Causal inference practice in Kuaishou short video recommendation
1. Kuaishou single column short video recommendation scenario
1. About Kuaishou
*Data is taken from the second quarter of 2023
Kuaishou is a popular short video and live broadcast community application. In the second quarter of this year, we achieved impressive new records for MAU and DAU. The core concept of Kuaishou is to enable everyone to become a content creator and disseminator by observing and sharing the lives of ordinary people. In Kuaishou applications, short video scenes are mainly divided into two forms: single column and double column. At present, the traffic of a single column is relatively large, and users can immersively browse video content by sliding up and down. The double-column presentation is similar to an information flow. Users need to select the ones they are interested in from the several contents appearing on the screen and click to watch. The recommendation algorithm is the core of Kuaishou's business ecosystem and plays an important role in traffic distribution and user experience improvement. By analyzing users' interests and behavioral data, Kuaishou can accurately push content that matches users' tastes, thereby improving user stickiness and satisfaction. In general, Kuaishou, as a national-level short video and live broadcast community application, continues to attract more users to join it with its unique concept of observing and sharing ordinary people's lives, as well as its excellent recommendation algorithm, and among users Remarkable results have been achieved in terms of experience and traffic distribution.
2. Kuaishou single column short video recommendation scenario
In the Kuaishou short video recommendation scenario, single column as the main form. Users browse videos through the behavior mode of sliding up and down. Once the video is slid, it will automatically play without the user having to select and then click to trigger playback. In addition, there are many forms of user feedback, including following, likes, comment sharing, progress bar dragging, etc. With the development of business, interactive forms are becoming increasingly diversified. Optimization goals include long-term goals and short-term goals. Long-term goals include optimizing user experience and retaining DAU, while short-term indicators cover various positive feedback from users.
The recommendation system is based on machine learning and deep learning. The logs mainly come from the characteristics and feedback generated by the user's actual behavior. However, logs have limitations and can only reflect limited information about the user's current interests, and private information such as real name, height and weight cannot be obtained. At the same time, the recommendation algorithm is based on previous log learning and training, and then recommends it to users, which has the characteristics of self-loop. In addition, due to the wide and varied audience, large number of videos and frequent updates, the recommendation system is prone to various biases, such as popularity bias, long and short video exposure bias, etc. In short video recommendation, bias modeling using causal inference technology can help correct bias and improve recommendation effects.
2. Causal inference technology and model representation
Next, we will share our experience with our brother team in causal inference and model representation. Work.
1. Background
Recommended systems usually perform model learning by analyzing interaction logs . User feedback not only comes from preference for content, but also is affected by herd mentality. Taking movie selection as an example, users may consider the award-winning status of the work or the opinions of people around them when making decisions. There are differences in the herd mentality among different users. Some users are more subjective and independent, while some users are more susceptible to the influence of others or popularity. Therefore, when attributing user interactions, in addition to considering the user's interests, it is also necessary to consider the factors of herd psychology.
Most existing work treats popularity as a static deviation. For example, the popularity of a movie is only related to an item, and the deviation between users is not considered when modeling user and item ratings. Popularity is usually used as a separate scoring item, which is related to the number of exposures of the item, and there is less bias for items with lower popularity. This way of modeling is static and item-relative. With the application of causal inference technology in the field of recommendation, some research attempts to deal with this problem through decoupled representation and consider the difference in herd psychology when users select items. Compared with existing methods, our method can more accurately model the differences in users' herd mentality, thereby more effectively correcting deviations and improving recommendation effects.
2. Related work
In a paper in Webconf2021, user interaction is modeled as both the user’s response to the item and Affected by the interest of the item, it is also affected by the degree of herd mentality of the user when choosing the item. The causal relationship diagram is shown on the left, and the relationship is relatively simple. In specific modeling, the representations of user and item are split into interest representation and conformity representation. For interest expression, an interest loss is constructed; for conformity expression, a confirmation loss is constructed; for feedback behavior, a click loss is constructed. Due to the splitting of the representation structure, interest loss is used as a supervision signal to learn the interest representation, while confirmation loss is used to model the herd mentality representation. Click loss is related to two factors and is therefore constructed by concatenation and intersection. The entire approach is clear and simple.
When constructing interest loss and confirmation loss, this research also uses some concepts and techniques in causal inference. For example, if an unpopular video or item gets positive interactions, it's likely because users actually liked it. This can be confirmed through reverse verification: if an item is not popular and users are not interested in it, there is unlikely to be a positive interaction. As for click loss, a common processing method is adopted, namely pairwise loss. Regarding the colliding effect, interested readers can refer to the paper for a more detailed construction method.
When solving complex problems in recommendation systems, some research starts from model representation, aiming to distinguish users' interest in items and herd mentality. . However, there are some problems in practical application. There are a huge number of videos in the recommendation system, and the exposures are unevenly distributed. Head videos have more exposures and long-tail videos have less exposures, resulting in sparse data. Sparsity brings learning difficulties to machine learning models.
To solve this problem, we introduced contrastive learning for sample augmentation. Specifically, in addition to the positive interaction between the user and the item, we also selected other videos within the user's behavior range as negative samples for expansion. At the same time, we used the cause-and-effect diagram to design the model and split the interest and conformity representations on the user and item sides. The main difference between this model and traditional DICE is that it adopts the method of contrastive learning and sample augmentation when learning the loss of interest and confirmation, and constructs the normalized ratio index term of item popularity for interest loss and confirmation loss respectively. In this way, the data sparsity problem can be better handled and users' interest and herd mentality for items of different popularity can be more accurately modeled.
3. Summary
This work is based on the superposition of interest and herd psychology in short video interaction , using causal inference technology and causal embedding methods to achieve decoupling of structural representation. At the same time, considering the sparse problem of long-tail video samples in the actual system, contrastive learning and sample augmentation methods are used to alleviate the sparsity. This work combines online representation models and causal inference to achieve a certain conformity decoupling effect. This method performed well in offline and online experiments, and was successfully used in Kuaishou recommendation LTR experiments, bringing certain effect improvements.
3. Viewing duration estimation and causal inference technology
1. Importance of viewing duration
In the short video recommendation business, viewing time is an important optimization goal, which is closely related to long-term indicators such as user retention, DAU and return visit rate. In order to improve user experience, we need to focus on intermediate behavioral indicators when recommending videos to users. Experience shows that watch time is a very valuable metric because users have limited attention spans. By observing changes in users’ viewing time, we can better understand what factors affect users’ viewing experience.
Video length is one of the important factors that affects the viewing time. As the length of the video increases, the user's viewing time will also increase accordingly, but a video that is too long may lead to diminishing marginal effects, or even a slight decrease in viewing time. Therefore, the recommendation system needs to find a balance point to recommend a video length that suits the user's needs.
In order to optimize the viewing time, the recommendation system needs to predict the user's viewing time. This involves a regression problem because duration is a continuous value. However, there is less work related to duration, probably because the short video recommendation business is relatively new, while recommendation system research has a long history.
When solving the problem of viewing duration estimation, factors other than video length can be considered, such as user interest, video content quality, etc. By taking these factors into consideration, the accuracy of predictions is improved and users are provided with a better recommendation experience. At the same time, we also need to continuously iterate and optimize the recommendation algorithm to adapt to changes in the market and user needs.
2, D2Q
At the KDD212 conference, we proposed a solution to short video recommendation A new approach to the medium-duration forecasting problem. This problem mainly stems from the self-reinforcing phenomenon of duration bias in causal inference. To address this issue, we introduce a cause-and-effect diagram to describe the relationship between users, videos, and viewing duration.
In the causal diagram, U and V represent the feature representation of the user and the video respectively, W represents the length of time the user watches the video, and D represents the length of the video. We found that due to the self-cyclic generation process of the recommendation system, duration is not only directly related to the viewing duration, but also affects the learning of video representation.
In order to eliminate the impact of duration on video representation, we used do calculus for derivation. The final conclusion shows that in order to solve this problem through backdoor adjustment, the simplest and most direct method is to separately estimate the viewing duration for the samples corresponding to each duration video. This can eliminate the amplification effect of duration on viewing duration, thereby effectively solving the problem of duration bias in causal inference. The core idea of this method is to eliminate the error from d to v, thereby mitigating bias amplification.
When solving the problem of duration estimation in short video recommendation, we adopt a method based on causal inference to eliminate the error from d to v And achieve relief from bias amplification. In order to deal with the problem of duration as a continuous variable and the distribution of the number of videos, we group the videos in the recommendation pool according to duration and use quantiles for calculation. The data within each group is split and used to train the model within the group. During the training process, the quantile corresponding to the video duration in each duration group is regressed instead of directly regressing the duration. This reduces data sparsity and avoids model overfitting. During online inference, for each video, first find its corresponding group, and then calculate the corresponding duration quantile. By looking up the table, you can find the actual viewing time based on the quantiles. This method simplifies the online reasoning process and improves the accuracy of duration estimation. In summary, our method effectively solves the duration estimation problem in short video recommendation by eliminating the error from d to v, and provides strong support for optimizing user experience.
When solving the problem of duration estimation in short video recommendation, we also introduced a parameter sharing method to reduce technical difficulty. In the split training process, an ideal approach is to achieve complete separation of data, features, and models, but this will increase deployment costs. Therefore, we chose a simpler way, which is to share the embedding of the underlying features and the model parameters of the middle layer, and only split it in the output layer. In order to further expand the impact of duration on the actual viewing duration, we introduce a residual connection to directly connect duration to the part that outputs the quantile of the estimated duration, thereby enhancing the influence of duration. This method reduces the technical difficulty and effectively solves the problem of duration estimation in short video recommendation.
In the experiment, the public data set released by Kuaishou was mainly used. By comparing several methods, we can see that the performance of direct regression and duration-weighted models have their own advantages. The duration-weighted model is no stranger to recommendation systems. Its core idea is to include viewing duration as the weight of positive samples into the model. D2Q and Res-D2Q are two model structures based on causal inference, among which Res-D2Q introduces residual connections. Through experiments, we found that the best results can be achieved when videos are grouped into 30 groups according to duration. Compared with the naive regression model, the D2Q method has significant improvements and can alleviate the self-loop amplification problem of duration bias to a certain extent. However, from the perspective of the duration estimation problem, the challenge is still not fully resolved.
3. TPM
As a core issue in the recommendation system, the duration estimation problem has its own Unique features and challenges. First, the regression model cannot reflect the sequential relationship of the recommendation results, so even when the regression error is the same, the actual recommendation results may be very different. In addition, in addition to ensuring the accuracy of the estimate, the prediction model also needs to consider the confidence of the estimate given by the model. A trustworthy model should not only give accurate estimates, but also give that estimate with a high probability. Therefore, when solving the problem of duration estimation, we must not only pay attention to the accuracy of the regression, but also consider the confidence of the model and the order relationship of the estimated values.
In the viewing behavior, there is a conditional dependency relationship between the user's continuous viewing of videos. Specifically, if watching the entire video is a random event, then watching 50% of the video first is also a random event, and there is a strict conditional dependence between them. Solving the bias amplification problem is very important in viewing time estimation, and the D2Q method solves this problem well. In contrast, our proposed TPM approach aims to comprehensively cover all duration estimation problems.
The main idea of the TPM method is to transform the duration estimation problem into a discrete search problem. By constructing a complete binary tree, the duration estimation problem is transformed into several classification problems that are conditionally dependent on each other, and then a binary classifier is used to solve these classification problems. By continuously performing a binary search downwards, the probability of viewing duration in each ordered interval is determined, and finally a multinomial distribution of viewing duration is formed. This method can effectively solve the uncertainty modeling problem, making the mean of the estimated duration as close as possible to the true value, while reducing the variance of the estimated duration. The entire viewing time problem or estimation process can be gradually solved by continuously solving interdependent binary classification problems. This method provides a new idea and framework for solving the duration estimation problem, which can improve the accuracy and confidence of the estimation.
When introducing the key duration ideas for modeling TPM, the seamless connection between TPM and D2Q’s backdoor adjustment was demonstrated. Here, a simple cause-and-effect diagram is used to associate user- and item-side features with confounding factors. In order to implement backdoor adjustment in TPM, it is necessary to build a corresponding model for each sample with a confounding factor value, and build a corresponding TPM tree for each confounding factor. Once these two steps are completed, the TPM can be seamlessly connected to the rear door adjustment. This type of connection allows the model to better handle confounding factors, improving prediction accuracy and confidence.
The specific solution is to build a corresponding model for each deep-level confounding factor. Like D2Q, this will also bring about the problems of data sparseness and too many model parameters, which requires share-bottom. Processing, integrating the samples of each confounding factor into the same model, but the underlying embedding representation, intermediate parameters, etc. of the model are all shared, only the output layer part is related to the actual node and interference factor values. During training, you only need to find the real leaf node corresponding to each training sample for training. When estimating, since we don’t know which leaf node the viewing duration belongs to, we need to traverse from top to bottom, and weight the sum of the probability of each leaf node where the viewing duration is located and the expected duration of the corresponding leaf node to get Actual viewing time. This processing method allows the model to better handle confounding factors and improves prediction accuracy and confidence.
We conducted experiments on the Kuaishou public data set and the CIKM16 data set on length of stay. Methods such as WLR, D2Q and OR were compared, and the results showed that TPM has significant advantages. Each module has its specific role, and we also conducted default experiments. The experimental results show that each module plays a role. We also experimented with TPM online. The experimental conditions were to divide Kuaishou's selected traffic evenly into ten groups, and 20% of the traffic was used as the baseline for comparison with the online experimental group. Experimental results show that TPM can significantly increase users' viewing time in the sorting stage, while other indicators remain basically the same. It is worth noting that negative indicators such as the number of user shortwaves have also declined, which we believe has a certain relationship with the accuracy of duration estimates and the reduction in uncertainty in estimates. Watching duration is the core indicator of the short video recommendation platform, and the introduction of TPM is of great significance for improving user experience and platform indicators.
Summarize this part of the introduction. In short video recommendation platforms, viewing time is the core indicator. There are two levels to consider in solving this problem: one is the bias problem, including duration bias and popularity bias, which needs to be solved in the self-loop of the entire system link log to training; the other is the duration estimation problem, which itself is a continuous Value prediction problems usually correspond to regression problems. However, for special duration estimation regression problems, specific methods need to be used. First of all, the bias problem can be alleviated through backdoor adjustment. The specific method is to group duration and design a corresponding model for each group for regression. Secondly, to deal with the regression problem of duration estimation, a tree structure can be used to decompose the duration estimation into several hierarchical ordered intervals. Through the tree traversal process, the problem can be disassembled along the path from the top to the leaf nodes. and resolve. When estimating, the duration is estimated through tree traversal. This processing method can more effectively solve the regression problem of duration estimation and improve prediction accuracy and confidence.
4. Future Outlook
With the acceleration of technological development, the world we live in has changed. It's getting more and more complicated. In Kuaishou’s short video recommendation scenario, the complexity of the recommendation system has become increasingly prominent. In order to make better recommendations, we need to deeply study the application of causal inference in recommendation systems. First, we need to define a problem with business value, such as watch time estimation. We can then understand and model this problem from a causal inference perspective. Through the method of causal adjustment or causal inference, we can better analyze and solve bias problems, such as duration bias and popularity bias. In addition, we can also use technical means, such as machine learning and operations optimization, to solve problems such as system complexity and scene distribution. In order to achieve efficient solutions, we need to find a systematic and automated way to solve the problem. This not only improves work efficiency, but also brings ongoing value to the business. Finally, we need to focus on the scalability and cost-effectiveness of the technology to ensure the feasibility and sustainability of the solution.
In summary, the application of causal inference in recommendation systems is a challenging and potential research direction. Through continuous exploration and practice, we can continuously improve the effectiveness of the recommendation system, bring a better experience to users, and create greater value for the business.
The above is the content shared this time, thank you all.
5. Q&A session
Q1: Compared with D2Q, TPM has made some improvements during its return to make better use of time. dependencies. I would like to ask what the dependency relationship here refers to?
A1: Going from the head node to the leaf node can be regarded as a continuous decision-making process similar to MDP. Conditional dependence means that the decision of the next layer is based on the results of the previous layer. For example, in order to reach the leaf node, which is the interval [0,1], you must first pass through the intermediate node, which is the interval [0,2]. This dependency is realized in actual online estimation by each classifier that only solves whether a specific node should go to the next leaf node. It's like in the age-guessing example, first asking if the age is older than 50, and then depending on the answer, asking if it's older than 25. There is a conditional dependence implicit here, that is, being younger than 50 years old is a prerequisite for answering the second question.
Q2: Will using the tree model bring difficulties to the cost of model training and online inference?
A2: In the comparison of the advantages of TPM and D2Q, the main advantage lies in the splitting of problems. TPM makes better use of temporal information and splits the problem into several binary classification problems with relatively balanced samples, which contributes to the learnability of model training and learning. In contrast, regression problems may be affected by outliers and other outliers, causing greater learning instability. In practical applications, we have carried out a lot of practical work, including sample construction and calculation of TF graph node labels. When deployed online, we use a model, but its output dimension is the number of intermediate node classifiers. For each video, we select only one of the duration groups and calculate the output of the corresponding classifier. Then the distribution on the leaf nodes is calculated through a loop, and finally a weighted sum is performed. Although the model structure is relatively simple, the classifiers of each duration group and each non-leaf node can share the underlying embedding and intermediate layers, so during forward inference, except for the output layer, it is not much different from the ordinary model.
The above is the detailed content of Causal inference practice in Kuaishou short video recommendation. For more information, please follow other related articles on the PHP Chinese website!

Hot AI Tools

Undresser.AI Undress
AI-powered app for creating realistic nude photos

AI Clothes Remover
Online AI tool for removing clothes from photos.

Undress AI Tool
Undress images for free

Clothoff.io
AI clothes remover

AI Hentai Generator
Generate AI Hentai for free.

Hot Article

Hot Tools

Notepad++7.3.1
Easy-to-use and free code editor

SublimeText3 Chinese version
Chinese version, very easy to use

Zend Studio 13.0.1
Powerful PHP integrated development environment

Dreamweaver CS6
Visual web development tools

SublimeText3 Mac version
God-level code editing software (SublimeText3)

Hot Topics


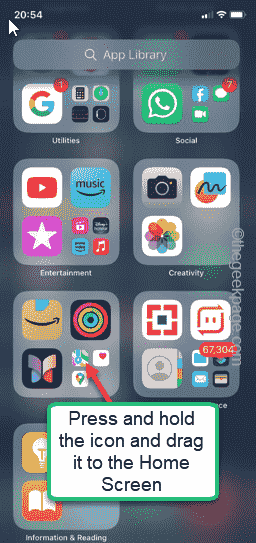
Deleted something important from your home screen and trying to get it back? You can put app icons back on the screen in a variety of ways. We have discussed all the methods you can follow and put the app icon back on the home screen. How to Undo Remove from Home Screen in iPhone As we mentioned before, there are several ways to restore this change on iPhone. Method 1 – Replace App Icon in App Library You can place an app icon on your home screen directly from the App Library. Step 1 – Swipe sideways to find all apps in the app library. Step 2 – Find the app icon you deleted earlier. Step 3 – Simply drag the app icon from the main library to the correct location on the home screen. This is the application diagram
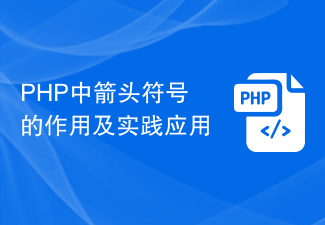
The role and practical application of arrow symbols in PHP In PHP, the arrow symbol (->) is usually used to access the properties and methods of objects. Objects are one of the basic concepts of object-oriented programming (OOP) in PHP. In actual development, arrow symbols play an important role in operating objects. This article will introduce the role and practical application of arrow symbols, and provide specific code examples to help readers better understand. 1. The role of the arrow symbol to access the properties of an object. The arrow symbol can be used to access the properties of an object. When we instantiate a pair
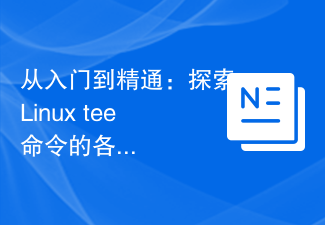
The Linuxtee command is a very useful command line tool that can write output to a file or send output to another command without affecting existing output. In this article, we will explore in depth the various application scenarios of the Linuxtee command, from entry to proficiency. 1. Basic usage First, let’s take a look at the basic usage of the tee command. The syntax of tee command is as follows: tee[OPTION]...[FILE]...This command will read data from standard input and save the data to
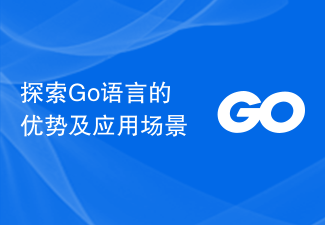
The Go language is an open source programming language developed by Google and first released in 2007. It is designed to be a simple, easy-to-learn, efficient, and highly concurrency language, and is favored by more and more developers. This article will explore the advantages of Go language, introduce some application scenarios suitable for Go language, and give specific code examples. Advantages: Strong concurrency: Go language has built-in support for lightweight threads-goroutine, which can easily implement concurrent programming. Goroutin can be started by using the go keyword
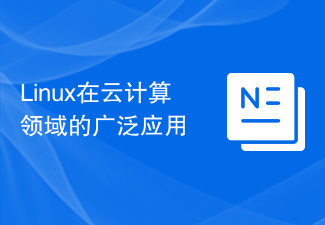
The wide application of Linux in the field of cloud computing With the continuous development and popularization of cloud computing technology, Linux, as an open source operating system, plays an important role in the field of cloud computing. Due to its stability, security and flexibility, Linux systems are widely used in various cloud computing platforms and services, providing a solid foundation for the development of cloud computing technology. This article will introduce the wide range of applications of Linux in the field of cloud computing and give specific code examples. 1. Application virtualization technology of Linux in cloud computing platform Virtualization technology
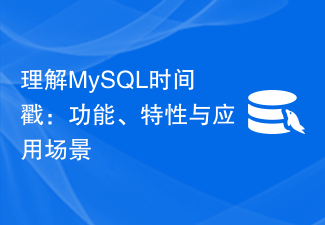
MySQL timestamp is a very important data type, which can store date, time or date plus time. In the actual development process, rational use of timestamps can improve the efficiency of database operations and facilitate time-related queries and calculations. This article will discuss the functions, features, and application scenarios of MySQL timestamps, and explain them with specific code examples. 1. Functions and characteristics of MySQL timestamps There are two types of timestamps in MySQL, one is TIMESTAMP
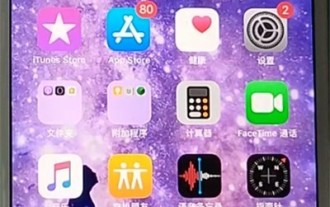
1. First we click on the little white dot. 2. Click the device. 3. Click More. 4. Click Application Switcher. 5. Just close the application background.
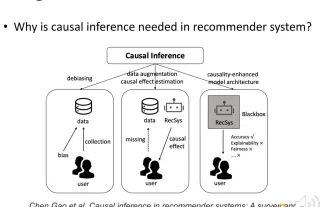
The theme of this sharing is recommendation systems based on causal inference. We review past related work and propose future prospects in this direction. Why do we need to use causal inference techniques in recommender systems? Existing research work uses causal inference to solve three types of problems (see Gaoe et al.'s TOIS2023 paper Causal Inference in Recommender Systems: ASurvey and Future Directions): First, there are various biases (BIAS) in recommendation systems, and causal inference is an effective way to remove these Tools for bias. Recommender systems may face challenges in addressing data scarcity and the inability to accurately estimate causal effects. in order to solve
