


Learning cross-modal occupancy knowledge: RadOcc using rendering-assisted distillation technology
Original title: Radocc: Learning Cross-Modality Occupancy Knowledge through Rendering Assisted Distillation
Paper link: https://arxiv.org/pdf/2312.11829.pdf
Author affiliation: FNii , CUHK-Shenzhen SSE, CUHK-Shenzhen Huawei Noah's Ark Laboratory
Conference: AAAI 2024
Paper idea:
3D occupancy prediction is an emerging task that aims to estimate the occupancy status and semantics of 3D scenes using multi-view images. However, image-based scene perception encounters significant challenges in achieving accurate predictions due to the lack of geometric priors. This paper addresses this problem by exploring cross-modal knowledge distillation in this task, i.e., we utilize a more powerful multi-modal model to guide the visual model during the training process. In practice, this paper observes that direct application of feature or logits alignment, proposed and widely used in bird's-eye view (BEV) perception, does not yield satisfactory results. To overcome this problem, this paper introduces RadOcc, a rendering-assisted distillation paradigm for 3D occupancy prediction. By employing differentiable volume rendering, we generate depth and semantic maps in perspective and propose two novel consistency criteria between the rendered output of teacher and student models. Specifically, the depth consistency loss aligns the termination distributions of rendering rays, while the semantic consistency loss mimics the intra-segment similarity guided by the visual base model (VLM). Experimental results on the nuScenes dataset demonstrate the effectiveness of the method proposed in this article in improving various 3D occupancy prediction methods. For example, the method proposed in this article improves the baseline of this article by 2.2% in the mIoU metric and reaches 2.2% in the Occ3D benchmark. 50%.
Main contributions:
This paper introduces a rendering-assisted distillation paradigm called RadOcc for 3D occupancy prediction. This is the first paper exploring cross-modal knowledge distillation in 3D-OP, providing valuable insights into the application of existing BEV distillation techniques in this task.
The authors propose two novel distillation constraints, namely rendering depth and semantic consistency (RDC and RSC). These constraints effectively enhance the knowledge transfer process by aligning light distribution and correlation matrices guided by the vision base model. The key to this approach is to use depth and semantic information to guide the rendering process, thereby improving the quality and accuracy of the rendering results. By combining these two constraints, the researchers achieved significant improvements, providing new solutions for knowledge transfer in vision tasks.
Equipped with the proposed method, RadOcc shows state-of-the-art dense and sparse occupancy prediction performance on Occ3D and nuScenes benchmarks. In addition, experiments have proven that the distillation method proposed in this article can effectively improve the performance of multiple baseline models.
Network design:
This paper is the first to study cross-modal knowledge distillation for the 3D occupancy prediction task. Based on the method of knowledge transfer using BEV or logits consistency in the BEV sensing field, this paper extends these distillation techniques to the 3D occupancy prediction task, aiming to align voxel features and voxel logits, as shown in Figure 1(a) . However, preliminary experiments show that these alignment techniques face significant challenges in 3D-OP tasks, especially the former method that introduces negative transfer. This challenge may stem from the fundamental difference between 3D object detection and occupancy prediction, which as a more fine-grained perception task requires capturing geometric details as well as background objects.
To address the above challenges, this paper proposes RadOcc, a novel method for cross-modal knowledge distillation using differentiable volume rendering. The core idea of RadOcc is to align the rendering results generated by the teacher model and the student model, as shown in Figure 1(b). Specifically, this article uses the intrinsic and extrinsic parameters of the camera to perform volume rendering of voxel features (Mildenhall et al. 2021), which enables this article to obtain corresponding depth maps and semantic maps from different viewpoints. To achieve better alignment between rendered outputs, this paper introduces novel Rendering Depth Consistency (RDC) and Rendering Semantic Consistency (RSC) losses. On the one hand, RDC loss enforces the consistency of ray distribution, which enables the student model to capture the underlying structure of the data. On the other hand, the RSC loss takes advantage of the visual base model (Kirillov et al. 2023) and utilizes pre-extracted segments for affinity distillation. This standard allows models to learn and compare semantic representations of different image regions, thereby enhancing their ability to capture fine-grained details. By combining the above constraints, the method proposed in this paper effectively leverages cross-modal knowledge distillation, thereby improving performance and better optimizing the student model. This paper demonstrates the effectiveness of our approach on dense and sparse occupancy prediction, achieving state-of-the-art results on both tasks.
Figure 1: Render-assisted distillation. (a) Existing methods align features or logits. (b) The RadOcc method proposed in this paper simultaneously constrains the rendered depth map and semantics. Figure 2: Overall framework of RadOcc. It adopts a teacher-student architecture, where the teacher network is a multi-modal model and the student network only accepts camera input. The predictions of both networks will be used to generate rendering depth and semantics through differentiable volume rendering. Newly proposed rendering depth and semantic consistency losses are adopted between rendering results.
Figure 3: Rendering depth analysis. Although the teacher (T) and student (S) have similar rendering depths, especially for foreground objects, their light termination distributions show large differences.
Figure 4: Generation of affinity matrix. This article first uses the Vision Foundation Model (VFM), namely SAM, to extract segments into the original image. Afterwards, this article performs segment aggregation on the semantic features rendered in each segment to obtain the affinity matrix.
Experimental results:
##Summary:
This paper proposes RadOcc, a new cross-modal approach for 3D occupancy prediction Knowledge distillation paradigm. It utilizes a multimodal teacher model to provide geometric and semantic guidance to the visual student model through differentiable volume rendering. Furthermore, this paper proposes two new consistency criteria, depth consistency loss and semantic consistency loss, to align the ray distribution and affinity matrix between teacher and student models. Extensive experiments on Occ3D and nuScenes datasets show that RadOcc can significantly improve the performance of various 3D occupancy prediction methods. Our method achieves state-of-the-art results on the Occ3D challenge benchmark and significantly outperforms existing published methods. We believe that our work opens up new possibilities for cross-modal learning in scene understanding.The above is the detailed content of Learning cross-modal occupancy knowledge: RadOcc using rendering-assisted distillation technology. For more information, please follow other related articles on the PHP Chinese website!

Hot AI Tools

Undresser.AI Undress
AI-powered app for creating realistic nude photos

AI Clothes Remover
Online AI tool for removing clothes from photos.

Undress AI Tool
Undress images for free

Clothoff.io
AI clothes remover

AI Hentai Generator
Generate AI Hentai for free.

Hot Article

Hot Tools

Notepad++7.3.1
Easy-to-use and free code editor

SublimeText3 Chinese version
Chinese version, very easy to use

Zend Studio 13.0.1
Powerful PHP integrated development environment

Dreamweaver CS6
Visual web development tools

SublimeText3 Mac version
God-level code editing software (SublimeText3)

Hot Topics


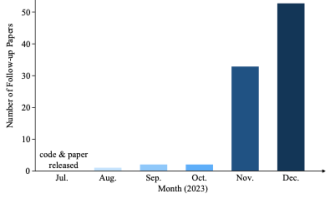
Written above & the author’s personal understanding Three-dimensional Gaussiansplatting (3DGS) is a transformative technology that has emerged in the fields of explicit radiation fields and computer graphics in recent years. This innovative method is characterized by the use of millions of 3D Gaussians, which is very different from the neural radiation field (NeRF) method, which mainly uses an implicit coordinate-based model to map spatial coordinates to pixel values. With its explicit scene representation and differentiable rendering algorithms, 3DGS not only guarantees real-time rendering capabilities, but also introduces an unprecedented level of control and scene editing. This positions 3DGS as a potential game-changer for next-generation 3D reconstruction and representation. To this end, we provide a systematic overview of the latest developments and concerns in the field of 3DGS for the first time.
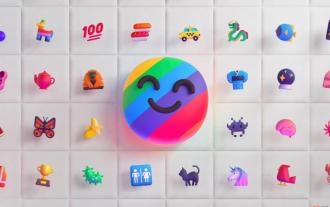
You must remember, especially if you are a Teams user, that Microsoft added a new batch of 3DFluent emojis to its work-focused video conferencing app. After Microsoft announced 3D emojis for Teams and Windows last year, the process has actually seen more than 1,800 existing emojis updated for the platform. This big idea and the launch of the 3DFluent emoji update for Teams was first promoted via an official blog post. Latest Teams update brings FluentEmojis to the app Microsoft says the updated 1,800 emojis will be available to us every day
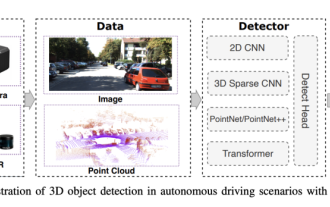
0.Written in front&& Personal understanding that autonomous driving systems rely on advanced perception, decision-making and control technologies, by using various sensors (such as cameras, lidar, radar, etc.) to perceive the surrounding environment, and using algorithms and models for real-time analysis and decision-making. This enables vehicles to recognize road signs, detect and track other vehicles, predict pedestrian behavior, etc., thereby safely operating and adapting to complex traffic environments. This technology is currently attracting widespread attention and is considered an important development area in the future of transportation. one. But what makes autonomous driving difficult is figuring out how to make the car understand what's going on around it. This requires that the three-dimensional object detection algorithm in the autonomous driving system can accurately perceive and describe objects in the surrounding environment, including their locations,
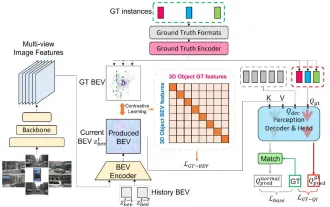
Written above & the author’s personal understanding: At present, in the entire autonomous driving system, the perception module plays a vital role. The autonomous vehicle driving on the road can only obtain accurate perception results through the perception module. The downstream regulation and control module in the autonomous driving system makes timely and correct judgments and behavioral decisions. Currently, cars with autonomous driving functions are usually equipped with a variety of data information sensors including surround-view camera sensors, lidar sensors, and millimeter-wave radar sensors to collect information in different modalities to achieve accurate perception tasks. The BEV perception algorithm based on pure vision is favored by the industry because of its low hardware cost and easy deployment, and its output results can be easily applied to various downstream tasks.
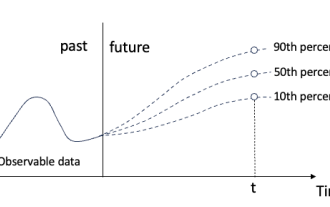
Do not change the meaning of the original content, fine-tune the content, rewrite the content, and do not continue. "Quantile regression meets this need, providing prediction intervals with quantified chances. It is a statistical technique used to model the relationship between a predictor variable and a response variable, especially when the conditional distribution of the response variable is of interest When. Unlike traditional regression methods, quantile regression focuses on estimating the conditional magnitude of the response variable rather than the conditional mean. "Figure (A): Quantile regression Quantile regression is an estimate. A modeling method for the linear relationship between a set of regressors X and the quantiles of the explained variables Y. The existing regression model is actually a method to study the relationship between the explained variable and the explanatory variable. They focus on the relationship between explanatory variables and explained variables

Original title: SIMPL: ASimpleandEfficientMulti-agentMotionPredictionBaselineforAutonomousDriving Paper link: https://arxiv.org/pdf/2402.02519.pdf Code link: https://github.com/HKUST-Aerial-Robotics/SIMPL Author unit: Hong Kong University of Science and Technology DJI Paper idea: This paper proposes a simple and efficient motion prediction baseline (SIMPL) for autonomous vehicles. Compared with traditional agent-cent
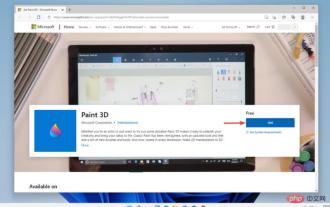
When the gossip started spreading that the new Windows 11 was in development, every Microsoft user was curious about how the new operating system would look like and what it would bring. After speculation, Windows 11 is here. The operating system comes with new design and functional changes. In addition to some additions, it comes with feature deprecations and removals. One of the features that doesn't exist in Windows 11 is Paint3D. While it still offers classic Paint, which is good for drawers, doodlers, and doodlers, it abandons Paint3D, which offers extra features ideal for 3D creators. If you are looking for some extra features, we recommend Autodesk Maya as the best 3D design software. like
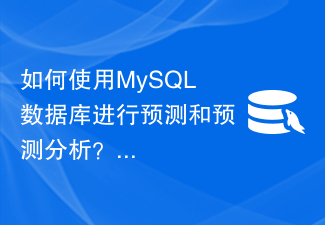
How to use MySQL database for forecasting and predictive analytics? Overview: Forecasting and predictive analytics play an important role in data analysis. MySQL, a widely used relational database management system, can also be used for prediction and predictive analysis tasks. This article will introduce how to use MySQL for prediction and predictive analysis, and provide relevant code examples. Data preparation: First, we need to prepare relevant data. Suppose we want to do sales forecasting, we need a table with sales data. In MySQL we can use
