AI simulator takes the new SOTA in physics simulation!
Machine learning makes Computer Graphics (CG) simulation more realistic!
The method is called Neural Flow Maps (Neural Flow Maps, NFM), which can accurately simulate the smoke of four vortices Type:
More complex ones can also be easily implemented:
You must know that in this AI application, there are so many In this era, CG physics simulation is still dominated by traditional numerical algorithms.
△NFM simulates "leapfrog"
Although the application of neural networks in CG can create dazzling visual effects, it cannot be rigorous and robust describe physical properties.
△NFM simulates "ink droplets"
It is precisely for this reason that physical simulation based on neural networks is still in proof of concept (proof of concept) stage, the effect generated is far from SOTA.
To solve this complex problem, a research team from Dartmouth College, Georgia Institute of Technology, and Stanford University proposed a new method called neural flow diagram. They combined the advantages of neural networks with advanced physical models to achieve unprecedented visual effects and physical accuracy.
This paper was published in the top journal ACM Transactions on Graphics (TOG), and won the best paper of SIGGRAPH Asia 2023.
What does NFM look like?
The core point of view of the research team is: if you want to use AI to better solve physical problems, you cannot limitly embed learnable modules into existing method frameworks (such as SPH, stable fluids) in.
Existing methods are tailored to the capabilities of traditional numerical methods. Because of this, the development of machine learning has proposed a series of new capabilities(such as NeRF's analysis of spatiotemporal signals) Compact expression) often cannot be found in existing frameworks.
Therefore, researchers believe that instead of applying AI to the existing framework, it is better to design a new mathematical and numerical framework based on the new capabilities proposed by AI to maximize the value of these capabilities. Physical modelBased on the above ideas, the researchers built a model beyond SOTA throughco-design(co-design) of physics and AI fluid simulator.
In the physics part, NFM first uses a set of impulse-basedfluid equations, by performing gauge transformation on the common Euler equation (gauge transformation) , the relationship between the velocity field and the flow map (flow map) and its spatial derivative is established. In other words, as long as an accurate numerical solution to the flow map can be obtained, the evolving velocity field can be accurately reconstructed.
In order to calculate the flow map most accurately, NFM proposes a carefully designed "bidirectional marching"
numerical algorithm. This algorithm is 3 to 5 orders of magnitude more accurate than existing algorithms, but it also requires the storage of long-term spatiotemporal
velocity fields.For large-scale 3D simulations, it is still challenging to store the velocity field of a single frame, but it is completely unfeasible to store the velocity field of tens or hundreds of frames. Therefore, although the "two-way travel" algorithm is accurate, it cannot be realized using traditional means.
Neural Network Storage
(implicit neural representation, or INR)
The ability to perform high-quality spatio-temporal signal compression makes the above highly accurate but unachievable simulation methods feasible.INR usually only needs to be trained once for each scenario, but NFM uses it as an intermediate variable to continuously update during the simulation process. This It also puts forward more stringent requirements on the performance of INR.
In response to this, NFM proposes a new high-performance INR called SSNF.
By automatically planning the opening state of each grid point in spatial sparse storage, and a time processing scheme based on Lagrange polynomials, SSNF achieves faster convergence speed and higher than Instant-NGP, KPlanes and other methods. compression ratio and higher storage accuracy.
Get the latest SOTA
The experimental results show that as an AI-based simulator, NFM significantly surpasses the SOTA method: bimocq, covector fluids and MC R.
In the experiment where 2D point vortex (point vortex) was maintained, the average absolute error of NFM was reduced by at least 14 and at most 308 times compared with the other three.
In the 3D leapfrogging vortices experiment, NFM also significantly improved the ability to conserve energy.
(such as solid interaction, Rayleigh Taylor instability, vortex tube reconnection, etc.) NFM's superiority in creating complex visual effects.
It is worth noting at this level that although AI is used to give more details to the fluid, the existing AI super-resolution algorithm can only improve the picture details, but NFM has a breakthrough in using physical This method improves dynamic details, thereby fundamentally improving the realism of fluid simulations.Project link: https://yitongdeng-projects.github.io/neural_flow_maps_webpage/
The above is the detailed content of AI simulator takes the new SOTA in physics simulation!. For more information, please follow other related articles on the PHP Chinese website!

Hot AI Tools

Undresser.AI Undress
AI-powered app for creating realistic nude photos

AI Clothes Remover
Online AI tool for removing clothes from photos.

Undress AI Tool
Undress images for free

Clothoff.io
AI clothes remover

AI Hentai Generator
Generate AI Hentai for free.

Hot Article

Hot Tools

Notepad++7.3.1
Easy-to-use and free code editor

SublimeText3 Chinese version
Chinese version, very easy to use

Zend Studio 13.0.1
Powerful PHP integrated development environment

Dreamweaver CS6
Visual web development tools

SublimeText3 Mac version
God-level code editing software (SublimeText3)

Hot Topics


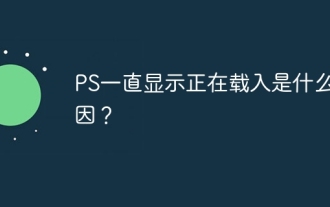
PS "Loading" problems are caused by resource access or processing problems: hard disk reading speed is slow or bad: Use CrystalDiskInfo to check the hard disk health and replace the problematic hard disk. Insufficient memory: Upgrade memory to meet PS's needs for high-resolution images and complex layer processing. Graphics card drivers are outdated or corrupted: Update the drivers to optimize communication between the PS and the graphics card. File paths are too long or file names have special characters: use short paths and avoid special characters. PS's own problem: Reinstall or repair the PS installer.
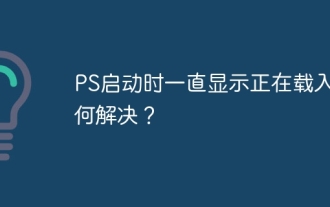
A PS stuck on "Loading" when booting can be caused by various reasons: Disable corrupt or conflicting plugins. Delete or rename a corrupted configuration file. Close unnecessary programs or upgrade memory to avoid insufficient memory. Upgrade to a solid-state drive to speed up hard drive reading. Reinstalling PS to repair corrupt system files or installation package issues. View error information during the startup process of error log analysis.
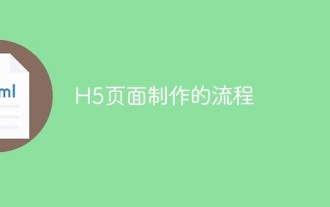
H5 page production process: design: plan page layout, style and content; HTML structure construction: use HTML tags to build a page framework; CSS style writing: use CSS to control the appearance and layout of the page; JavaScript interaction implementation: write code to achieve page animation and interaction; Performance optimization: compress pictures, code and reduce HTTP requests to improve page loading speed.
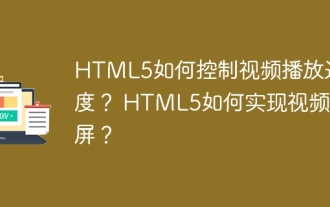
In HTML5, the playback speed of video can be controlled through the playbackRate attribute, which accepts the following values: less than 1: slow playback equals 1: normal speed playback greater than 1: fast playback equals 0: pause in HTML5, the video full screen can be realized through the requestFullscreen() method, which can be applied to video elements or their parent elements.
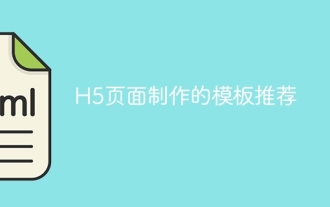
How to choose H5 page template? 1. Clarify the goal: determine the page type (display type, interactive type, static, dynamic) 2. Free templates have risks: poor code quality, simple functions, and security risks 3. Paid templates are more reliable: save effort and avoid unnecessary trouble 4. Choose the appropriate template type: Bootstrap: mature and stable, easy to use, suitable for quickly building prototypes Tailwind CSS: Flexible customization, steep learning curve Vue.js, React: complex interaction, easy to maintain, and high learning cost 5. Template is just a tool, and the core is technical mastery: HTML, CSS, JavaScript 6. View the document before selecting a template to avoid being confused by the preview image
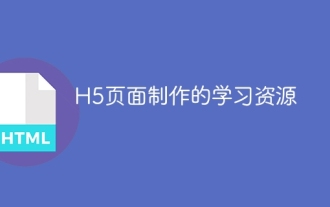
To learn H5 page production, you need to master the three musketeers of HTML, CSS, and JavaScript, and to deeply study the new features of HTML5, CSS selector, layout, animation and other knowledge, master the basics and libraries of JavaScript. Advanced skills include animation effects, responsive design, and server interaction. If you encounter problems, you can use search engines, technology communities, and developer consultation to solve them. Continuously learn new technologies and maintain competitiveness, select suitable learning resources, and persist in practicing is the key.
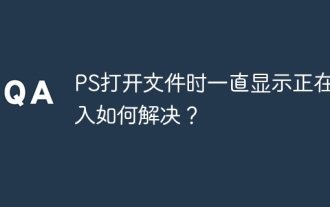
"Loading" stuttering occurs when opening a file on PS. The reasons may include: too large or corrupted file, insufficient memory, slow hard disk speed, graphics card driver problems, PS version or plug-in conflicts. The solutions are: check file size and integrity, increase memory, upgrade hard disk, update graphics card driver, uninstall or disable suspicious plug-ins, and reinstall PS. This problem can be effectively solved by gradually checking and making good use of PS performance settings and developing good file management habits.
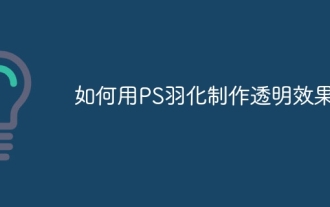
Transparent effect production method: Use selection tool and feathering to cooperate: select transparent areas and feathering to soften edges; change the layer blending mode and opacity to control transparency. Use masks and feathers: select and feather areas; add layer masks, and grayscale gradient control transparency.
