Analyzing Meta-Learning Neural Networks for Memory Enhancement
Memory-Augmented Neural Networks (MANNs) are a type of deep learning model that combines neural networks and external memory storage. Compared with traditional neural networks that only rely on internal parameters for calculations, MANNs can store and read data in external memory to achieve more complex calculations and reasoning tasks. This model has excellent memory and generalization capabilities and can better handle various scenarios and problems. By utilizing external memory, MANNs are able to store and retrieve large amounts of data, allowing them to better understand and utilize historical information, thereby improving model performance and effectiveness. Therefore, MANNs have shown great potential in many fields, such as natural language processing, image recognition, and intelligent reasoning.
The core idea of MANNs is to combine external memory with neural networks to achieve storage, access and update of data. Common memories include data structures such as matrices, vectors, graphs, and trees. The appropriate memory type can be selected based on task requirements. In MANNs, memory is viewed as a collection of readable and writable registers, each with a unique address and stored value. Neural networks can access memory through read and write operations, perform calculations on values in the memory as input, and write the calculation results back to the memory. This combination enables MANNs to flexibly store and update information during data processing, thereby improving the processing capabilities and adaptability of neural networks.
The typical structure of MANNs consists of two main parts: controller and memory. The main task of the controller is to determine the read and write operations of the memory and fuse the read information with the calculation results of the neural network. Controllers usually adopt structures such as recurrent neural networks or convolutional neural networks. The memory is responsible for actually storing and reading data, and is usually composed of memory cells based on key-value pairs. Each memory cell includes a key, a value, and a flag bit to indicate whether the cell has been written to. The design of this structure enables MANNs to have higher flexibility and memory capabilities when processing and storing data.
The training process of MANNs usually adopts end-to-end learning. This means that the controller and memory are trained as a whole, rather than individually. During the training process, the controller learns how to fuse the information in the memory with the calculation results of the neural network by reading and writing memory to maximize the performance indicators of the model. These performance metrics can include accuracy, loss functions, task-specific metrics, etc. Through continuous training and optimization, MANNs can gradually improve their performance to better complete specific tasks.
MANNs (Memory Augmented Neural Networks) is a neural network model widely used in various fields. They have important applications in natural language processing, computer vision, reinforcement learning and other fields. Among them, the DNC (Differentiable Neural Computer) model proposed by DeepMind is one of the most famous and widely used MANNs. The DNC model uses an address-based addressing mechanism and an attention mechanism, which gives it excellent generalization and memory capabilities. Therefore, it has been successfully used in many tasks such as natural language generation, image classification, sequence prediction, etc. The emergence of DNC models has greatly promoted the development and application of MANNs in various fields.
In short, memory-enhanced neural network is a type of deep learning model that combines neural network and external memory. It has better memory ability and generalization ability, and is widely used in various field.
The above is the detailed content of Analyzing Meta-Learning Neural Networks for Memory Enhancement. For more information, please follow other related articles on the PHP Chinese website!

Hot AI Tools

Undresser.AI Undress
AI-powered app for creating realistic nude photos

AI Clothes Remover
Online AI tool for removing clothes from photos.

Undress AI Tool
Undress images for free

Clothoff.io
AI clothes remover

AI Hentai Generator
Generate AI Hentai for free.

Hot Article

Hot Tools

Notepad++7.3.1
Easy-to-use and free code editor

SublimeText3 Chinese version
Chinese version, very easy to use

Zend Studio 13.0.1
Powerful PHP integrated development environment

Dreamweaver CS6
Visual web development tools

SublimeText3 Mac version
God-level code editing software (SublimeText3)

Hot Topics


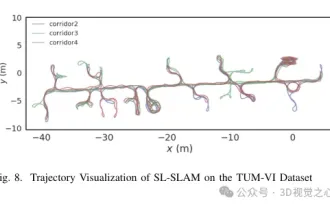
Written previously, today we discuss how deep learning technology can improve the performance of vision-based SLAM (simultaneous localization and mapping) in complex environments. By combining deep feature extraction and depth matching methods, here we introduce a versatile hybrid visual SLAM system designed to improve adaptation in challenging scenarios such as low-light conditions, dynamic lighting, weakly textured areas, and severe jitter. sex. Our system supports multiple modes, including extended monocular, stereo, monocular-inertial, and stereo-inertial configurations. In addition, it also analyzes how to combine visual SLAM with deep learning methods to inspire other research. Through extensive experiments on public datasets and self-sampled data, we demonstrate the superiority of SL-SLAM in terms of positioning accuracy and tracking robustness.
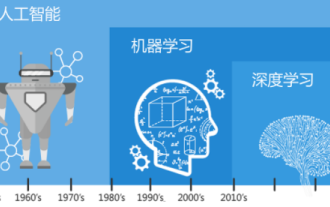
In today's wave of rapid technological changes, Artificial Intelligence (AI), Machine Learning (ML) and Deep Learning (DL) are like bright stars, leading the new wave of information technology. These three words frequently appear in various cutting-edge discussions and practical applications, but for many explorers who are new to this field, their specific meanings and their internal connections may still be shrouded in mystery. So let's take a look at this picture first. It can be seen that there is a close correlation and progressive relationship between deep learning, machine learning and artificial intelligence. Deep learning is a specific field of machine learning, and machine learning
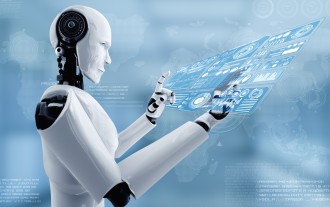
Almost 20 years have passed since the concept of deep learning was proposed in 2006. Deep learning, as a revolution in the field of artificial intelligence, has spawned many influential algorithms. So, what do you think are the top 10 algorithms for deep learning? The following are the top algorithms for deep learning in my opinion. They all occupy an important position in terms of innovation, application value and influence. 1. Deep neural network (DNN) background: Deep neural network (DNN), also called multi-layer perceptron, is the most common deep learning algorithm. When it was first invented, it was questioned due to the computing power bottleneck. Until recent years, computing power, The breakthrough came with the explosion of data. DNN is a neural network model that contains multiple hidden layers. In this model, each layer passes input to the next layer and
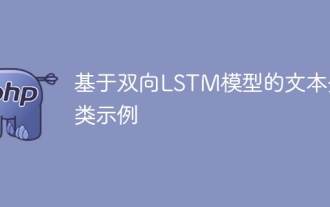
The bidirectional LSTM model is a neural network used for text classification. Below is a simple example demonstrating how to use bidirectional LSTM for text classification tasks. First, we need to import the required libraries and modules: importosimportnumpyasnpfromkeras.preprocessing.textimportTokenizerfromkeras.preprocessing.sequenceimportpad_sequencesfromkeras.modelsimportSequentialfromkeras.layersimportDense,Em

Editor | Radish Skin Since the release of the powerful AlphaFold2 in 2021, scientists have been using protein structure prediction models to map various protein structures within cells, discover drugs, and draw a "cosmic map" of every known protein interaction. . Just now, Google DeepMind released the AlphaFold3 model, which can perform joint structure predictions for complexes including proteins, nucleic acids, small molecules, ions and modified residues. The accuracy of AlphaFold3 has been significantly improved compared to many dedicated tools in the past (protein-ligand interaction, protein-nucleic acid interaction, antibody-antigen prediction). This shows that within a single unified deep learning framework, it is possible to achieve
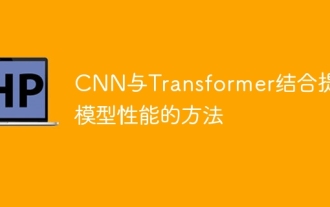
Convolutional Neural Network (CNN) and Transformer are two different deep learning models that have shown excellent performance on different tasks. CNN is mainly used for computer vision tasks such as image classification, target detection and image segmentation. It extracts local features on the image through convolution operations, and performs feature dimensionality reduction and spatial invariance through pooling operations. In contrast, Transformer is mainly used for natural language processing (NLP) tasks such as machine translation, text classification, and speech recognition. It uses a self-attention mechanism to model dependencies in sequences, avoiding the sequential computation in traditional recurrent neural networks. Although these two models are used for different tasks, they have similarities in sequence modeling, so
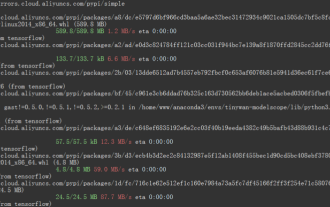
Overview In order to enable ModelScope users to quickly and conveniently use various models provided by the platform, a set of fully functional Python libraries are provided, which includes the implementation of ModelScope official models, as well as the necessary tools for using these models for inference, finetune and other tasks. Code related to data pre-processing, post-processing, effect evaluation and other functions, while also providing a simple and easy-to-use API and rich usage examples. By calling the library, users can complete tasks such as model reasoning, training, and evaluation by writing just a few lines of code. They can also quickly perform secondary development on this basis to realize their own innovative ideas. The algorithm model currently provided by the library is:
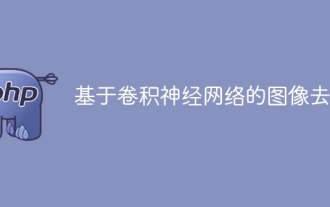
Convolutional neural networks perform well in image denoising tasks. It utilizes the learned filters to filter the noise and thereby restore the original image. This article introduces in detail the image denoising method based on convolutional neural network. 1. Overview of Convolutional Neural Network Convolutional neural network is a deep learning algorithm that uses a combination of multiple convolutional layers, pooling layers and fully connected layers to learn and classify image features. In the convolutional layer, the local features of the image are extracted through convolution operations, thereby capturing the spatial correlation in the image. The pooling layer reduces the amount of calculation by reducing the feature dimension and retains the main features. The fully connected layer is responsible for mapping learned features and labels to implement image classification or other tasks. The design of this network structure makes convolutional neural networks useful in image processing and recognition.
