Edge Intelligence: Definition, Applications, Components and Benefits
Edge intelligence is a further development of edge computing. It allows smart sensor nodes to make decisions locally, with the option to send data to a gateway for further filtering and finally to the cloud or other storage system. Edge intelligence combines artificial intelligence and edge computing so that it no longer relies on the cloud, but directly senses information locally and responds quickly to changes in the environment through real-time decision-making applications. The emergence of this technology will bring more efficient data processing and faster decision-making capabilities to various industries.
Applications based on edge intelligence
Machine learning aspect
Machine learning (ML) is The core of edge intelligence, it detects patterns and anomalies in data streams to trigger appropriate responses. ML has a wide range of applications, including factories, smart cities, smart grids, augmented and virtual reality, connected vehicles, and healthcare systems. ML models are trained in the cloud and then used to empower edge devices with intelligence.
Machine learning is an effective way to create functional AI. ML techniques such as decision trees, Bayesian networks, and K-means clustering are used for classification and prediction of AI entities. Deep learning uses artificial neural networks to perform tasks such as image classification and face recognition.
Artificial intelligence aspect
Machine learning has become very popular in manufacturing, while artificial intelligence is more used to extract content from social media , business informatics, and big data collected in things like online shopping records. Transmitting large amounts of data from IoT devices to the cloud for analysis is expensive and inefficient. Edge intelligence pushes cloud services from the core of the network to the edge of the network for processing. The edge node sensor can be a smartphone or other mobile device.
Among them, real-time video analysis is expected to become a very popular application for edge computing. As an application built on computer vision, real-time video analytics will continuously collect high-definition video captured from surveillance cameras. These applications require high computation, high bandwidth, and low latency to analyze video. This can be achieved by extending the cloud’s AI to gateways covering the edge.
Benefits of edge intelligence
1. Low latency intelligence
Cloud computing or centralized The system has been having latency issues. Capturing data and sending it to a central location, processing and responding takes time and does not facilitate real-time decision making.
The core benefit of edge intelligence is to reduce latency, thereby executing actionable events in near real-time, thereby improving overall system performance. This also frees cloud computing and centralized systems from having to deal with raw and irrelevant data; they can instead process highly structured, context-rich, actionable data. In this way, latency is improved not only at the edge, but also across the entire system.
2. Low-bandwidth data storage
In any IoT model, transmitting all the data collected by thousands of edge devices has a significant impact on bandwidth. The requirements are very high. As the number of these devices increases, it will grow exponentially. The remote site location may not even have the bandwidth to transfer data and analytics back and forth from the cloud server. Edge intelligence helps perform analysis and take required actions. It can store data, metadata, and operational reports that can be collected later.
3. Linear Scalability
Edge intelligence architecture can scale linearly as IoT deployments grow. Edge intelligence architecture leverages the computing power of deployed devices. It can take on the heavy lifting of executing deep learning and machine learning models. This relieves the pressure on centralized cloud systems as edge devices share the main burden of performing intelligent functions.
4. Reduce operating costs
#Since edge intelligence operates on time-sensitive data locally, it can save a lot of cloud space, Because they provide rich data to central systems. This also reduces operating costs. Edge intelligence drives operations in real time for all connected IoT devices, which enables professionals to deploy and maintain devices more efficiently.
Edge intelligence architecture components
The 4 main components in the edge intelligence architecture: edge caching, edge training, edge inference and edge offloading.
1. Edge caching
Under edge intelligence, edge caching mainly handles the traffic from end users and their surrounding environment to edge devices. Enter distributed data. In addition to this data, data generated by the edge device itself also belongs to the edge cache. In addition, mobile sensors collect environmental data, process and store it in reasonable places for use by artificial intelligence algorithms to provide services to users.
This module is responsible for complete edge data storage management.
2. Edge training
To date, the training of AI models deployed on the intelligent edge has mostly been centralized. We train deep learning models on powerful central servers equipped with powerful GPUs and port them to edge devices using their compatible edge SDKs and runtime environments. This remains the best way to control the retraining and deployment of models to edge devices using cloud connectivity or other IO interfaces. But for a true edge intelligence architecture, we need to implement edge training.
Edge training refers to the process of learning optimal values of weights and biases for a model deployed on data or identifying hidden patterns on training data captured on the edge.
3. Edge reasoning
Edge reasoning is to evaluate the training model or algorithm on the test data set by calculating the output on the edge device. performance process.
4. Edge offloading
Edge offloading is another important feature of edge intelligence, where edge devices can offload certain tasks, such as Offload training, edge caching, or edge inference to other edge devices in the network. It is similar to the distributed computing paradigm where edge devices create intelligent ecosystems. Edge offload is an abstract service layer on top of the other three components, so it is very important that it also provides a fail-safe strategy to overcome the shortcomings of standalone edge devices. Structured and effective implementation will enable edge intelligence to make the most of available resources in the edge environment.
The above is the detailed content of Edge Intelligence: Definition, Applications, Components and Benefits. For more information, please follow other related articles on the PHP Chinese website!

Hot AI Tools

Undresser.AI Undress
AI-powered app for creating realistic nude photos

AI Clothes Remover
Online AI tool for removing clothes from photos.

Undress AI Tool
Undress images for free

Clothoff.io
AI clothes remover

AI Hentai Generator
Generate AI Hentai for free.

Hot Article

Hot Tools

Notepad++7.3.1
Easy-to-use and free code editor

SublimeText3 Chinese version
Chinese version, very easy to use

Zend Studio 13.0.1
Powerful PHP integrated development environment

Dreamweaver CS6
Visual web development tools

SublimeText3 Mac version
God-level code editing software (SublimeText3)

Hot Topics
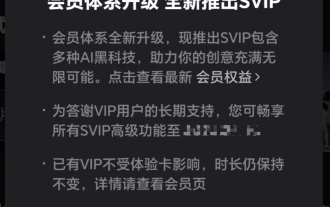
This site reported on June 27 that Jianying is a video editing software developed by FaceMeng Technology, a subsidiary of ByteDance. It relies on the Douyin platform and basically produces short video content for users of the platform. It is compatible with iOS, Android, and Windows. , MacOS and other operating systems. Jianying officially announced the upgrade of its membership system and launched a new SVIP, which includes a variety of AI black technologies, such as intelligent translation, intelligent highlighting, intelligent packaging, digital human synthesis, etc. In terms of price, the monthly fee for clipping SVIP is 79 yuan, the annual fee is 599 yuan (note on this site: equivalent to 49.9 yuan per month), the continuous monthly subscription is 59 yuan per month, and the continuous annual subscription is 499 yuan per year (equivalent to 41.6 yuan per month) . In addition, the cut official also stated that in order to improve the user experience, those who have subscribed to the original VIP
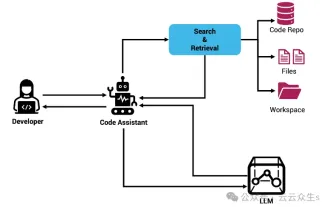
Improve developer productivity, efficiency, and accuracy by incorporating retrieval-enhanced generation and semantic memory into AI coding assistants. Translated from EnhancingAICodingAssistantswithContextUsingRAGandSEM-RAG, author JanakiramMSV. While basic AI programming assistants are naturally helpful, they often fail to provide the most relevant and correct code suggestions because they rely on a general understanding of the software language and the most common patterns of writing software. The code generated by these coding assistants is suitable for solving the problems they are responsible for solving, but often does not conform to the coding standards, conventions and styles of the individual teams. This often results in suggestions that need to be modified or refined in order for the code to be accepted into the application

To learn more about AIGC, please visit: 51CTOAI.x Community https://www.51cto.com/aigc/Translator|Jingyan Reviewer|Chonglou is different from the traditional question bank that can be seen everywhere on the Internet. These questions It requires thinking outside the box. Large Language Models (LLMs) are increasingly important in the fields of data science, generative artificial intelligence (GenAI), and artificial intelligence. These complex algorithms enhance human skills and drive efficiency and innovation in many industries, becoming the key for companies to remain competitive. LLM has a wide range of applications. It can be used in fields such as natural language processing, text generation, speech recognition and recommendation systems. By learning from large amounts of data, LLM is able to generate text
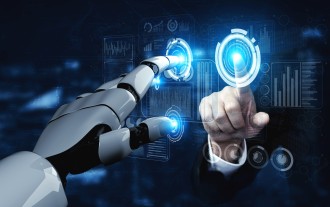
Large Language Models (LLMs) are trained on huge text databases, where they acquire large amounts of real-world knowledge. This knowledge is embedded into their parameters and can then be used when needed. The knowledge of these models is "reified" at the end of training. At the end of pre-training, the model actually stops learning. Align or fine-tune the model to learn how to leverage this knowledge and respond more naturally to user questions. But sometimes model knowledge is not enough, and although the model can access external content through RAG, it is considered beneficial to adapt the model to new domains through fine-tuning. This fine-tuning is performed using input from human annotators or other LLM creations, where the model encounters additional real-world knowledge and integrates it
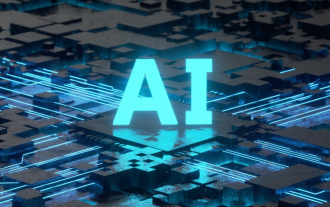
Editor |ScienceAI Question Answering (QA) data set plays a vital role in promoting natural language processing (NLP) research. High-quality QA data sets can not only be used to fine-tune models, but also effectively evaluate the capabilities of large language models (LLM), especially the ability to understand and reason about scientific knowledge. Although there are currently many scientific QA data sets covering medicine, chemistry, biology and other fields, these data sets still have some shortcomings. First, the data form is relatively simple, most of which are multiple-choice questions. They are easy to evaluate, but limit the model's answer selection range and cannot fully test the model's ability to answer scientific questions. In contrast, open-ended Q&A
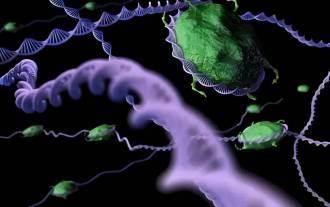
Editor | KX In the field of drug research and development, accurately and effectively predicting the binding affinity of proteins and ligands is crucial for drug screening and optimization. However, current studies do not take into account the important role of molecular surface information in protein-ligand interactions. Based on this, researchers from Xiamen University proposed a novel multi-modal feature extraction (MFE) framework, which for the first time combines information on protein surface, 3D structure and sequence, and uses a cross-attention mechanism to compare different modalities. feature alignment. Experimental results demonstrate that this method achieves state-of-the-art performance in predicting protein-ligand binding affinities. Furthermore, ablation studies demonstrate the effectiveness and necessity of protein surface information and multimodal feature alignment within this framework. Related research begins with "S
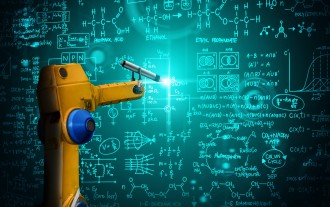
Machine learning is an important branch of artificial intelligence that gives computers the ability to learn from data and improve their capabilities without being explicitly programmed. Machine learning has a wide range of applications in various fields, from image recognition and natural language processing to recommendation systems and fraud detection, and it is changing the way we live. There are many different methods and theories in the field of machine learning, among which the five most influential methods are called the "Five Schools of Machine Learning". The five major schools are the symbolic school, the connectionist school, the evolutionary school, the Bayesian school and the analogy school. 1. Symbolism, also known as symbolism, emphasizes the use of symbols for logical reasoning and expression of knowledge. This school of thought believes that learning is a process of reverse deduction, through existing
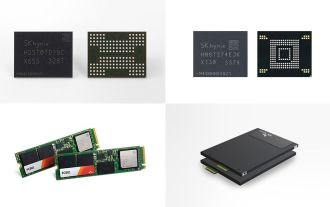
According to news from this site on August 1, SK Hynix released a blog post today (August 1), announcing that it will attend the Global Semiconductor Memory Summit FMS2024 to be held in Santa Clara, California, USA from August 6 to 8, showcasing many new technologies. generation product. Introduction to the Future Memory and Storage Summit (FutureMemoryandStorage), formerly the Flash Memory Summit (FlashMemorySummit) mainly for NAND suppliers, in the context of increasing attention to artificial intelligence technology, this year was renamed the Future Memory and Storage Summit (FutureMemoryandStorage) to invite DRAM and storage vendors and many more players. New product SK hynix launched last year
