


Tips for quickly creating multi-dimensional arrays using Numpy
Tips to quickly create multi-dimensional arrays using Numpy
Numpy is one of the most commonly used scientific computing libraries in Python. It provides efficient multi-dimensional array (ndarray) objects , and supports various array operations and mathematical operations. In data analysis and numerical calculations, it is often necessary to create and manipulate multidimensional arrays. This article will introduce some techniques for quickly creating multi-dimensional arrays using Numpy, and attach specific code examples.
-
Create one-dimensional array
Numpy's one-dimensional array can be created directly using a list object. For example, to create a one-dimensional array containing the integers 1 to 5, you can use the following code:1
2
3
import numpy
as
np
arr = np.
array
([1, 2, 3, 4, 5])
print
(arr)
Copy after loginThe output result is: [1 2 3 4 5].
Create a two-dimensional array
When creating a two-dimensional array, you can use a list of lists to represent data in matrix form. For example, to create a two-dimensional array with 3 rows and 3 columns, you can use the following code:1
2
3
import numpy
as
np
arr = np.
array
([[1, 2, 3], [4, 5, 6], [7, 8, 9]])
print
(arr)
Copy after loginThe output result is:
1
2
3
[[1 2 3]
[4 5 6]
[7 8 9]]
Copy after loginIn addition, you can also use some functions provided by Numpy to create specific shapes two-dimensional array. For example, to create an all-zero matrix with 3 rows and 3 columns, you can use the following code:
1
2
3
import numpy
as
np
arr = np.zeros((3, 3))
print
(arr)
Copy after loginThe output result is:
1
2
3
[[0. 0. 0.]
[0. 0. 0.]
[0. 0. 0.]]
Copy after loginCreate a multi-dimensional array
Numpy supports creation Arrays of arbitrary dimensions. For example, to create a three-dimensional array with 3 rows, 3 columns and 3 depths, you can use the following code:1
2
3
4
5
import numpy
as
np
arr = np.
array
([[[1, 2, 3], [4, 5, 6], [7, 8, 9]],
[[10, 11, 12], [13, 14, 15], [16, 17, 18]],
[[19, 20, 21], [22, 23, 24], [25, 26, 27]]])
print
(arr)
Copy after loginThe output result is:
1
2
3
4
5
6
7
8
9
10
11
[[[ 1 2 3]
[ 4 5 6]
[ 7 8 9]]
[[10 11 12]
[13 14 15]
[16 17 18]]
[[19 20 21]
[22 23 24]
[25 26 27]]]
Copy after loginUse the functions provided by Numpy to create a specific shape Array
In practical applications, we sometimes need to create arrays of certain shapes. Numpy provides some functions to easily create these arrays. For example:- np.zeros(shape): Creates an array of all zeros, shape is a tuple parameter representing the shape.
- np.ones(shape): Create an all-one array, the shape parameters are the same as above.
- np.full(shape, value): Create an array of the specified shape, each element has the same value value.
- np.eye(N): Create an identity matrix with N rows and N columns.
- np.random.random(shape): Create a random array of a specified shape, with elements ranging from 0 to 1.
The following are a few examples:
1
2
3
4
5
6
7
8
9
10
11
12
13
14
15
16
import numpy
as
np
arr_zeros = np.zeros((2, 3)) # 创建一个2行3列的全零数组
print
(arr_zeros)
arr_ones = np.ones((2, 3)) # 创建一个2行3列的全一数组
print
(arr_ones)
arr_full = np.full((2, 3), 5) # 创建一个2行3列的数组,每个元素都是5
print
(arr_full)
arr_eye = np.eye(3) # 创建一个3行3列的单位矩阵
print
(arr_eye)
arr_random = np.random.random((2, 3)) # 创建一个2行3列的随机数组
print
(arr_random)
Copy after loginThe output result is:
1
2
3
4
5
6
7
8
9
10
11
12
13
14
15
[[0. 0. 0.]
[0. 0. 0.]]
[[1. 1. 1.]
[1. 1. 1.]]
[[5 5 5]
[5 5 5]]
[[1. 0. 0.]
[0. 1. 0.]
[0. 0. 1.]]
[[0.34634205 0.24187985 0.32349873]
[0.76366044 0.10267694 0.07813336]]
Copy after login
Create multi-dimensional arrays through various techniques provided by Numpy , we can easily create arrays of various shapes and use them in scientific calculations and data analysis. At the same time, Numpy also provides a wealth of array operation functions and mathematical operation methods, which can efficiently handle computing tasks on multi-dimensional arrays. For users who use Numpy for scientific computing and data analysis, it is very important to master the skills of quickly creating multi-dimensional arrays.
The above is the detailed content of Tips for quickly creating multi-dimensional arrays using Numpy. For more information, please follow other related articles on the PHP Chinese website!

Hot AI Tools

Undresser.AI Undress
AI-powered app for creating realistic nude photos

AI Clothes Remover
Online AI tool for removing clothes from photos.

Undress AI Tool
Undress images for free

Clothoff.io
AI clothes remover

AI Hentai Generator
Generate AI Hentai for free.

Hot Article

Hot Tools

Notepad++7.3.1
Easy-to-use and free code editor

SublimeText3 Chinese version
Chinese version, very easy to use

Zend Studio 13.0.1
Powerful PHP integrated development environment

Dreamweaver CS6
Visual web development tools

SublimeText3 Mac version
God-level code editing software (SublimeText3)

Hot Topics


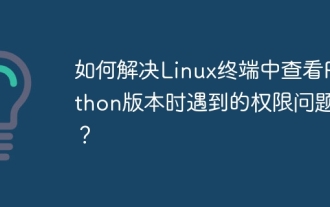
Solution to permission issues when viewing Python version in Linux terminal When you try to view Python version in Linux terminal, enter python...
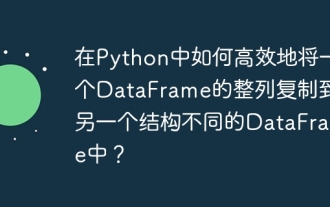
When using Python's pandas library, how to copy whole columns between two DataFrames with different structures is a common problem. Suppose we have two Dats...
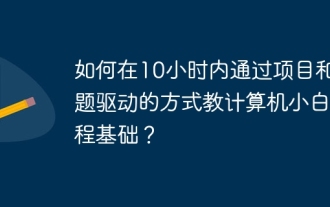
How to teach computer novice programming basics within 10 hours? If you only have 10 hours to teach computer novice some programming knowledge, what would you choose to teach...
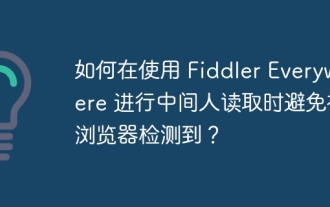
How to avoid being detected when using FiddlerEverywhere for man-in-the-middle readings When you use FiddlerEverywhere...
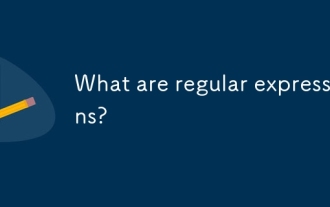
Regular expressions are powerful tools for pattern matching and text manipulation in programming, enhancing efficiency in text processing across various applications.
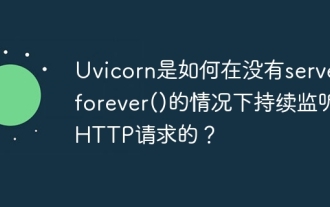
How does Uvicorn continuously listen for HTTP requests? Uvicorn is a lightweight web server based on ASGI. One of its core functions is to listen for HTTP requests and proceed...
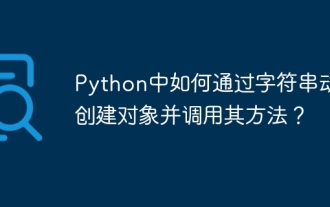
In Python, how to dynamically create an object through a string and call its methods? This is a common programming requirement, especially if it needs to be configured or run...
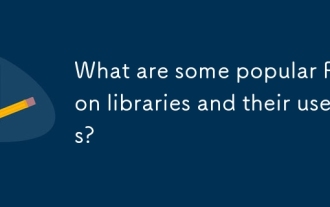
The article discusses popular Python libraries like NumPy, Pandas, Matplotlib, Scikit-learn, TensorFlow, Django, Flask, and Requests, detailing their uses in scientific computing, data analysis, visualization, machine learning, web development, and H
