


Advanced techniques and practical applications of Pandas data filtering
Pandas is a powerful data processing and analysis tool. It provides many flexible functions and methods to easily filter and process data. This article will introduce several advanced techniques for Pandas data filtering and provide specific code examples through actual cases.
1. Basic data filtering
Pandas provides a variety of methods to perform basic filtering of data, such as using Boolean index, loc or iloc method, etc. Here are some common basic data filtering cases.
- Boolean index filtering
Boolean index can be used to filter data based on certain conditions. For example, we have a data frame containing student information, and we want to filter out students with scores greater than 60 points. This can be achieved using the following code:
import pandas as pd data = { '姓名': ['张三', '李四', '王五', '赵六'], '成绩': [80, 70, 90, 50] } df = pd.DataFrame(data) df_filtered = df[df['成绩'] > 60] print(df_filtered)
- loc method filtering
The loc method can filter data based on row labels and column labels. For example, we have a data frame containing student information, and we want to filter out the grades and ages of students named Zhang San and Li Si. You can use the following code to achieve this:
import pandas as pd data = { '姓名': ['张三', '李四', '王五', '赵六'], '成绩': [80, 70, 90, 50], '年龄': [18, 19, 20, 21] } df = pd.DataFrame(data) df_filtered = df.loc[df['姓名'].isin(['张三', '李四']), ['成绩', '年龄']] print(df_filtered)
2. Advanced data filtering
In addition to basic data filtering methods, Pandas also provides many advanced data filtering techniques, such as using the query method and using index objects. MultiIndex performs multi-level filtering, etc. Below are several case illustrations.
- query method filtering
The query method can filter data through SQL-like syntax. For example, we have a data frame containing student information, and we want to filter out students whose scores are greater than 60 and are between 18 and 20 years old. You can use the following code to achieve this:
import pandas as pd data = { '姓名': ['张三', '李四', '王五', '赵六'], '成绩': [80, 70, 90, 50], '年龄': [18, 19, 20, 21] } df = pd.DataFrame(data) df_filtered = df.query('成绩 > 60 and 18 <= 年龄 <= 20') print(df_filtered)
- Use MultiIndex to filter
If the data frame has multiple levels of indexes, you can use the MultiIndex object for multi-level filtering. For example, we have a data frame containing student information. The index includes two levels: class and student number. We want to filter out students with student numbers 001 and 002 in class 1. This can be achieved using the following code:
import pandas as pd data = { '姓名': ['张三', '李四', '王五', '赵六'], '成绩': [80, 70, 90, 50], } index = pd.MultiIndex.from_tuples([('1班', '001'), ('1班', '002'), ('2班', '001'), ('2班', '002')]) df = pd.DataFrame(data, index=index) df_filtered = df.loc[('1班', ['001', '002']), :] print(df_filtered)
3. Case Analysis
Now we take a real data set as an example to further illustrate the advanced techniques of Pandas data filtering. Suppose we have a car sales data set, which contains information such as vehicle brand, model, sales volume, and sales volume. We want to filter out models with sales of more than 1,000 units and sales of more than 1 million. The following is a code example:
import pandas as pd data = { '品牌': ['宝马', '奥迪', '奔驰', '大众'], '型号': ['X3', 'A6', 'E级', '朗逸'], '销售量': [1200, 800, 1500, 900], '销售额': [1200, 900, 1800, 800] } df = pd.DataFrame(data) df_filtered = df.query('销售量 > 1000 and 销售额 > 1000000') print(df_filtered)
Through the above code, we successfully screened out models with sales of more than 1,000 units and sales of more than 1 million yuan.
To sum up, Pandas provides a wealth of data filtering functions and methods, from basic Boolean index, loc and iloc methods to advanced query methods and MultiIndex filtering, which can meet the data filtering needs in different scenarios. . The above cases demonstrate some common data filtering techniques and applications, and hope to be helpful to readers in practical applications.
The above is the detailed content of Advanced techniques and practical applications of Pandas data filtering. For more information, please follow other related articles on the PHP Chinese website!

Hot AI Tools

Undresser.AI Undress
AI-powered app for creating realistic nude photos

AI Clothes Remover
Online AI tool for removing clothes from photos.

Undress AI Tool
Undress images for free

Clothoff.io
AI clothes remover

AI Hentai Generator
Generate AI Hentai for free.

Hot Article

Hot Tools

Notepad++7.3.1
Easy-to-use and free code editor

SublimeText3 Chinese version
Chinese version, very easy to use

Zend Studio 13.0.1
Powerful PHP integrated development environment

Dreamweaver CS6
Visual web development tools

SublimeText3 Mac version
God-level code editing software (SublimeText3)

Hot Topics


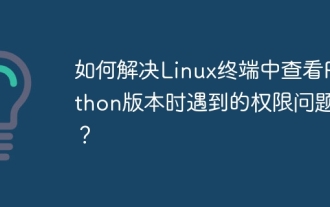
Solution to permission issues when viewing Python version in Linux terminal When you try to view Python version in Linux terminal, enter python...
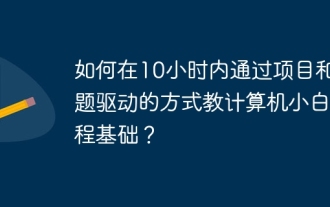
How to teach computer novice programming basics within 10 hours? If you only have 10 hours to teach computer novice some programming knowledge, what would you choose to teach...
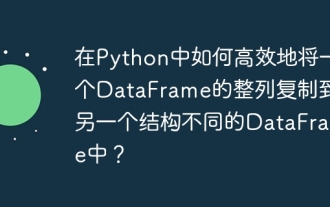
When using Python's pandas library, how to copy whole columns between two DataFrames with different structures is a common problem. Suppose we have two Dats...
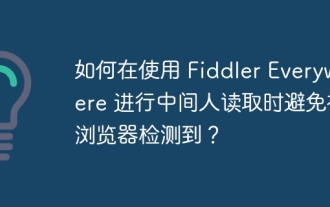
How to avoid being detected when using FiddlerEverywhere for man-in-the-middle readings When you use FiddlerEverywhere...
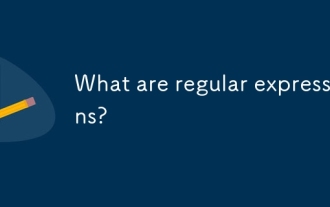
Regular expressions are powerful tools for pattern matching and text manipulation in programming, enhancing efficiency in text processing across various applications.
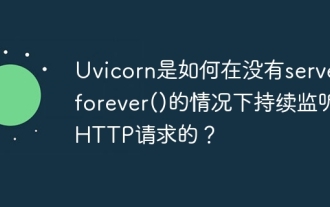
How does Uvicorn continuously listen for HTTP requests? Uvicorn is a lightweight web server based on ASGI. One of its core functions is to listen for HTTP requests and proceed...
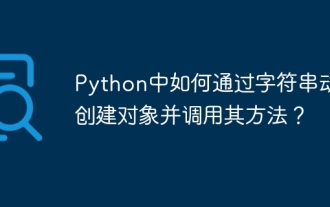
In Python, how to dynamically create an object through a string and call its methods? This is a common programming requirement, especially if it needs to be configured or run...
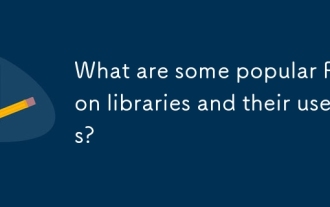
The article discusses popular Python libraries like NumPy, Pandas, Matplotlib, Scikit-learn, TensorFlow, Django, Flask, and Requests, detailing their uses in scientific computing, data analysis, visualization, machine learning, web development, and H
