Explore the mutual benefits of generative AI and the cloud
It’s no coincidence that interest in generative artificial intelligence and cloud convergence has continued to grow in recent years. Generative artificial intelligence (AI) and cloud computing have revolutionized the IT industry, redefining industries and bringing unprecedented functionality to new technology tools. Let’s take a deeper look at the profound impact of generative AI on cloud computing, and how cloud computing empowers and enhances the capabilities of generative AI. The emergence of generative artificial intelligence has brought new opportunities and challenges to cloud computing. By combining generative artificial intelligence with cloud computing, enterprises can better utilize data resources, improve work efficiency, and accelerate innovation and development. Cloud computing provides efficient computing and storage resources for generative artificial intelligence, allowing it to handle complex tasks faster and at scale
The cloud unlocks the full power of generative AI for business use cases
The cloud provides several important enhancements to generative AI, especially in business use cases:
- Scalability: Generative AI models often require significant computing resources, especially during the training phase. Cloud platforms allow companies to scale up or down dynamically, allowing IT teams to allocate resources as needed. This scalability ensures that organizations can handle the computational demands of training generative AI models at scale without having to invest in expensive on-premises infrastructure if they don’t want to.
- Cost Effectiveness: Cloud computing uses a pay-as-you-go model, giving companies the options they want most. Instead of traditional processing stacks, which are rigid and sometimes waste resources and sometimes limit processing, companies can implement a more flexible approach. With the cloud, enterprises can provision resources on demand, thereby avoiding expensive hardware investments and reducing operating costs.
- Accessibility: The cloud democratizes access to generative AI capabilities, making them easier for businesses of all sizes to use. Companies can leverage cloud-based AI services and platforms instead of developing and maintaining their own infrastructure. This access levels the playing field for smaller companies without large AI teams or deep-pocketed IT investments. It could also allow companies of all sizes to start with small generative AI projects to see if they fit a specific project or business need.
- Collaboration and knowledge sharing: Creating and deploying generative AI projects often involves collaboration between data scientists, researchers, and engineers. Cloud platforms provide excellent collaboration tools, version control systems, and shared development environments that enable teams to work together seamlessly, rather than arguing about which version is the latest or losing important information in silos. Cloud-based services also enable easy code sharing, debugging, and project management, greatly accelerating the development and deployment of generative AI models.
- Data management: Generative artificial intelligence models require large amounts of training data. Cloud-based data storage and management solutions provide enterprises with the infrastructure to efficiently store, process and manage the massive data sets required for generative AI model training. With the cloud, organizations can leverage data lakes, data warehouses, and data pipelines to handle the storage, organization, and processing of training data so that all training data is of high enough quality and consistent enough to produce optimal results.
- Real-time inference: While training generative AI models may benefit from the abundant resources of the cloud, real-time inference typically requires low latency and immediate response. Cloud-based edge computing allows organizations to deploy trained generative AI models closer to the data source, reducing latency and enabling real-time decision-making. This is especially important in use cases such as real-time image or speech generation, where immediate response time is critical.
Generative AI automates and optimizes cloud operations
The connection between these two technologies is not one-way. Generative AI also has many advantages, such as optimizing cloud operations, improving performance, and enhancing enterprise user experience, which are its unique value.
- Increase efficiency and automation: Companies can leverage generative AI tools to automate and optimize various aspects of cloud operations, such as resource allocation, workload management, and system optimization. AI algorithms can analyze historical data, patterns and trends, leveraging truly large data sets to make intelligent decisions and dynamically allocate resources in the cloud. With cloud costs spiraling out of control for many organizations, this level of automation and control is a welcome way to manage costs without sacrificing performance.
- Intelligent resource allocation: Generative artificial intelligence models help companies shift from reactive to proactive actions by learning historical usage patterns to predict future resource needs. This gives enterprises the headroom and ability to proactively provision cloud resources based on forecasted workloads because the necessary infrastructure is already in place to handle anticipated demand and prevent resource shortages and over-provisioning.
- Enhanced Security and Threat Detection: Generative artificial intelligence algorithms can analyze large amounts of log data, network traffic, and system behavior to detect anomalies and potential security threats in real time. Enterprises can enhance their security posture by identifying and mitigating security risks, detecting intrusions, and improving incident response capabilities, ultimately protecting sensitive data and ensuring business continuity.
- Intelligent Monitoring and Predictive Maintenance: Generative AI can analyze system logs, performance metrics and historical data to identify patterns and detect early signs of potential system failure or performance degradation. By leveraging generative AI for monitoring and predictive maintenance in the cloud, enterprises can proactively resolve issues, reduce downtime, and optimize cloud infrastructure performance and reliability to ensure seamless operations and user satisfaction.
- Enhanced Service Personalization: Generative AI can analyze user behavior, preferences and contextual data to generate personalized recommendations, content or experiences. In cloud services, generative AI can tailor service offerings based on individual user needs, preferences or business requirements, providing a personalized and optimized cloud experience that meets specific business use cases and improves customer satisfaction.
- Automated troubleshooting and problem resolution: Generative AI models can be trained on a vast repository of troubleshooting data, system logs, and historical problem solutions. By applying generative AI technologies, businesses can automate troubleshooting processes, predict potential issues, and even provide automated solutions or recommendations, thereby reducing the time and effort required to resolve issues and improving overall operational efficiency.
Where should we go in the future?
The above is the detailed content of Explore the mutual benefits of generative AI and the cloud. For more information, please follow other related articles on the PHP Chinese website!

Hot AI Tools

Undresser.AI Undress
AI-powered app for creating realistic nude photos

AI Clothes Remover
Online AI tool for removing clothes from photos.

Undress AI Tool
Undress images for free

Clothoff.io
AI clothes remover

AI Hentai Generator
Generate AI Hentai for free.

Hot Article

Hot Tools

Notepad++7.3.1
Easy-to-use and free code editor

SublimeText3 Chinese version
Chinese version, very easy to use

Zend Studio 13.0.1
Powerful PHP integrated development environment

Dreamweaver CS6
Visual web development tools

SublimeText3 Mac version
God-level code editing software (SublimeText3)

Hot Topics
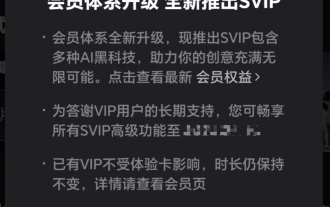
This site reported on June 27 that Jianying is a video editing software developed by FaceMeng Technology, a subsidiary of ByteDance. It relies on the Douyin platform and basically produces short video content for users of the platform. It is compatible with iOS, Android, and Windows. , MacOS and other operating systems. Jianying officially announced the upgrade of its membership system and launched a new SVIP, which includes a variety of AI black technologies, such as intelligent translation, intelligent highlighting, intelligent packaging, digital human synthesis, etc. In terms of price, the monthly fee for clipping SVIP is 79 yuan, the annual fee is 599 yuan (note on this site: equivalent to 49.9 yuan per month), the continuous monthly subscription is 59 yuan per month, and the continuous annual subscription is 499 yuan per year (equivalent to 41.6 yuan per month) . In addition, the cut official also stated that in order to improve the user experience, those who have subscribed to the original VIP
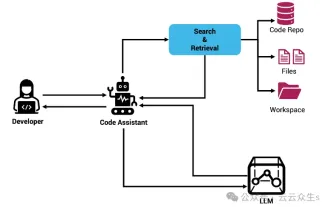
Improve developer productivity, efficiency, and accuracy by incorporating retrieval-enhanced generation and semantic memory into AI coding assistants. Translated from EnhancingAICodingAssistantswithContextUsingRAGandSEM-RAG, author JanakiramMSV. While basic AI programming assistants are naturally helpful, they often fail to provide the most relevant and correct code suggestions because they rely on a general understanding of the software language and the most common patterns of writing software. The code generated by these coding assistants is suitable for solving the problems they are responsible for solving, but often does not conform to the coding standards, conventions and styles of the individual teams. This often results in suggestions that need to be modified or refined in order for the code to be accepted into the application
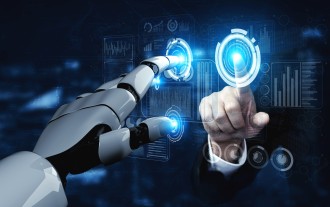
Large Language Models (LLMs) are trained on huge text databases, where they acquire large amounts of real-world knowledge. This knowledge is embedded into their parameters and can then be used when needed. The knowledge of these models is "reified" at the end of training. At the end of pre-training, the model actually stops learning. Align or fine-tune the model to learn how to leverage this knowledge and respond more naturally to user questions. But sometimes model knowledge is not enough, and although the model can access external content through RAG, it is considered beneficial to adapt the model to new domains through fine-tuning. This fine-tuning is performed using input from human annotators or other LLM creations, where the model encounters additional real-world knowledge and integrates it
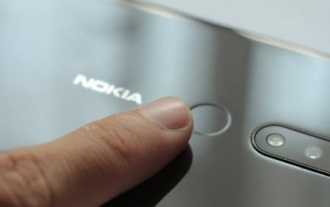
According to news from this site on July 31, technology giant Amazon sued Finnish telecommunications company Nokia in the federal court of Delaware on Tuesday, accusing it of infringing on more than a dozen Amazon patents related to cloud computing technology. 1. Amazon stated in the lawsuit that Nokia abused Amazon Cloud Computing Service (AWS) related technologies, including cloud computing infrastructure, security and performance technologies, to enhance its own cloud service products. Amazon launched AWS in 2006 and its groundbreaking cloud computing technology had been developed since the early 2000s, the complaint said. "Amazon is a pioneer in cloud computing, and now Nokia is using Amazon's patented cloud computing innovations without permission," the complaint reads. Amazon asks court for injunction to block
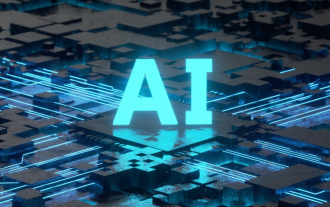
Editor |ScienceAI Question Answering (QA) data set plays a vital role in promoting natural language processing (NLP) research. High-quality QA data sets can not only be used to fine-tune models, but also effectively evaluate the capabilities of large language models (LLM), especially the ability to understand and reason about scientific knowledge. Although there are currently many scientific QA data sets covering medicine, chemistry, biology and other fields, these data sets still have some shortcomings. First, the data form is relatively simple, most of which are multiple-choice questions. They are easy to evaluate, but limit the model's answer selection range and cannot fully test the model's ability to answer scientific questions. In contrast, open-ended Q&A
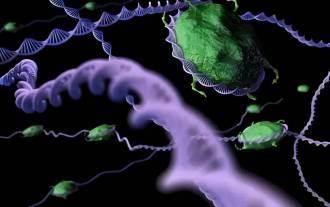
Editor | KX In the field of drug research and development, accurately and effectively predicting the binding affinity of proteins and ligands is crucial for drug screening and optimization. However, current studies do not take into account the important role of molecular surface information in protein-ligand interactions. Based on this, researchers from Xiamen University proposed a novel multi-modal feature extraction (MFE) framework, which for the first time combines information on protein surface, 3D structure and sequence, and uses a cross-attention mechanism to compare different modalities. feature alignment. Experimental results demonstrate that this method achieves state-of-the-art performance in predicting protein-ligand binding affinities. Furthermore, ablation studies demonstrate the effectiveness and necessity of protein surface information and multimodal feature alignment within this framework. Related research begins with "S
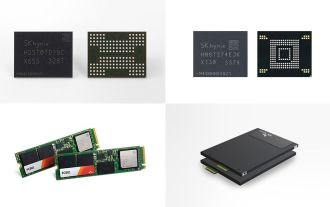
According to news from this site on August 1, SK Hynix released a blog post today (August 1), announcing that it will attend the Global Semiconductor Memory Summit FMS2024 to be held in Santa Clara, California, USA from August 6 to 8, showcasing many new technologies. generation product. Introduction to the Future Memory and Storage Summit (FutureMemoryandStorage), formerly the Flash Memory Summit (FlashMemorySummit) mainly for NAND suppliers, in the context of increasing attention to artificial intelligence technology, this year was renamed the Future Memory and Storage Summit (FutureMemoryandStorage) to invite DRAM and storage vendors and many more players. New product SK hynix launched last year
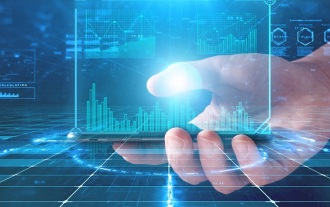
In the world of front-end development, VSCode has become the tool of choice for countless developers with its powerful functions and rich plug-in ecosystem. In recent years, with the rapid development of artificial intelligence technology, AI code assistants on VSCode have sprung up, greatly improving developers' coding efficiency. AI code assistants on VSCode have sprung up like mushrooms after a rain, greatly improving developers' coding efficiency. It uses artificial intelligence technology to intelligently analyze code and provide precise code completion, automatic error correction, grammar checking and other functions, which greatly reduces developers' errors and tedious manual work during the coding process. Today, I will recommend 12 VSCode front-end development AI code assistants to help you in your programming journey.
