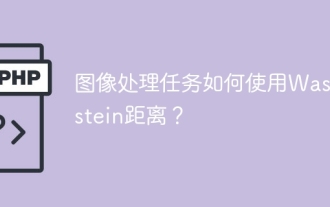
Wasserstein distance, also known as EarthMover's Distance (EMD), is a metric used to measure the difference between two probability distributions. Compared with traditional KL divergence or JS divergence, Wasserstein distance takes into account the structural information between distributions and therefore exhibits better performance in many image processing tasks. By calculating the minimum transportation cost between two distributions, Wasserstein distance is able to measure the minimum amount of work required to transform one distribution into another. This metric is able to capture the geometric differences between distributions, thereby playing an important role in tasks such as image generation and style transfer. Therefore, the Wasserstein distance becomes the concept
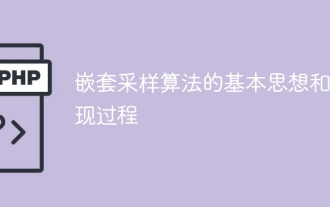
The nested sampling algorithm is an efficient Bayesian statistical inference algorithm used to calculate the integral or summation under complex probability distributions. It works by decomposing the parameter space into multiple hypercubes of equal volume, and gradually and iteratively "pushing out" one of the smallest volume hypercubes, and then filling the hypercube with random samples to better estimate the integral value of the probability distribution. . Through continuous iteration, the nested sampling algorithm can obtain high-precision integral values and boundaries of parameter space, which can be applied to statistical problems such as model comparison, parameter estimation, and model selection. The core idea of this algorithm is to transform complex integration problems into a series of simple integration problems, and approach the real integral value by gradually reducing the volume of the parameter space. Each iteration step randomly samples from the parameter space
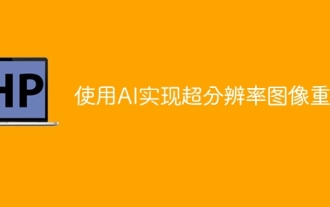
Super-resolution image reconstruction is the process of generating high-resolution images from low-resolution images using deep learning techniques, such as convolutional neural networks (CNN) and generative adversarial networks (GAN). The goal of this method is to improve the quality and detail of images by converting low-resolution images into high-resolution images. This technology has wide applications in many fields, such as medical imaging, surveillance cameras, satellite images, etc. Through super-resolution image reconstruction, we can obtain clearer and more detailed images, which helps to more accurately analyze and identify targets and features in images. Reconstruction methods Super-resolution image reconstruction methods can generally be divided into two categories: interpolation-based methods and deep learning-based methods. 1) Interpolation-based method Super-resolution image reconstruction based on interpolation
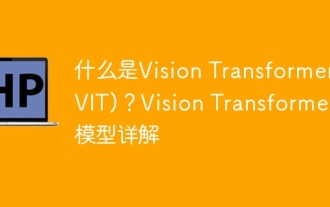
VisionTransformer (VIT) is a Transformer-based image classification model proposed by Google. Different from traditional CNN models, VIT represents images as sequences and learns the image structure by predicting the class label of the image. To achieve this, VIT divides the input image into multiple patches and concatenates the pixels in each patch through channels and then performs linear projection to achieve the desired input dimensions. Finally, each patch is flattened into a single vector, forming the input sequence. Through Transformer's self-attention mechanism, VIT is able to capture the relationship between different patches and perform effective feature extraction and classification prediction. This serialized image representation is
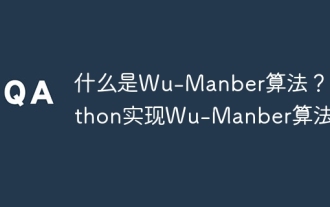
The Wu-Manber algorithm is a string matching algorithm used to search strings efficiently. It is a hybrid algorithm that combines the advantages of Boyer-Moore and Knuth-Morris-Pratt algorithms to provide fast and accurate pattern matching. Wu-Manber algorithm step 1. Create a hash table that maps each possible substring of the pattern to the pattern position where that substring occurs. 2. This hash table is used to quickly identify potential starting locations of patterns in text. 3. Iterate through the text and compare each character to the corresponding character in the pattern. 4. If the characters match, you can move to the next character and continue the comparison. 5. If the characters do not match, you can use a hash table to determine the next potential character in the pattern.
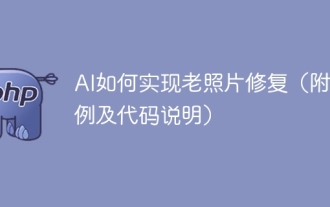
Old photo restoration is a method of using artificial intelligence technology to repair, enhance and improve old photos. Using computer vision and machine learning algorithms, the technology can automatically identify and repair damage and flaws in old photos, making them look clearer, more natural and more realistic. The technical principles of old photo restoration mainly include the following aspects: 1. Image denoising and enhancement. When restoring old photos, they need to be denoised and enhanced first. Image processing algorithms and filters, such as mean filtering, Gaussian filtering, bilateral filtering, etc., can be used to solve noise and color spots problems, thereby improving the quality of photos. 2. Image restoration and repair In old photos, there may be some defects and damage, such as scratches, cracks, fading, etc. These problems can be solved by image restoration and repair algorithms
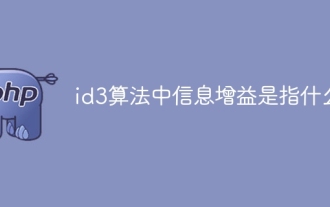
The ID3 algorithm is one of the basic algorithms in decision tree learning. It selects the best split point by calculating the information gain of each feature to generate a decision tree. Information gain is an important concept in the ID3 algorithm, which is used to measure the contribution of features to the classification task. This article will introduce in detail the concept, calculation method and application of information gain in the ID3 algorithm. 1. The concept of information entropy Information entropy is a concept in information theory, which measures the uncertainty of random variables. For a discrete random variable number, and p(x_i) represents the probability that the random variable X takes the value x_i. letter
