


RNN model challenges Transformer hegemony! 1% cost and performance comparable to Mistral-7B, supporting 100+ languages, the most in the world
While large models are being rolled in, Transformer’s status is also being challenged one after another.
Recently, RWKV released the Eagle 7B model, based on the latest RWKV-v5 architecture.
Eagle 7B excels in multilingual benchmarks and is on par with top models in English tests.
At the same time, Eagle 7B uses the RNN architecture. Compared with the Transformer model of the same size, the inference cost is reduced by more than 10-100 times. It can be said to be the most environmentally friendly 7B in the world. Model.
Since the RWKV-v5 paper may not be released until next month, we first provide the RWKV paper, which is the first non-Transformer architecture to scale parameters to tens of billions.
Picture
Paper address: https://arxiv.org/pdf/2305.13048.pdf
This work was accepted by EMNLP 2023. The authors come from top universities, research institutions and technology companies around the world.
The following is the official picture of Eagle 7B, showing that the eagle is flying over Transformers.
Pictures
Eagle 7B
Eagle 7B is available in over 100 languages With 1.1T (trillion) Token training data, Eagle 7B ranked first in average score in the multi-language benchmark test in the figure below.
Benchmarks include xLAMBDA, xStoryCloze, xWinograd, and xCopa, covering 23 languages, as well as commonsense reasoning in their respective languages.
Eagle 7B won the first place in three of them. Although one of them did not beat Mistral-7B and ranked second, the training data used by the opponent was much higher than Eagle.
Picture
The English test pictured below contains 12 separate benchmarks, common sense reasoning, and world knowledge.
In the English performance test, the level of Eagle 7B is close to Falcon (1.5T), LLaMA2 (2T), and Mistral (>2T), which also uses about 1T training data. MPT-7B is comparable.
Picture
And, in both tests, the new v5 architecture has huge improvements compared to the previous v4 overall leap.
Eagle 7B is currently hosted by the Linux Foundation and is licensed under the Apache 2.0 license for unrestricted personal or commercial use.
Multi-language support
As mentioned earlier, the training data of Eagle 7B comes from more than 100 languages, and the 4 multi-languages used above The benchmark only included 23 languages.
Picture
Although it achieved first place, in general, Eagle 7B suffered a loss, after all , the benchmark cannot directly evaluate the model's performance in more than 70 other languages.
The extra training cost will not help you improve your rankings. If you focus on English, you may get better results than you do now.
——So, why did RWKV do this? The official said:
Building inclusive AI for everyone in this world —— not just the English
##In response to the numerous feedback on the RWKV model Among them, the most common are:
Multilingual approaches hurt the model’s English evaluation score and slow down the development of the linear Transformer;
It is unfair to compare the multilingual performance of multilingual models with pure English models
The official said, "In most cases, we agree with these opinions,"
"But we have no plans to change that, because we are building artificial intelligence for the world - and it's not just an English-speaking world."
Picture
In 2023, only 17% of the world’s population will speak English (approximately 1.3 billion people), however, by supporting the top 25 languages in the world, the model can cover approximately 40 billion people, or 50% of the world’s total population.
The team hopes that future artificial intelligence can help everyone, such as allowing models to run on low-end hardware at a low price, such as supporting more languages.
The team will gradually expand the multilingual data set to support a wider range of languages, and slowly expand the coverage to 100% of the world's regions, - ensuring that there is no language was left out.
Data set scalable architecture
During the training process of the model, there is a phenomenon worth noting:
As the size of training data continues to increase, the performance of the model gradually improves. When the training data reaches about 300B, the model shows similar performance to pythia-6.9b, while the latter's training data volume is 300B.
Picture
This phenomenon is the same as a previous experiment conducted on the RWKV-v4 architecture - that is to say , when the size of the training data is the same, the performance of a linear Transformer like RWKV will be similar to that of the Transformer.
So we can’t help but ask, if this is indeed the case, is the data more important to the performance improvement of the model than the exact architecture?
Picture
We know that the calculation and storage cost of the Transformer class model is square, and in the picture above The computational cost of the RWKV architecture only increases linearly with the number of Tokens.
Perhaps we should pursue more efficient and scalable architectures that increase accessibility, lower the cost of AI for everyone, and reduce environmental impact.
RWKV
The RWKV architecture is an RNN with GPT-level LLM performance, and at the same time can be trained in parallel like Transformer.
RWKV combines the advantages of RNN and Transformer - excellent performance, fast inference, fast training, VRAM saving, "unlimited" context length and free sentence embedding. RWKV does not Use attention mechanism.
The following figure shows the comparison of computational costs between RWKV and Transformer models:
Picture
In order to solve the time and space complexity problems of Transformer, researchers have proposed a variety of architectures:
Picture
The RWKV architecture consists of a series of stacked residual blocks, each residual block consists of a time mixing with a loop structure and a channel mixing sub-block
In the figure below RWKV block elements on the left, RWKV residual block on the right, and the final header for language modeling.
Picture
Recursion can be expressed as linear interpolation between the current input and the input of the previous time step (as shown in the figure below ), can be adjusted independently for each linear projection of the input embedding.
A vector that handles the current Token separately is also introduced here to compensate for potential degradation.
Picture
RWKV can be efficiently parallelized (matrix multiplication) in what we call temporal parallelism mode.
In a recurrent network, the output of the previous moment is usually used as the input of the current moment. This is particularly evident in autoregressive decoding inference for language models, which requires each token to be computed before inputting the next step, allowing RWKV to take advantage of its RNN-like structure, called temporal mode.
In this case, RWKV can be conveniently formulated recursively for decoding during inference, which takes advantage of each output token relying only on the latest state, state The size of is constant regardless of sequence length.
Then then acts as an RNN decoder, yielding constant speed and memory footprint relative to sequence length, enabling longer sequences to be processed more efficiently.
In contrast, self-attention’s KV cache grows continuously relative to the sequence length, resulting in decreased efficiency and increased memory usage and time as the sequence lengthens.
Reference:
The above is the detailed content of RNN model challenges Transformer hegemony! 1% cost and performance comparable to Mistral-7B, supporting 100+ languages, the most in the world. For more information, please follow other related articles on the PHP Chinese website!

Hot AI Tools

Undresser.AI Undress
AI-powered app for creating realistic nude photos

AI Clothes Remover
Online AI tool for removing clothes from photos.

Undress AI Tool
Undress images for free

Clothoff.io
AI clothes remover

AI Hentai Generator
Generate AI Hentai for free.

Hot Article

Hot Tools

Notepad++7.3.1
Easy-to-use and free code editor

SublimeText3 Chinese version
Chinese version, very easy to use

Zend Studio 13.0.1
Powerful PHP integrated development environment

Dreamweaver CS6
Visual web development tools

SublimeText3 Mac version
God-level code editing software (SublimeText3)

Hot Topics


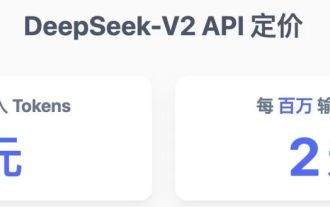
Imagine an artificial intelligence model that not only has the ability to surpass traditional computing, but also achieves more efficient performance at a lower cost. This is not science fiction, DeepSeek-V2[1], the world’s most powerful open source MoE model is here. DeepSeek-V2 is a powerful mixture of experts (MoE) language model with the characteristics of economical training and efficient inference. It consists of 236B parameters, 21B of which are used to activate each marker. Compared with DeepSeek67B, DeepSeek-V2 has stronger performance, while saving 42.5% of training costs, reducing KV cache by 93.3%, and increasing the maximum generation throughput to 5.76 times. DeepSeek is a company exploring general artificial intelligence
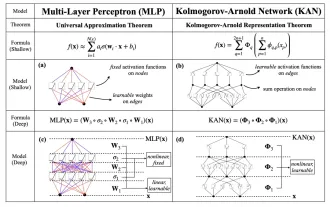
Earlier this month, researchers from MIT and other institutions proposed a very promising alternative to MLP - KAN. KAN outperforms MLP in terms of accuracy and interpretability. And it can outperform MLP running with a larger number of parameters with a very small number of parameters. For example, the authors stated that they used KAN to reproduce DeepMind's results with a smaller network and a higher degree of automation. Specifically, DeepMind's MLP has about 300,000 parameters, while KAN only has about 200 parameters. KAN has a strong mathematical foundation like MLP. MLP is based on the universal approximation theorem, while KAN is based on the Kolmogorov-Arnold representation theorem. As shown in the figure below, KAN has
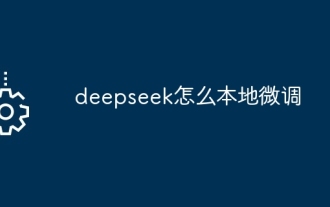
Local fine-tuning of DeepSeek class models faces the challenge of insufficient computing resources and expertise. To address these challenges, the following strategies can be adopted: Model quantization: convert model parameters into low-precision integers, reducing memory footprint. Use smaller models: Select a pretrained model with smaller parameters for easier local fine-tuning. Data selection and preprocessing: Select high-quality data and perform appropriate preprocessing to avoid poor data quality affecting model effectiveness. Batch training: For large data sets, load data in batches for training to avoid memory overflow. Acceleration with GPU: Use independent graphics cards to accelerate the training process and shorten the training time.
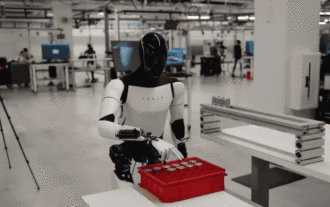
The latest video of Tesla's robot Optimus is released, and it can already work in the factory. At normal speed, it sorts batteries (Tesla's 4680 batteries) like this: The official also released what it looks like at 20x speed - on a small "workstation", picking and picking and picking: This time it is released One of the highlights of the video is that Optimus completes this work in the factory, completely autonomously, without human intervention throughout the process. And from the perspective of Optimus, it can also pick up and place the crooked battery, focusing on automatic error correction: Regarding Optimus's hand, NVIDIA scientist Jim Fan gave a high evaluation: Optimus's hand is the world's five-fingered robot. One of the most dexterous. Its hands are not only tactile
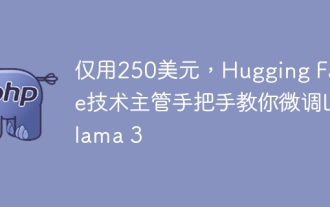
The familiar open source large language models such as Llama3 launched by Meta, Mistral and Mixtral models launched by MistralAI, and Jamba launched by AI21 Lab have become competitors of OpenAI. In most cases, users need to fine-tune these open source models based on their own data to fully unleash the model's potential. It is not difficult to fine-tune a large language model (such as Mistral) compared to a small one using Q-Learning on a single GPU, but efficient fine-tuning of a large model like Llama370b or Mixtral has remained a challenge until now. Therefore, Philipp Sch, technical director of HuggingFace
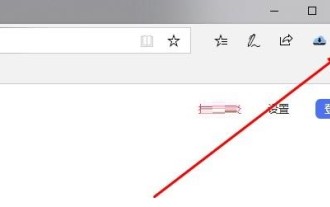
1. First, enter the Edge browser and click the three dots in the upper right corner. 2. Then, select [Extensions] in the taskbar. 3. Next, close or uninstall the plug-ins you do not need.
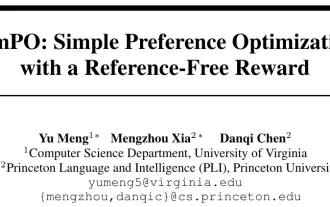
In order to align large language models (LLMs) with human values and intentions, it is critical to learn human feedback to ensure that they are useful, honest, and harmless. In terms of aligning LLM, an effective method is reinforcement learning based on human feedback (RLHF). Although the results of the RLHF method are excellent, there are some optimization challenges involved. This involves training a reward model and then optimizing a policy model to maximize that reward. Recently, some researchers have explored simpler offline algorithms, one of which is direct preference optimization (DPO). DPO learns the policy model directly based on preference data by parameterizing the reward function in RLHF, thus eliminating the need for an explicit reward model. This method is simple and stable
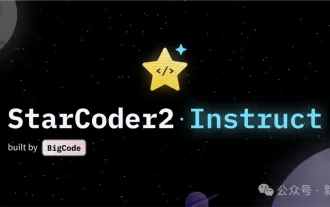
At the forefront of software technology, UIUC Zhang Lingming's group, together with researchers from the BigCode organization, recently announced the StarCoder2-15B-Instruct large code model. This innovative achievement achieved a significant breakthrough in code generation tasks, successfully surpassing CodeLlama-70B-Instruct and reaching the top of the code generation performance list. The unique feature of StarCoder2-15B-Instruct is its pure self-alignment strategy. The entire training process is open, transparent, and completely autonomous and controllable. The model generates thousands of instructions via StarCoder2-15B in response to fine-tuning the StarCoder-15B base model without relying on expensive manual annotation.
