Introducing the concepts and methods of ensemble learning
Ensemble learning is a machine learning method that improves classification performance by combining multiple classifiers. It uses the wisdom of multiple classifiers to weight or vote their classification results to get more accurate classification results. Ensemble learning can effectively improve the accuracy, generalization ability and stability of classification models.
Ensemble learning methods can be divided into two major categories: sample-based methods and model-based methods.
Sample-based method
Bagging (bootstrap aggregation method) is a method of repeatedly sampling a data set with random replacement . Improve classification accuracy and stability by training multiple classifiers and averaging or voting on their results.
Boosting is a method that weights samples. Its purpose is to focus on misclassified samples, thereby making the classifier more sensitive to these samples, thereby improving Classification performance. Common Boosting algorithms include AdaBoost and Gradient Boosting. By adjusting the weight of samples, the Boosting algorithm can effectively improve the accuracy of the classifier. The AdaBoost algorithm gradually improves the performance of the overall classifier by iteratively training multiple weak classifiers and adjusting sample weights based on the error rate of the previous classifier. The Gradient Boosting algorithm iteratively trains multiple weak classifiers and uses the gradient descent method to minimize the loss.
Random Forest (Random Forest): It is a method based on Bagging Decision tree ensemble algorithm. It constructs multiple trees by randomly selecting features and samples, and finally weights the results of all trees or votes.
Model-based method
Stacking: By taking the prediction results of multiple basic classifiers as input, a meta- classifier to obtain the final classification result. Stacking can be trained and tested through cross-validation.
Adaboost.M1: Based on the Boosting idea, using exponential loss function and weight distribution strategy, iteratively trains multiple weak classifiers, and finally combines them to obtain a strong classifier.
Gradient Boosting Machine (GBM): Based on the Boosting idea, gradient descent is used to optimize the loss function, and iteratively trains multiple weak classifiers to finally obtain a strong classifier.
It should be noted that the integrated learning method is not omnipotent, and its performance improvement also has certain limitations. In practical applications, it is necessary to select appropriate integration methods according to specific scenarios and use them in combination with other technical means to achieve the best results.
Variant methods and techniques
In addition, integrated learning also has some other variant methods and techniques, such as:
Weighted Voting: The weights of different classifiers can be different, and the accuracy of the classifier can be further improved by adjusting the weights.
Cross-Validation Ensemble: Use the cross-validation method to construct multiple training sets and test sets, train multiple classifiers respectively, and compare the results of all classifiers Average or vote to get more accurate classification results.
Consensus Voting: Using the different characteristics of different classifiers, classify each sample multiple times, and finally perform a weighted average or vote on all classification results, so as to Get more accurate classification results.
In short, ensemble learning is a very useful machine learning method that can effectively improve the performance and generalization ability of classification models. In practical applications, it is necessary to select appropriate integration methods according to specific scenarios and use them in combination with other technical means to achieve the best results.
The above is the detailed content of Introducing the concepts and methods of ensemble learning. For more information, please follow other related articles on the PHP Chinese website!

Hot AI Tools

Undresser.AI Undress
AI-powered app for creating realistic nude photos

AI Clothes Remover
Online AI tool for removing clothes from photos.

Undress AI Tool
Undress images for free

Clothoff.io
AI clothes remover

AI Hentai Generator
Generate AI Hentai for free.

Hot Article

Hot Tools

Notepad++7.3.1
Easy-to-use and free code editor

SublimeText3 Chinese version
Chinese version, very easy to use

Zend Studio 13.0.1
Powerful PHP integrated development environment

Dreamweaver CS6
Visual web development tools

SublimeText3 Mac version
God-level code editing software (SublimeText3)

Hot Topics
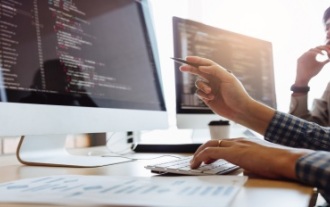
Image annotation is the process of associating labels or descriptive information with images to give deeper meaning and explanation to the image content. This process is critical to machine learning, which helps train vision models to more accurately identify individual elements in images. By adding annotations to images, the computer can understand the semantics and context behind the images, thereby improving the ability to understand and analyze the image content. Image annotation has a wide range of applications, covering many fields, such as computer vision, natural language processing, and graph vision models. It has a wide range of applications, such as assisting vehicles in identifying obstacles on the road, and helping in the detection and diagnosis of diseases through medical image recognition. . This article mainly recommends some better open source and free image annotation tools. 1.Makesens
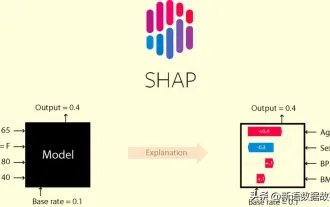
In the fields of machine learning and data science, model interpretability has always been a focus of researchers and practitioners. With the widespread application of complex models such as deep learning and ensemble methods, understanding the model's decision-making process has become particularly important. Explainable AI|XAI helps build trust and confidence in machine learning models by increasing the transparency of the model. Improving model transparency can be achieved through methods such as the widespread use of multiple complex models, as well as the decision-making processes used to explain the models. These methods include feature importance analysis, model prediction interval estimation, local interpretability algorithms, etc. Feature importance analysis can explain the decision-making process of a model by evaluating the degree of influence of the model on the input features. Model prediction interval estimate
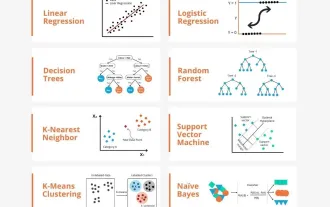
In layman’s terms, a machine learning model is a mathematical function that maps input data to a predicted output. More specifically, a machine learning model is a mathematical function that adjusts model parameters by learning from training data to minimize the error between the predicted output and the true label. There are many models in machine learning, such as logistic regression models, decision tree models, support vector machine models, etc. Each model has its applicable data types and problem types. At the same time, there are many commonalities between different models, or there is a hidden path for model evolution. Taking the connectionist perceptron as an example, by increasing the number of hidden layers of the perceptron, we can transform it into a deep neural network. If a kernel function is added to the perceptron, it can be converted into an SVM. this one
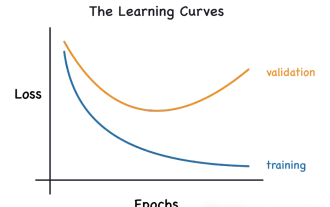
This article will introduce how to effectively identify overfitting and underfitting in machine learning models through learning curves. Underfitting and overfitting 1. Overfitting If a model is overtrained on the data so that it learns noise from it, then the model is said to be overfitting. An overfitted model learns every example so perfectly that it will misclassify an unseen/new example. For an overfitted model, we will get a perfect/near-perfect training set score and a terrible validation set/test score. Slightly modified: "Cause of overfitting: Use a complex model to solve a simple problem and extract noise from the data. Because a small data set as a training set may not represent the correct representation of all data." 2. Underfitting Heru
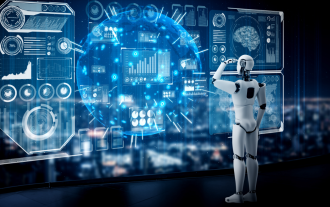
In the 1950s, artificial intelligence (AI) was born. That's when researchers discovered that machines could perform human-like tasks, such as thinking. Later, in the 1960s, the U.S. Department of Defense funded artificial intelligence and established laboratories for further development. Researchers are finding applications for artificial intelligence in many areas, such as space exploration and survival in extreme environments. Space exploration is the study of the universe, which covers the entire universe beyond the earth. Space is classified as an extreme environment because its conditions are different from those on Earth. To survive in space, many factors must be considered and precautions must be taken. Scientists and researchers believe that exploring space and understanding the current state of everything can help understand how the universe works and prepare for potential environmental crises
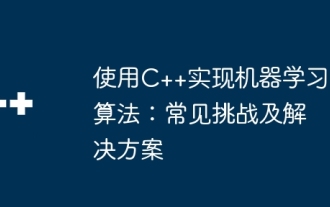
Common challenges faced by machine learning algorithms in C++ include memory management, multi-threading, performance optimization, and maintainability. Solutions include using smart pointers, modern threading libraries, SIMD instructions and third-party libraries, as well as following coding style guidelines and using automation tools. Practical cases show how to use the Eigen library to implement linear regression algorithms, effectively manage memory and use high-performance matrix operations.
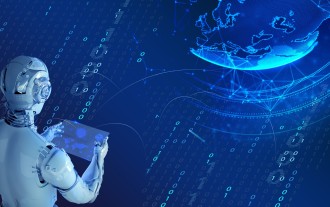
Translator | Reviewed by Li Rui | Chonglou Artificial intelligence (AI) and machine learning (ML) models are becoming increasingly complex today, and the output produced by these models is a black box – unable to be explained to stakeholders. Explainable AI (XAI) aims to solve this problem by enabling stakeholders to understand how these models work, ensuring they understand how these models actually make decisions, and ensuring transparency in AI systems, Trust and accountability to address this issue. This article explores various explainable artificial intelligence (XAI) techniques to illustrate their underlying principles. Several reasons why explainable AI is crucial Trust and transparency: For AI systems to be widely accepted and trusted, users need to understand how decisions are made
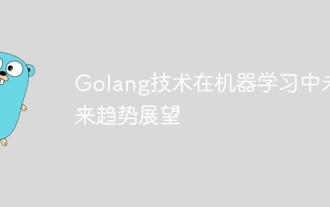
The application potential of Go language in the field of machine learning is huge. Its advantages are: Concurrency: It supports parallel programming and is suitable for computationally intensive operations in machine learning tasks. Efficiency: The garbage collector and language features ensure that the code is efficient, even when processing large data sets. Ease of use: The syntax is concise, making it easy to learn and write machine learning applications.
