


more_itertools cannot import cached_property from functools in Python 3.6
I tried running grade_analysis.py from the terminal in visual studio code using the following command:
~/documents/school/ml4t_2023fall/assess_portfolio$ pythonpath=../:. python grade_analysis.py
According to the class setting instructions
However, when I run the command, grade_analysis.py doesn't seem to be able to level up and get the information from the grading.grading.py file.
Am I using this command wrong or am I missing something?
This is the error I receive:
2023fall/assess_portfolio$ pythonpath=../:. python grade_analysis.py traceback (most recent call last): file "grade_analysis.py", line 20, in <module> import pytest file "/home/clopez/miniconda3/envs/ml4t/lib/python3.6/site-packages/pytest.py", line 34, in <module> from _pytest.python_api import approx file "/home/clopez/miniconda3/envs/ml4t/lib/python3.6/site-packages/_pytest/python_api.py", line 13, in <module> from more_itertools.more import always_iterable file "/home/clopez/miniconda3/envs/ml4t/lib/python3.6/site-packages/more_itertools/__init__.py", line 3, in <module> from .more import * # noqa file "/home/clopez/miniconda3/envs/ml4t/lib/python3.6/site-packages/more_itertools/more.py", line 5, in <module> from functools import cached_property, partial, reduce, wraps importerror: cannot import name 'cached_property'
Environment setting instructions
conda environment yml
name: ml4t channels: - conda-forge - defaults dependencies: - python=3.6 - cycler=0.10.0 - kiwisolver=1.1.0 - matplotlib=3.0.3 - numpy=1.16.3 - pandas=0.24.2 - pyparsing=2.4.0 - python-dateutil=2.8.0 - pytz=2019.1 - scipy=1.2.1 - seaborn=0.9.0 - six=1.12.0 - joblib=0.13.2 - pytest=5.0 - pytest-json=0.4.0 - future=0.17.1 - pprofile=2.0.2 - pip - pip: - jsons==0.8.8 - gradescope-utils - subprocess32
Level analysis.py
"""MC1-P1: Analyze a portfolio - grading script. Usage: - Switch to a student feedback directory first (will write "points.txt" and "comments.txt" in pwd). - Run this script with both ml4t/ and student solution in PYTHONPATH, e.g.: PYTHONPATH=ml4t:MC1-P1/jdoe7 python ml4t/mc1_p1_grading/grade_analysis.py Copyright 2017, Georgia Tech Research Corporation Atlanta, Georgia 30332-0415 All Rights Reserved """ import datetime import os import sys import traceback as tb from collections import OrderedDict, namedtuple import pandas as pd import pytest from grading.grading import ( GradeResult, IncorrectOutput, grader, run_with_timeout, ) from util import get_data # Student code # Spring '16 renamed package to just "analysis" (BPH) main_code = "analysis" # module name to import # Test cases # Spring '16 test cases only check sharp ratio, avg daily ret, and cum_ret (BPH) PortfolioTestCase = namedtuple( "PortfolioTestCase", ["inputs", "outputs", "description"] ) portfolio_test_cases = [ PortfolioTestCase( inputs=dict( start_date="2010-01-01", end_date="2010-12-31", symbol_allocs=OrderedDict( [("GOOG", 0.2), ("AAPL", 0.3), ("GLD", 0.4), ("XOM", 0.1)] ), start_val=1000000, ), outputs=dict( cum_ret=0.255646784534, avg_daily_ret=0.000957366234238, sharpe_ratio=1.51819243641, ), description="Wiki example 1", ), PortfolioTestCase( inputs=dict( start_date="2010-01-01", end_date="2010-12-31", symbol_allocs=OrderedDict( [("AXP", 0.0), ("HPQ", 0.0), ("IBM", 0.0), ("HNZ", 1.0)] ), start_val=1000000, ), outputs=dict( cum_ret=0.198105963655, avg_daily_ret=0.000763106152672, sharpe_ratio=1.30798398744, ), description="Wiki example 2", ), PortfolioTestCase( inputs=dict( start_date="2010-06-01", end_date="2010-12-31", symbol_allocs=OrderedDict( [("GOOG", 0.2), ("AAPL", 0.3), ("GLD", 0.4), ("XOM", 0.1)] ), start_val=1000000, ), outputs=dict( cum_ret=0.205113938792, avg_daily_ret=0.00129586924366, sharpe_ratio=2.21259766672, ), description="Wiki example 3: Six month range", ), PortfolioTestCase( inputs=dict( start_date="2010-01-01", end_date="2013-05-31", symbol_allocs=OrderedDict( [("AXP", 0.3), ("HPQ", 0.5), ("IBM", 0.1), ("GOOG", 0.1)] ), start_val=1000000, ), outputs=dict( cum_ret=-0.110888530433, avg_daily_ret=-6.50814806831e-05, sharpe_ratio=-0.0704694718385, ), description="Normalization check", ), PortfolioTestCase( inputs=dict( start_date="2010-01-01", end_date="2010-01-31", symbol_allocs=OrderedDict( [("AXP", 0.9), ("HPQ", 0.0), ("IBM", 0.1), ("GOOG", 0.0)] ), start_val=1000000, ), outputs=dict( cum_ret=-0.0758725033871, avg_daily_ret=-0.00411578300489, sharpe_ratio=-2.84503813366, ), description="One month range", ), PortfolioTestCase( inputs=dict( start_date="2011-01-01", end_date="2011-12-31", symbol_allocs=OrderedDict( [("WFR", 0.25), ("ANR", 0.25), ("MWW", 0.25), ("FSLR", 0.25)] ), start_val=1000000, ), outputs=dict( cum_ret=-0.686004563165, avg_daily_ret=-0.00405018240566, sharpe_ratio=-1.93664660013, ), description="Low Sharpe ratio", ), PortfolioTestCase( inputs=dict( start_date="2010-01-01", end_date="2010-12-31", symbol_allocs=OrderedDict( [("AXP", 0.0), ("HPQ", 1.0), ("IBM", 0.0), ("HNZ", 0.0)] ), start_val=1000000, ), outputs=dict( cum_ret=-0.191620333598, avg_daily_ret=-0.000718040989619, sharpe_ratio=-0.71237182415, ), description="All your eggs in one basket", ), PortfolioTestCase( inputs=dict( start_date="2006-01-03", end_date="2008-01-02", symbol_allocs=OrderedDict( [("MMM", 0.0), ("MO", 0.9), ("MSFT", 0.1), ("INTC", 0.0)] ), start_val=1000000, ), outputs=dict( cum_ret=0.43732715979, avg_daily_ret=0.00076948918955, sharpe_ratio=1.26449481371, ), description="Two year range", ), ] abs_margins = dict( cum_ret=0.001, avg_daily_ret=0.00001, sharpe_ratio=0.001 ) # absolute margin of error for each output points_per_output = dict( cum_ret=2.5, avg_daily_ret=2.5, sharpe_ratio=5.0 ) # points for each output, for partial credit points_per_test_case = sum(points_per_output.values()) max_seconds_per_call = 5 # Grading parameters (picked up by module-level grading fixtures) max_points = float(len(portfolio_test_cases) * points_per_test_case) html_pre_block = ( True # surround comments with HTML <pre class="brush:php;toolbar:false"> tag (for T-Square comments field) ) # Test functon(s) @pytest.mark.parametrize("inputs,outputs,description", portfolio_test_cases) def test_analysis(inputs, outputs, description, grader): """Test get_portfolio_value() and get_portfolio_stats() return correct values. Requires test inputs, expected outputs, description, and a grader fixture. """ points_earned = 0.0 # initialize points for this test case try: # Try to import student code (only once) if not main_code in globals(): import importlib # * Import module mod = importlib.import_module(main_code) globals()[main_code] = mod # Unpack test case start_date_str = inputs["start_date"].split("-") start_date = datetime.datetime( int(start_date_str[0]), int(start_date_str[1]), int(start_date_str[2]), ) end_date_str = inputs["end_date"].split("-") end_date = datetime.datetime( int(end_date_str[0]), int(end_date_str[1]), int(end_date_str[2]) ) symbols = list( inputs["symbol_allocs"].keys() ) # e.g.: ['GOOG', 'AAPL', 'GLD', 'XOM'] allocs = list( inputs["symbol_allocs"].values() ) # e.g.: [0.2, 0.3, 0.4, 0.1] start_val = inputs["start_val"] risk_free_rate = inputs.get("risk_free_rate", 0.0) # the wonky unpacking here is so that we only pull out the values we say we'll test. def timeoutwrapper_analysis(): student_rv = analysis.assess_portfolio( sd=start_date, ed=end_date, syms=symbols, allocs=allocs, sv=start_val, rfr=risk_free_rate, sf=252.0, gen_plot=False, ) return student_rv result = run_with_timeout( timeoutwrapper_analysis, max_seconds_per_call, (), {} ) student_cr = result[0] student_adr = result[1] student_sr = result[3] port_stats = OrderedDict( [ ("cum_ret", student_cr), ("avg_daily_ret", student_adr), ("sharpe_ratio", student_sr), ] ) # Verify against expected outputs and assign points incorrect = False msgs = [] for key, value in port_stats.items(): if abs(value - outputs[key]) > abs_margins[key]: incorrect = True msgs.append( " {}: {} (expected: {})".format( key, value, outputs[key] ) ) else: points_earned += points_per_output[key] # partial credit if incorrect: inputs_str = ( " start_date: {}\n" " end_date: {}\n" " symbols: {}\n" " allocs: {}\n" " start_val: {}".format( start_date, end_date, symbols, allocs, start_val ) ) raise IncorrectOutput( "One or more stats were incorrect.\n Inputs:\n{}\n Wrong" " values:\n{}".format(inputs_str, "\n".join(msgs)) ) except Exception as e: # Test result: failed msg = "Test case description: {}\n".format(description) # Generate a filtered stacktrace, only showing erroneous lines in student file(s) tb_list = tb.extract_tb(sys.exc_info()[2]) for i in range(len(tb_list)): row = tb_list[i] tb_list[i] = ( os.path.basename(row[0]), row[1], row[2], row[3], ) # show only filename instead of long absolute path tb_list = [row for row in tb_list if row[0] == "analysis.py"] if tb_list: msg += "Traceback:\n" msg += "".join(tb.format_list(tb_list)) # contains newlines msg += "{}: {}".format(e.__class__.__name__, str(e)) # Report failure result to grader, with stacktrace grader.add_result( GradeResult(outcome="failed", points=points_earned, msg=msg) ) raise else: # Test result: passed (no exceptions) grader.add_result( GradeResult(outcome="passed", points=points_earned, msg=None) ) if __name__ == "__main__": pytest.main(["-s", __file__])
I have activated the conda environment and set up the files so that it should be able to access the util.py file and the grading.py file.
I hope that after running the command, the analysis.py file will be graded using grade_analysis.py.
Correct answer
This is why using conda-lock lock files (or containerization) is better for long-term reproducibility than using yaml. Additional dependencies (such as more-itertools
) are not restricted in yaml, and dependencies of other packages may not have appropriate caps. In this case, the op ended up with a version of the more_itertools
module that referenced something that was only later added to functools
.
The dichotomy shows problematic references starting with more_itertools
v10 (to cached_property
), so setting a cap should do the trick:
name: ml4t channels: - conda-forge - defaults dependencies: - python=3.6 - cycler=0.10.0 - kiwisolver=1.1.0 - matplotlib=3.0.3 - more-itertools<10 # <- prevent v10+ - numpy=1.16.3 - pandas=0.24.2 - pyparsing=2.4.0 - python-dateutil=2.8.0 - pytz=2019.1 - scipy=1.2.1 - seaborn=0.9.0 - six=1.12.0 - joblib=0.13.2 - pytest=5.0 - pytest-json=0.4.0 - future=0.17.1 - pprofile=2.0.2 - pip - pip: - jsons==0.8.8 - gradescope-utils - subprocess32
Use this yaml and test that the import that caused the error now works:
$ python -c "from more_itertools.more import always_iterable" $ echo $? 0
The above is the detailed content of more_itertools cannot import cached_property from functools in Python 3.6. For more information, please follow other related articles on the PHP Chinese website!

Hot AI Tools

Undresser.AI Undress
AI-powered app for creating realistic nude photos

AI Clothes Remover
Online AI tool for removing clothes from photos.

Undress AI Tool
Undress images for free

Clothoff.io
AI clothes remover

AI Hentai Generator
Generate AI Hentai for free.

Hot Article

Hot Tools

Notepad++7.3.1
Easy-to-use and free code editor

SublimeText3 Chinese version
Chinese version, very easy to use

Zend Studio 13.0.1
Powerful PHP integrated development environment

Dreamweaver CS6
Visual web development tools

SublimeText3 Mac version
God-level code editing software (SublimeText3)

Hot Topics
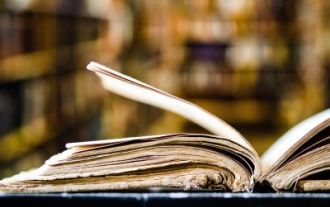
This tutorial demonstrates how to use Python to process the statistical concept of Zipf's law and demonstrates the efficiency of Python's reading and sorting large text files when processing the law. You may be wondering what the term Zipf distribution means. To understand this term, we first need to define Zipf's law. Don't worry, I'll try to simplify the instructions. Zipf's Law Zipf's law simply means: in a large natural language corpus, the most frequently occurring words appear about twice as frequently as the second frequent words, three times as the third frequent words, four times as the fourth frequent words, and so on. Let's look at an example. If you look at the Brown corpus in American English, you will notice that the most frequent word is "th
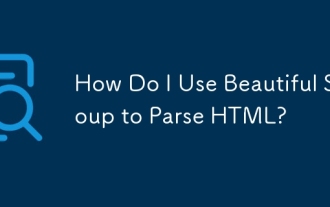
This article explains how to use Beautiful Soup, a Python library, to parse HTML. It details common methods like find(), find_all(), select(), and get_text() for data extraction, handling of diverse HTML structures and errors, and alternatives (Sel
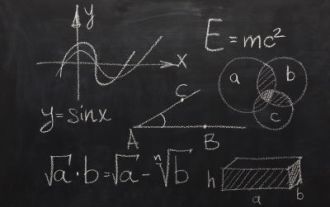
Python's statistics module provides powerful data statistical analysis capabilities to help us quickly understand the overall characteristics of data, such as biostatistics and business analysis. Instead of looking at data points one by one, just look at statistics such as mean or variance to discover trends and features in the original data that may be ignored, and compare large datasets more easily and effectively. This tutorial will explain how to calculate the mean and measure the degree of dispersion of the dataset. Unless otherwise stated, all functions in this module support the calculation of the mean() function instead of simply summing the average. Floating point numbers can also be used. import random import statistics from fracti
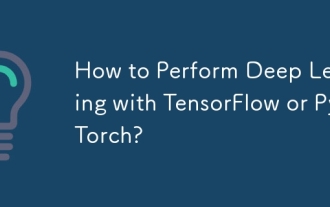
This article compares TensorFlow and PyTorch for deep learning. It details the steps involved: data preparation, model building, training, evaluation, and deployment. Key differences between the frameworks, particularly regarding computational grap
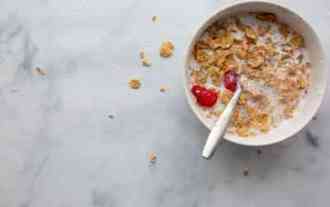
Serialization and deserialization of Python objects are key aspects of any non-trivial program. If you save something to a Python file, you do object serialization and deserialization if you read the configuration file, or if you respond to an HTTP request. In a sense, serialization and deserialization are the most boring things in the world. Who cares about all these formats and protocols? You want to persist or stream some Python objects and retrieve them in full at a later time. This is a great way to see the world on a conceptual level. However, on a practical level, the serialization scheme, format or protocol you choose may determine the speed, security, freedom of maintenance status, and other aspects of the program
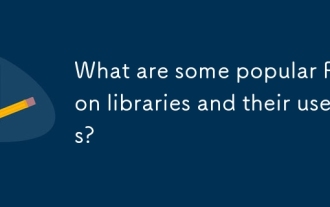
The article discusses popular Python libraries like NumPy, Pandas, Matplotlib, Scikit-learn, TensorFlow, Django, Flask, and Requests, detailing their uses in scientific computing, data analysis, visualization, machine learning, web development, and H
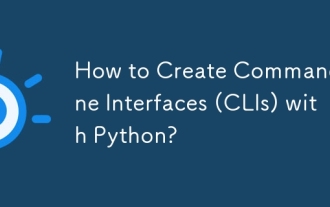
This article guides Python developers on building command-line interfaces (CLIs). It details using libraries like typer, click, and argparse, emphasizing input/output handling, and promoting user-friendly design patterns for improved CLI usability.
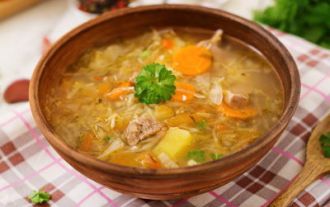
This tutorial builds upon the previous introduction to Beautiful Soup, focusing on DOM manipulation beyond simple tree navigation. We'll explore efficient search methods and techniques for modifying HTML structure. One common DOM search method is ex
