


LLaVA-1.6, which catches up with Gemini Pro and improves reasoning and OCR capabilities, is too powerful
In April last year, researchers from the University of Wisconsin-Madison, Microsoft Research, and Columbia University jointly released LLaVA (Large Language and Vision Assistant). Although LLaVA is only trained with a small multi-modal instruction data set, it shows very similar inference results to GPT-4 on some samples. Then in October, they launched LLaVA-1.5, which refreshed the SOTA in 11 benchmarks with simple modifications to the original LLaVA. The results of this upgrade are very exciting, bringing new breakthroughs to the field of multi-modal AI assistants.
The research team announced the launch of LLaVA-1.6 version, which has made major performance improvements in reasoning, OCR and world knowledge. This version of LLaVA-1.6 even outperforms the Gemini Pro in multiple benchmarks.
- ##demo address: https://llava.hliu.cc/
- Project address: https://github.com/haotian-liu/LLaVA
Compared with LLaVA-1.5, LLaVA-1.6 has the following improvements:
- Increases the input image resolution by 4 times, supports three aspect ratios, up to Up to 672x672, 336x1344, 1344x336 resolution. This enables LLaVA-1.6 to capture more visual details.
- LLaVA-1.6 gains better visual reasoning and OCR capabilities through improved visual instructions to adjust data mixing.
- Better visual dialogue, more scenarios, covering different applications. LLaVA-1.6 has mastered more world knowledge and has better logical reasoning ability.
- Use SGLang for efficient deployment and inference.
LLaVA-1.6 is fine-tuned and optimized based on LLaVA-1.5. It retains the simple design and efficient data processing capabilities of LLaVA-1.5, and continues to use less than 1M visual instruction tuning samples. By using 32 A100 graphics cards, the largest 34B model was trained in approximately 1 day. In addition, LLaVA-1.6 utilizes 1.3 million data samples, and its calculation/training data cost is only 100-1000 times that of other methods. These improvements make LLaVA-1.6 a more efficient and cost-effective version.
Method Improvement
Dynamic High Resolution
Research Team The LLaVA-1.6 model was designed at high resolution to maintain its data efficiency. When provided with high-resolution images and detail-preserving representations, the model's ability to perceive complex details in images improves significantly. It reduces model hallucination when faced with low-resolution images, i.e. guessing the imagined visual content.
Data Mixing
High quality user command data. The study’s definition of high-quality visual instruction following data depends on two main criteria: first, the diversity of task instructions, ensuring that the broad range of user intentions that may be encountered in real-life scenarios is adequately represented, especially It is during the model deployment phase. Second, prioritization of responses is critical, aiming to solicit favorable user feedback.
Therefore, the study considered two data sources:Existing GPT-V data (LAION-GPT-V and ShareGPT -4V);
In order to further promote better visual dialogue in more scenarios, the research team collected a small 15K visual instruction tuning data set covering different applications, carefully filtering samples that may have privacy issues or may be harmful, And use GPT-4V to generate the response. Multimodal document/chart data. (1) Remove TextCap from the training data because the research team realized that TextCap uses the same training image set as TextVQA. This allowed the research team to better understand the model's zero-shot OCR capabilities when evaluating TextVQA. In order to maintain and further improve the OCR capabilities of the model, this study replaced TextCap with DocVQA and SynDog-EN. (2) With Qwen-VL-7B-Chat, this study further adds ChartQA, DVQA, and AI2D for better understanding of plots and charts. The research team also stated that in addition to Vicuna-1.5 (7B and 13B), it is also considering using more LLM solutions, including Mistral-7B and Nous-Hermes-2-Yi-34B, to Enable LLaVA to support a wider range of users and more scenarios.
The above is the detailed content of LLaVA-1.6, which catches up with Gemini Pro and improves reasoning and OCR capabilities, is too powerful. For more information, please follow other related articles on the PHP Chinese website!

Hot AI Tools

Undresser.AI Undress
AI-powered app for creating realistic nude photos

AI Clothes Remover
Online AI tool for removing clothes from photos.

Undress AI Tool
Undress images for free

Clothoff.io
AI clothes remover

AI Hentai Generator
Generate AI Hentai for free.

Hot Article

Hot Tools

Notepad++7.3.1
Easy-to-use and free code editor

SublimeText3 Chinese version
Chinese version, very easy to use

Zend Studio 13.0.1
Powerful PHP integrated development environment

Dreamweaver CS6
Visual web development tools

SublimeText3 Mac version
God-level code editing software (SublimeText3)

Hot Topics


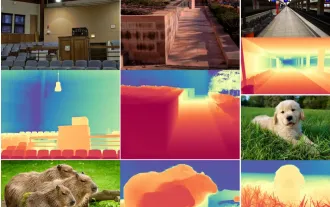
0.What does this article do? We propose DepthFM: a versatile and fast state-of-the-art generative monocular depth estimation model. In addition to traditional depth estimation tasks, DepthFM also demonstrates state-of-the-art capabilities in downstream tasks such as depth inpainting. DepthFM is efficient and can synthesize depth maps within a few inference steps. Let’s read about this work together ~ 1. Paper information title: DepthFM: FastMonocularDepthEstimationwithFlowMatching Author: MingGui, JohannesS.Fischer, UlrichPrestel, PingchuanMa, Dmytr
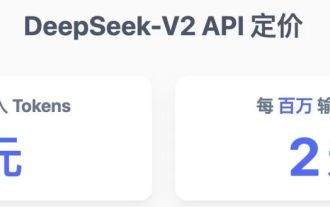
Imagine an artificial intelligence model that not only has the ability to surpass traditional computing, but also achieves more efficient performance at a lower cost. This is not science fiction, DeepSeek-V2[1], the world’s most powerful open source MoE model is here. DeepSeek-V2 is a powerful mixture of experts (MoE) language model with the characteristics of economical training and efficient inference. It consists of 236B parameters, 21B of which are used to activate each marker. Compared with DeepSeek67B, DeepSeek-V2 has stronger performance, while saving 42.5% of training costs, reducing KV cache by 93.3%, and increasing the maximum generation throughput to 5.76 times. DeepSeek is a company exploring general artificial intelligence
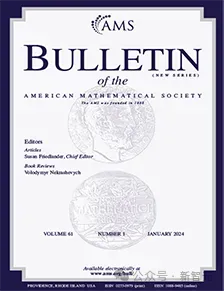
AI is indeed changing mathematics. Recently, Tao Zhexuan, who has been paying close attention to this issue, forwarded the latest issue of "Bulletin of the American Mathematical Society" (Bulletin of the American Mathematical Society). Focusing on the topic "Will machines change mathematics?", many mathematicians expressed their opinions. The whole process was full of sparks, hardcore and exciting. The author has a strong lineup, including Fields Medal winner Akshay Venkatesh, Chinese mathematician Zheng Lejun, NYU computer scientist Ernest Davis and many other well-known scholars in the industry. The world of AI has changed dramatically. You know, many of these articles were submitted a year ago.
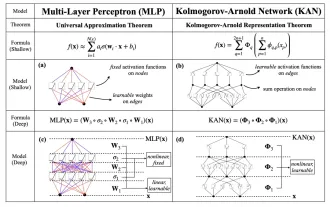
Earlier this month, researchers from MIT and other institutions proposed a very promising alternative to MLP - KAN. KAN outperforms MLP in terms of accuracy and interpretability. And it can outperform MLP running with a larger number of parameters with a very small number of parameters. For example, the authors stated that they used KAN to reproduce DeepMind's results with a smaller network and a higher degree of automation. Specifically, DeepMind's MLP has about 300,000 parameters, while KAN only has about 200 parameters. KAN has a strong mathematical foundation like MLP. MLP is based on the universal approximation theorem, while KAN is based on the Kolmogorov-Arnold representation theorem. As shown in the figure below, KAN has
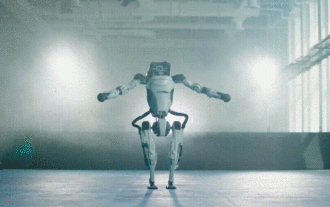
Boston Dynamics Atlas officially enters the era of electric robots! Yesterday, the hydraulic Atlas just "tearfully" withdrew from the stage of history. Today, Boston Dynamics announced that the electric Atlas is on the job. It seems that in the field of commercial humanoid robots, Boston Dynamics is determined to compete with Tesla. After the new video was released, it had already been viewed by more than one million people in just ten hours. The old people leave and new roles appear. This is a historical necessity. There is no doubt that this year is the explosive year of humanoid robots. Netizens commented: The advancement of robots has made this year's opening ceremony look like a human, and the degree of freedom is far greater than that of humans. But is this really not a horror movie? At the beginning of the video, Atlas is lying calmly on the ground, seemingly on his back. What follows is jaw-dropping
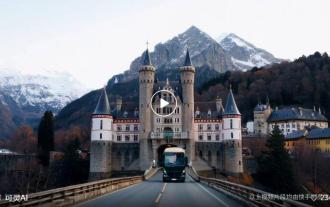
What? Is Zootopia brought into reality by domestic AI? Exposed together with the video is a new large-scale domestic video generation model called "Keling". Sora uses a similar technical route and combines a number of self-developed technological innovations to produce videos that not only have large and reasonable movements, but also simulate the characteristics of the physical world and have strong conceptual combination capabilities and imagination. According to the data, Keling supports the generation of ultra-long videos of up to 2 minutes at 30fps, with resolutions up to 1080p, and supports multiple aspect ratios. Another important point is that Keling is not a demo or video result demonstration released by the laboratory, but a product-level application launched by Kuaishou, a leading player in the short video field. Moreover, the main focus is to be pragmatic, not to write blank checks, and to go online as soon as it is released. The large model of Ke Ling is already available in Kuaiying.
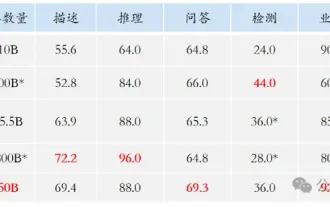
I cry to death. The world is madly building big models. The data on the Internet is not enough. It is not enough at all. The training model looks like "The Hunger Games", and AI researchers around the world are worrying about how to feed these data voracious eaters. This problem is particularly prominent in multi-modal tasks. At a time when nothing could be done, a start-up team from the Department of Renmin University of China used its own new model to become the first in China to make "model-generated data feed itself" a reality. Moreover, it is a two-pronged approach on the understanding side and the generation side. Both sides can generate high-quality, multi-modal new data and provide data feedback to the model itself. What is a model? Awaker 1.0, a large multi-modal model that just appeared on the Zhongguancun Forum. Who is the team? Sophon engine. Founded by Gao Yizhao, a doctoral student at Renmin University’s Hillhouse School of Artificial Intelligence.
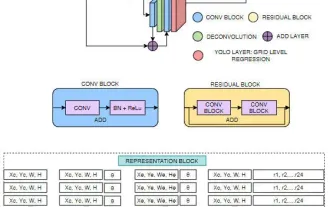
Target detection is a relatively mature problem in autonomous driving systems, among which pedestrian detection is one of the earliest algorithms to be deployed. Very comprehensive research has been carried out in most papers. However, distance perception using fisheye cameras for surround view is relatively less studied. Due to large radial distortion, standard bounding box representation is difficult to implement in fisheye cameras. To alleviate the above description, we explore extended bounding box, ellipse, and general polygon designs into polar/angular representations and define an instance segmentation mIOU metric to analyze these representations. The proposed model fisheyeDetNet with polygonal shape outperforms other models and simultaneously achieves 49.5% mAP on the Valeo fisheye camera dataset for autonomous driving
