


Classic algorithms in Python concurrent programming: using multi-threading to solve tough problems
Multi-threadingProgramming is a powerful technique in python to solve complex problems. It improves the efficiency and performance of your program by performing multiple tasks simultaneously. This article explores the classic algorithm in Python and shows how to take advantage of multiple threads to enhance its functionality.
Multi-threading, Python, classic algorithms, parallel processing, tricky problems
Multi-threading allows Python programs to perform multiple tasks simultaneously, thereby improving performance and maximizing the use of available resources. Here are some common classic Python algorithms that can be significantly improved with multithreading:
-
Fast Fourier Transform (FFT): FFT is an algorithm for quickly calculating convolutions. By breaking the problem into smaller parts and using multiple threads to execute these parts in parallel, the execution time of the algorithm can be significantly reduced.
-
Genetic Algorithm (GA): GA is an algorithm used to solve optimization problems. By creating multiple processing threads to evaluate different populations, GA can significantly speed up convergence and find better solutions.
-
Depth First Search (DFS): DFS is an algorithm for traversing directed or undirected graphs. Leveraging multithreading allows you to explore different branches of the graph in parallel, thereby reducing traversal time.
Demo code:
The following example demonstrates how to use multi-threading in Python to speed up the FFT algorithm:
import numpy as np from concurrent.futures import ThreadPoolExecutor def fft_thread(x): return np.fft.fft(x) def fft_parallel(x, num_threads): with ThreadPoolExecutor(num_threads) as executor: results = executor.map(fft_thread, np.split(x, num_threads)) return np.concatenate(results)
Advantage:
- Improve efficiency: Multithreading can significantly increase the speed of algorithm execution, especially when tasks can be subdivided into smaller parallel parts.
- Optimize Resource Utilization: Multiple threads can maximize the use of available processor cores, thereby reducing idle time and improving overall performance.
- Enhance algorithm performance: By executing different parts of the algorithm in parallel, multi-threading can help the algorithm explore the search space or handle complex calculations more efficiently.
in conclusion:
Multithreading is a powerful technique in Python for solving tough problems. By performing multiple tasks simultaneously, it improves program efficiency, optimizes resource utilization, and enhances the performance of classic algorithms. As multi-threading capabilities continue to increase in Python, we can see more and more algorithms leveraging the power of multi-threading to improve performance in the future.
The above is the detailed content of Classic algorithms in Python concurrent programming: using multi-threading to solve tough problems. For more information, please follow other related articles on the PHP Chinese website!

Hot AI Tools

Undresser.AI Undress
AI-powered app for creating realistic nude photos

AI Clothes Remover
Online AI tool for removing clothes from photos.

Undress AI Tool
Undress images for free

Clothoff.io
AI clothes remover

AI Hentai Generator
Generate AI Hentai for free.

Hot Article

Hot Tools

Notepad++7.3.1
Easy-to-use and free code editor

SublimeText3 Chinese version
Chinese version, very easy to use

Zend Studio 13.0.1
Powerful PHP integrated development environment

Dreamweaver CS6
Visual web development tools

SublimeText3 Mac version
God-level code editing software (SublimeText3)

Hot Topics


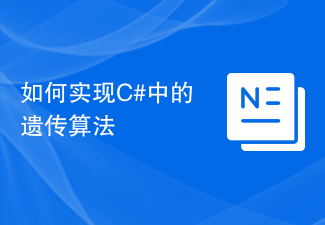
How to implement genetic algorithm in C# Introduction: Genetic algorithm is an optimization algorithm that simulates the mechanism of natural selection and genetic inheritance. Its main idea is to search for the optimal solution by simulating the process of biological evolution. In the field of computer science, genetic algorithms are widely used to solve optimization problems, such as machine learning, parameter optimization, combinatorial optimization, etc. This article will introduce how to implement a genetic algorithm in C# and provide specific code examples. 1. Basic Principles of Genetic Algorithms Genetic algorithms represent candidate solutions in the solution space by using coding, and use selection, crossover and
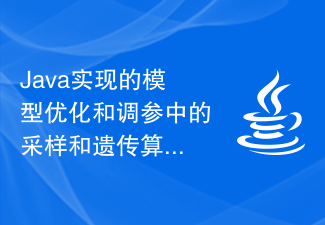
Sampling and genetic algorithm technology and applications in model optimization and parameter adjustment implemented in Java With the widespread application of machine learning in various industries, model training and parameter adjustment have become important research directions. The goal of model optimization and parameter tuning is to find the optimal combination of model parameters to improve the accuracy and generalization ability of the model. Sampling and genetic algorithms are two commonly used model optimization and parameter adjustment technologies, which have high efficiency and reliability. This article will introduce the working principles and application cases of sampling and genetic algorithms in model optimization and parameter adjustment implemented in Java. Sampling algorithm
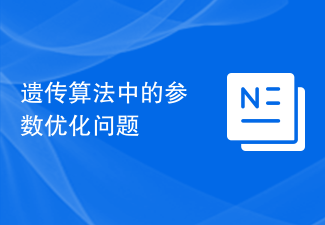
Parameter optimization problems in genetic algorithms require specific code examples. With the continuous advancement and development of science and technology, genetic algorithms have become a powerful tool for solving complex problems. Genetic algorithms simulate the evolutionary process in the biological world and perform parameter optimization and problem solving through operations such as natural selection, genetic variation, and genetic crossover. This article will introduce the parameter optimization problem in genetic algorithms and give specific code examples. In genetic algorithms, parameter optimization refers to adjusting the parameters of the genetic algorithm to obtain better solution results. Common parameters include population size
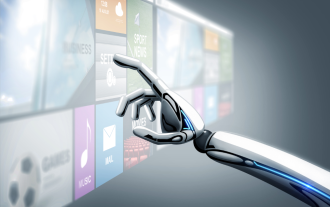
Reinforcement learning algorithm (RL) and evolutionary algorithm (EA) are two unique algorithms in the field of machine learning. Although they both belong to the category of machine learning, there are obvious differences in the methods and concepts of problem solving. Reinforcement learning algorithm: Reinforcement learning is a machine learning method whose core lies in the agent interacting with the environment and learning optimal behavioral strategies through trial and error to maximize cumulative rewards. The key to reinforcement learning is that the agent constantly tries various behaviors and adjusts its strategy based on reward signals. By interacting with the environment, the agent gradually optimizes its decision-making process to achieve the established goal. This method imitates the way humans learn, improving performance through continuous trial and error and adjustments, allowing the agent to perform complex tasks, including the main components of reinforcement learning.
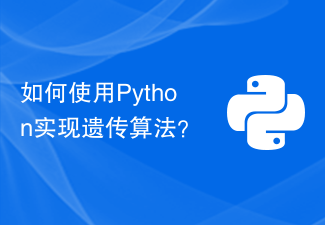
How to implement genetic algorithm using Python? Introduction: Genetic algorithm, as a computational model that simulates the evolutionary process of biological evolution, has been widely used in solving optimization problems. Python, as a powerful programming language that is easy to learn and use, provides a wealth of libraries and tools to implement genetic algorithms. This article will introduce how to use Python to implement a genetic algorithm and provide specific code examples. 1. Overview of Genetic Algorithms Genetic algorithms simulate the process of biological evolution and gradually optimize through operations such as selection, crossover and mutation.
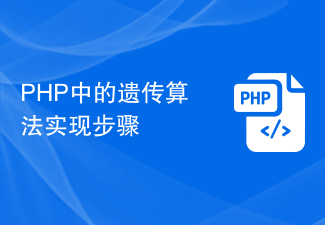
Introduction to the implementation steps of genetic algorithm in PHP: Genetic algorithm is an optimization algorithm based on the principle of evolution. By simulating the genetic and evolutionary process of nature, it can find the optimal solution in the solution space of the search problem. In PHP, we can use genetic algorithms to solve some optimization problems, such as solving parameter optimization, machine learning, scheduling problems, etc. This article will introduce the implementation steps of genetic algorithm in PHP and provide relevant code examples. 1. Initializing the population In the genetic algorithm, the population refers to a set of solutions to be optimized. First, we need to define
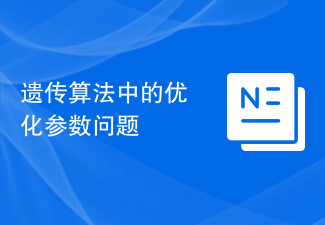
The optimization parameter problem in genetic algorithm requires specific code examples. Summary: Genetic algorithm is an optimization algorithm that simulates the evolutionary process and can be applied to various optimization problems. This article will focus on the optimization parameter problem in genetic algorithms and give specific code examples. Introduction: Genetic algorithm is an optimization algorithm inspired by the theory of biological evolution. Its basic idea is to search for the optimal solution to the problem by simulating operations such as selection, crossover, and mutation in the evolutionary process. Genetic algorithm has the advantages of adaptability and parallelism. It can be used when the objective function is complex and there are many parameters.
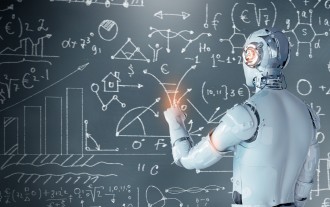
Integrating artificial intelligence technology into various products has become a game changer, especially in network service systems. The definition of artificial intelligence has expanded to include heuristics and probabilities in programming code, paving the way for more efficient data processing and problem-solving capabilities. The machine learning (ML) market is booming globally. In 2022, it will be worth approximately $19.2 billion. Experts predict that this number will soar to $225.91 billion by 2030. This article delves into the profound impact of artificial intelligence and machine learning (ML) on web services, revealing how they are revolutionizing the way we process large amounts of data. In the past few years, machine learning technology has made huge breakthroughs in various fields, especially in data processing
