Microsoft's 6-page paper explodes: ternary LLM, so delicious!
This is the conclusion put forward by Microsoft and the University of Chinese Academy of Sciences in the latest study-
All LLMs will be 1.58 bit.
Specifically, the method proposed in this study is called BitNet b1.58, which can be said to be "rooted in" from the large language model. "Start with the parameters on.
The traditional storage in the form of 16-bit floating point numbers (such as FP16 or BF16) has been changed into ternary , that is, {- 1, 0, 1}.
It should be noted that "1.58 bit" does not mean that each parameter occupies 1.58 bytes of storage space, but that each parameter can use 1.58 bits of information. coding.
After such conversion, the calculation in the matrix will only involve the addition of integers, thus allowing large models to significantly reduce the storage space required while maintaining a certain accuracy. and computing resources.
For example, BitNet b1.58 is compared with Llama when the model size is 3B. While the speed is increased by 2.71 times, the GPU memory usage is almost only a quarter of the original.
And when the size of the model is larger (for example, 70B) , the speed improvement and memory saving will be more significant!
This subversive idea of tradition really makes netizens shine. The paper also received high attention on X:
Netizens were amazed While "changing the rules of the game", it also played up the old joke of Google's attention paper:
1 bit is all YOU need.
So how is BitNet b1.58 implemented? Let's continue reading.
Change the parameters into ternary
This research is actually an optimization done by the original team based on a previously published paper, that is, adding additional data to the original BitNet An extra value of 0 is added.
Overall, BitNet b1.58 is still based on the BitNet architecture (a Transformer) , replacing nn.Linear with BitLinear.
As for the detailed optimization, the first thing is the "adding a 0" we just mentioned, that is, weight quantization(weight quantization).
The weights of the BitNet b1.58 model are quantized into ternary values {-1, 0, 1}, which is equivalent to using 1.58 bits to represent each weight in the binary system. This quantification method reduces the memory footprint of the model and simplifies the calculation process.
Secondly, in terms of quantitative function design, in order to limit the weight to -1, 0 or 1, the researchers adopted a A quantification function called absmean.
This function first scales according to the average absolute value of the weight matrix, and then rounds each value to the nearest integer (-1, 0, 1).
The next step is activation quantization(activation quantization).
The quantization of activation values is the same as the implementation in BitNet, but the activation values are not scaled to the range [0, Qb] before the nonlinear function. Instead, the activations are scaled to the range [−Qb, Qb] to eliminate zero-point quantization.
It is worth mentioning that in order to make BitNet b1.58 compatible with the open source community, the research team adopted components of the LLaMA model, such as RMSNorm, SwiGLU, etc., so that it can be easily integrated into mainstream open source software.
Finally, in terms of experimental performance comparison, the team compared BitNet b1.58 and FP16 LLaMA LLM on models of different sizes.
The results show that BitNet b1.58 starts to match the full-precision LLaMA LLM in perplexity at 3B model size, while having better performance in latency, memory usage and throughput. Significantly improved.
And when the model size becomes larger, this performance improvement will become more significant.
Netizen: Can run 120B large model on consumer-grade GPU
As mentioned above, the unique method of this study has caused a lot of heated discussion on the Internet.
DeepLearning.scala author Yang Bo said:
Compared with the original BitNet, the biggest feature of BitNet b1.58 is that it allows 0 parameters. I think that by slightly modifying the quantization function, we may be able to control the proportion of 0 parameters. When the proportion of 0 parameters is large, the weights can be stored in a sparse format, so that the average video memory occupied by each parameter is even less than 1 bit. This is equivalent to a weight-level MoE. I think it's more elegant than regular MoE.
At the same time, he also raised the shortcomings of BitNet:
The biggest shortcoming of BitNet is that although it can reduce the memory overhead during inference, the optimizer state and gradient still use floating point numbers. , training is still very memory intensive. I think if BitNet can be combined with technology that saves video memory during training, then compared to traditional half-precision networks, it can support more parameters with the same computing power and video memory, which will have great advantages.
The current way to save the graphics memory overhead of the optimizer state is offloading. A way to save the memory usage of gradients may be ReLoRA. However, the ReLoRA paper experiment only used a model with one billion parameters, and there is no evidence that it can be generalized to models with tens or hundreds of billions of parameters.
If the paper is established, Then we can run a 120B large model on a 24GB consumer-grade GPU.
The above is the detailed content of Microsoft's 6-page paper explodes: ternary LLM, so delicious!. For more information, please follow other related articles on the PHP Chinese website!

Hot AI Tools

Undresser.AI Undress
AI-powered app for creating realistic nude photos

AI Clothes Remover
Online AI tool for removing clothes from photos.

Undress AI Tool
Undress images for free

Clothoff.io
AI clothes remover

AI Hentai Generator
Generate AI Hentai for free.

Hot Article

Hot Tools

Notepad++7.3.1
Easy-to-use and free code editor

SublimeText3 Chinese version
Chinese version, very easy to use

Zend Studio 13.0.1
Powerful PHP integrated development environment

Dreamweaver CS6
Visual web development tools

SublimeText3 Mac version
God-level code editing software (SublimeText3)

Hot Topics


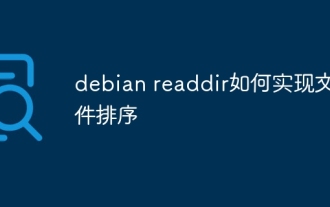
In Debian systems, the readdir function is used to read directory contents, but the order in which it returns is not predefined. To sort files in a directory, you need to read all files first, and then sort them using the qsort function. The following code demonstrates how to sort directory files using readdir and qsort in Debian system: #include#include#include#include#include//Custom comparison function, used for qsortintcompare(constvoid*a,constvoid*b){returnstrcmp(*(
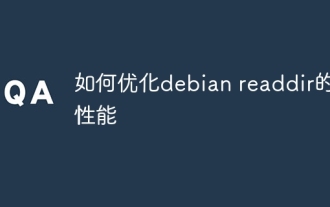
In Debian systems, readdir system calls are used to read directory contents. If its performance is not good, try the following optimization strategy: Simplify the number of directory files: Split large directories into multiple small directories as much as possible, reducing the number of items processed per readdir call. Enable directory content caching: build a cache mechanism, update the cache regularly or when directory content changes, and reduce frequent calls to readdir. Memory caches (such as Memcached or Redis) or local caches (such as files or databases) can be considered. Adopt efficient data structure: If you implement directory traversal by yourself, select more efficient data structures (such as hash tables instead of linear search) to store and access directory information
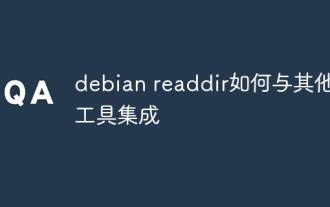
The readdir function in the Debian system is a system call used to read directory contents and is often used in C programming. This article will explain how to integrate readdir with other tools to enhance its functionality. Method 1: Combining C language program and pipeline First, write a C program to call the readdir function and output the result: #include#include#include#includeintmain(intargc,char*argv[]){DIR*dir;structdirent*entry;if(argc!=2){
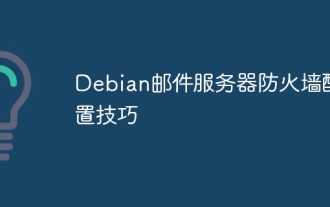
Configuring a Debian mail server's firewall is an important step in ensuring server security. The following are several commonly used firewall configuration methods, including the use of iptables and firewalld. Use iptables to configure firewall to install iptables (if not already installed): sudoapt-getupdatesudoapt-getinstalliptablesView current iptables rules: sudoiptables-L configuration
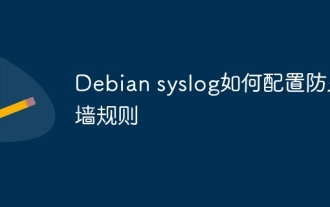
This article describes how to configure firewall rules using iptables or ufw in Debian systems and use Syslog to record firewall activities. Method 1: Use iptablesiptables is a powerful command line firewall tool in Debian system. View existing rules: Use the following command to view the current iptables rules: sudoiptables-L-n-v allows specific IP access: For example, allow IP address 192.168.1.100 to access port 80: sudoiptables-AINPUT-ptcp--dport80-s192.16
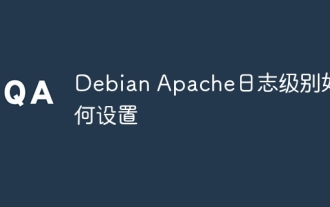
This article describes how to adjust the logging level of the ApacheWeb server in the Debian system. By modifying the configuration file, you can control the verbose level of log information recorded by Apache. Method 1: Modify the main configuration file to locate the configuration file: The configuration file of Apache2.x is usually located in the /etc/apache2/ directory. The file name may be apache2.conf or httpd.conf, depending on your installation method. Edit configuration file: Open configuration file with root permissions using a text editor (such as nano): sudonano/etc/apache2/apache2.conf
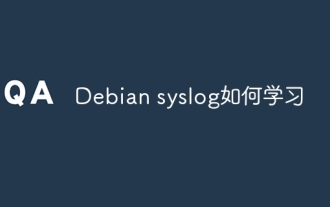
This guide will guide you to learn how to use Syslog in Debian systems. Syslog is a key service in Linux systems for logging system and application log messages. It helps administrators monitor and analyze system activity to quickly identify and resolve problems. 1. Basic knowledge of Syslog The core functions of Syslog include: centrally collecting and managing log messages; supporting multiple log output formats and target locations (such as files or networks); providing real-time log viewing and filtering functions. 2. Install and configure Syslog (using Rsyslog) The Debian system uses Rsyslog by default. You can install it with the following command: sudoaptupdatesud
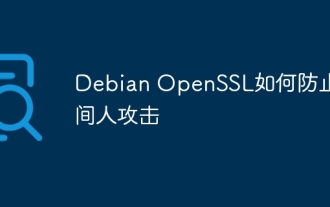
In Debian systems, OpenSSL is an important library for encryption, decryption and certificate management. To prevent a man-in-the-middle attack (MITM), the following measures can be taken: Use HTTPS: Ensure that all network requests use the HTTPS protocol instead of HTTP. HTTPS uses TLS (Transport Layer Security Protocol) to encrypt communication data to ensure that the data is not stolen or tampered during transmission. Verify server certificate: Manually verify the server certificate on the client to ensure it is trustworthy. The server can be manually verified through the delegate method of URLSession
