


Python GIL Alternative: Pushing the Limits of Multithreaded Programming
python The GIL (Global Interpreter Lock) is a tool used to prevent multiple threads from executing bytecode at the same time Mechanisms. It makes Pythoninterpreterthreadingsafe, but can also lead to poor multi-threaded programming performance. In order to break through the limitations of the GIL, a variety of alternatives have been proposed, some of which have been integrated into the Python interpreter, and others are provided as third-party libraries.
1. Limitations of GIL
Python GIL is a mutex lock used to ensure that only one thread can execute Python byte code at the same time. This prevents multiple threads from modifying the same object at the same time, causing data races. However, the GIL also has a negative impact on the performance of multithreaded programming. Because the GIL only allows one thread to execute byte code at the same time, other threads must wait in line, which may cause serious performance bottlenecks.
2. GIL alternatives
To address the limitations of GIL, various alternatives have been proposed. These solutions are mainly divided into two categories: one is integrated into the Python interpreter, and the other is provided as a third-party library.
1. GIL alternative integrated into the Python interpreter
Two GIL alternatives are integrated into the Python interpreter:
- Thread Local Storage (TLS): TLS allows each thread to have its own copy of local variables, thus avoiding competition for shared data. This can improve the performance of multi-threaded programming, but also increases memory usage.
- Concurrent programmingToolsPackage (concurrent.futures): The concurrent.futures module provides a series of tools for concurrent programming, including Thread pool and process pool. Thread pools can be used to manage the creation and destruction of threads, while process pools can be used to manage the creation and destruction of processes. Both tools can improve the performance of multi-threaded programming.
2. GIL alternatives provided by third-party libraries
In addition to GIL alternatives integrated into the Python interpreter, there are also some third-party libraries that provide GIL alternatives. These libraries include:
- Cython: Cython is a compiler that compiles Python code into C code. C code can be executed in parallel, so using Cython can improve the performance of Python multi-threaded programming.
- Numba: Numba is a compiler that compiles Python code into machine code. Machine code can also be executed in parallel, so using Numba can also improve the performance of multi-threaded programming in Python.
- PyPy: PyPy is an interpreter that implements the Python language. PyPy uses a different GIL implementation that improves the performance of multi-threaded programming.
3. Choose the appropriate GIL alternative
When choosing a GIL alternative, there are several factors to consider:
- Application Characteristics: Some GIL alternatives are better suited for certain types of applications. For example, TLS is better suited for applications with fewer data races, while concurrent programming toolkits are better suited for applications with more data races.
- Performance requirements of the application: Some GIL alternatives can provide higher performance, but may require more memory or more complex programming.
- Compatibility requirements for applications: Some GIL alternatives may be incompatible with certain Python libraries or
- frameworks .
4. Demonstration code
The following demo code shows how to use the concurrent.futures module to improve the performance of Python multi-threaded programming:
import concurrent.futures # 要执行的任务列表 tasks = [1, 2, 3, 4, 5] # 使用线程池执行任务 with concurrent.futures.ThreadPoolExecutor() as executor: # 使用map()方法并行执行任务 results = executor.map(lambda x: x * x, tasks) # 打印结果 print(results)
The above is the detailed content of Python GIL Alternative: Pushing the Limits of Multithreaded Programming. For more information, please follow other related articles on the PHP Chinese website!

Hot AI Tools

Undresser.AI Undress
AI-powered app for creating realistic nude photos

AI Clothes Remover
Online AI tool for removing clothes from photos.

Undress AI Tool
Undress images for free

Clothoff.io
AI clothes remover

AI Hentai Generator
Generate AI Hentai for free.

Hot Article

Hot Tools

Notepad++7.3.1
Easy-to-use and free code editor

SublimeText3 Chinese version
Chinese version, very easy to use

Zend Studio 13.0.1
Powerful PHP integrated development environment

Dreamweaver CS6
Visual web development tools

SublimeText3 Mac version
God-level code editing software (SublimeText3)

Hot Topics
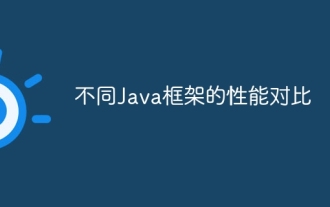
Performance comparison of different Java frameworks: REST API request processing: Vert.x is the best, with a request rate of 2 times SpringBoot and 3 times Dropwizard. Database query: SpringBoot's HibernateORM is better than Vert.x and Dropwizard's ORM. Caching operations: Vert.x's Hazelcast client is superior to SpringBoot and Dropwizard's caching mechanisms. Suitable framework: Choose according to application requirements. Vert.x is suitable for high-performance web services, SpringBoot is suitable for data-intensive applications, and Dropwizard is suitable for microservice architecture.
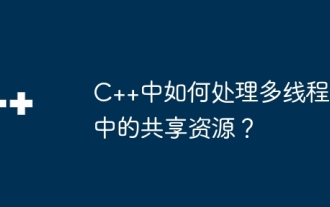
Mutexes are used in C++ to handle multi-threaded shared resources: create mutexes through std::mutex. Use mtx.lock() to obtain a mutex and provide exclusive access to shared resources. Use mtx.unlock() to release the mutex.
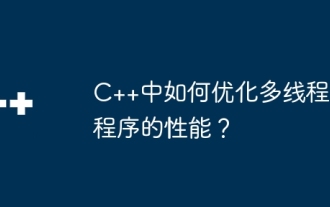
Effective techniques for optimizing C++ multi-threaded performance include limiting the number of threads to avoid resource contention. Use lightweight mutex locks to reduce contention. Optimize the scope of the lock and minimize the waiting time. Use lock-free data structures to improve concurrency. Avoid busy waiting and notify threads of resource availability through events.
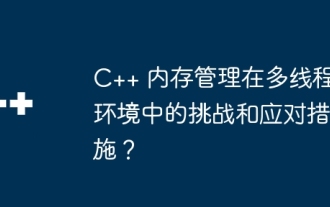
In a multi-threaded environment, C++ memory management faces the following challenges: data races, deadlocks, and memory leaks. Countermeasures include: 1. Use synchronization mechanisms, such as mutexes and atomic variables; 2. Use lock-free data structures; 3. Use smart pointers; 4. (Optional) implement garbage collection.
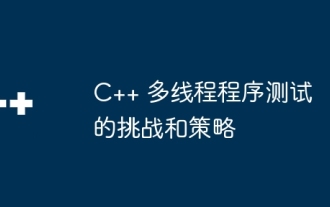
Multi-threaded program testing faces challenges such as non-repeatability, concurrency errors, deadlocks, and lack of visibility. Strategies include: Unit testing: Write unit tests for each thread to verify thread behavior. Multi-threaded simulation: Use a simulation framework to test your program with control over thread scheduling. Data race detection: Use tools to find potential data races, such as valgrind. Debugging: Use a debugger (such as gdb) to examine the runtime program status and find the source of the data race.
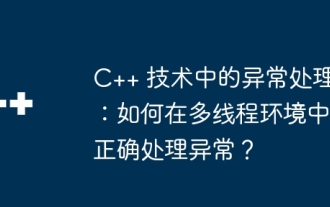
In multithreaded C++, exception handling follows the following principles: timeliness, thread safety, and clarity. In practice, you can ensure thread safety of exception handling code by using mutex or atomic variables. Additionally, consider reentrancy, performance, and testing of your exception handling code to ensure it runs safely and efficiently in a multi-threaded environment.
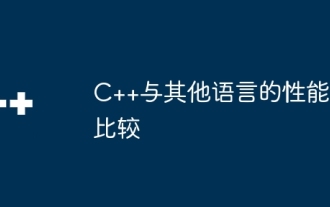
When developing high-performance applications, C++ outperforms other languages, especially in micro-benchmarks. In macro benchmarks, the convenience and optimization mechanisms of other languages such as Java and C# may perform better. In practical cases, C++ performs well in image processing, numerical calculations and game development, and its direct control of memory management and hardware access brings obvious performance advantages.
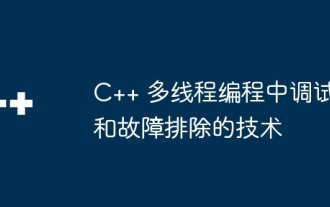
Debugging techniques for C++ multi-threaded programming include using a data race analyzer to detect read and write conflicts and using synchronization mechanisms (such as mutex locks) to resolve them. Use thread debugging tools to detect deadlocks and resolve them by avoiding nested locks and using deadlock detection mechanisms. Use the Data Race Analyzer to detect data races and resolve them by moving write operations into critical sections or using atomic operations. Use performance analysis tools to measure context switch frequency and resolve excessive overhead by reducing the number of threads, using thread pools, and offloading tasks.
