


New work by Chen Danqi's team: Llama-2 context is expanded to 128k, 10 times throughput only requires 1/6 of the memory
The Chen Danqi team has just released a new LLMContext window extensionMethod:
It only uses 8k token documents for training, and can Llama-2 Window extended to 128k.
The most important thing is that in this process, the model only requires 1/6 of the original memory, and the model obtains 10 times the throughput.
In addition, it can also greatly reduce the training cost:
Use this method to train 7B alpaca 2 For transformation, you only need a piece of A100 to complete it.
The team expressed:We hope this method will be useful and easy to use, and provideCurrently, the model and code have been released on HuggingFace and GitHub.cheap and effective long context capabilities for future LLMs.
CEPE, the full name is "Parallel Encoding Context Extension(Context Expansion with Parallel Encoding)”.
As a lightweight framework, it can be used to extend the context window of anypre-trained and directive fine-tuning model.
For any pretrained decoder-only language model, CEPE extends it by adding two small components:One is a small encoder for long The context is block-encoded;
One is the cross-attention module, which is inserted into each layer of the decoder to focus on the encoder representation.
The complete architecture is as follows:(1) The length can be generalized
because it is not subject to positional encoding A constraint, instead, has its context encoded in segments, each segment having its own positional encoding.(2) High efficiencyUsing small encoders and parallel encoding to process context can reduce computational costs.
(3) Reduce training cost
with a 400M encoder and cross-attention layer (a total of 1.4 billion parameters), it can be completed with an 80GB A100 GPU.
Perplexity continues to decreaseThe team applies CEPE to Llama-2 and trains on the filtered version of RedPajama with 20 billion tokens(only the Llama-2 pre-training budget 1%).
First, compared to two fully fine-tuned models, LLAMA2-32K and YARN-64K, CEPE achieves lower or comparableperplexity## on all datasets. #, with both lower memory usage and higher throughput.
When the context is increased to 128k
, CEPE’s perplexity continues to decrease while remaining low memory status. In contrast, Llama-2-32K and YARN-64K not only fail to generalize beyond their training length, but are also accompanied by a significant increase in memory cost.
Secondly,
retrieval capability is enhanced. As shown in the following table:
By using the retrieved context, CEPE can effectively improve model perplexity, and its performance is better than RePlug.
It is worth noting that even if paragraph k=50 (training is 60), CEPE will continue to improve the perplexity.
This shows that CEPE transfers well to the retrieval enhancement setting, whereas the full-context decoder model degrades in this ability.
Third, open domain question and answer capabilitiesare significantly surpassed.
As shown in the figure below, CEPE is significantly better than other models in all data sets and paragraph k parameters, and unlike other models, the performance drops significantly as the k value becomes larger and larger.
This also shows that CEPE is not sensitive to a large number of redundant or irrelevant paragraphs.
So to summarize, CEPE outperforms on all the above tasks with much lower memory and computational cost compared to most other solutions.
Finally, based on these, the author proposed CEPE-Distilled (CEPED) specifically for the instruction tuning model.
It uses only unlabeled data to expand the context window of the model, distilling the behavior of the original instruction-tuned model into a new architecture through assisted KL divergence loss, thereby eliminating the need to manage expensive long context instruction tracking data.
Ultimately, CEPED can expand the context window of Llama-2 and improve the long text performance of the model while retaining the ability to understand instructions.
Team Introduction
CEPE has a total of 3 authors.
Yan Heguang(Howard Yen) is a master's student in computer science at Princeton University.
The second person is Gao Tianyu, a doctoral student at the same school and a bachelor's degree graduate from Tsinghua University.
They are all students of the corresponding author Chen Danqi.
Original paper: https://arxiv.org/abs/2402.16617
Reference link: https://twitter. com/HowardYen1/status/1762474556101661158
The above is the detailed content of New work by Chen Danqi's team: Llama-2 context is expanded to 128k, 10 times throughput only requires 1/6 of the memory. For more information, please follow other related articles on the PHP Chinese website!

Hot AI Tools

Undresser.AI Undress
AI-powered app for creating realistic nude photos

AI Clothes Remover
Online AI tool for removing clothes from photos.

Undress AI Tool
Undress images for free

Clothoff.io
AI clothes remover

AI Hentai Generator
Generate AI Hentai for free.

Hot Article

Hot Tools

Notepad++7.3.1
Easy-to-use and free code editor

SublimeText3 Chinese version
Chinese version, very easy to use

Zend Studio 13.0.1
Powerful PHP integrated development environment

Dreamweaver CS6
Visual web development tools

SublimeText3 Mac version
God-level code editing software (SublimeText3)

Hot Topics
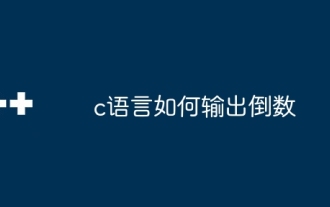
How to output a countdown in C? Answer: Use loop statements. Steps: 1. Define the variable n and store the countdown number to output; 2. Use the while loop to continuously print n until n is less than 1; 3. In the loop body, print out the value of n; 4. At the end of the loop, subtract n by 1 to output the next smaller reciprocal.
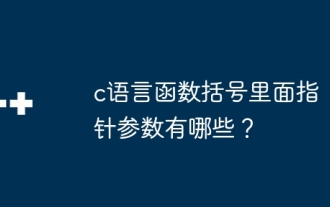
The pointer parameters of C language function directly operate the memory area passed by the caller, including pointers to integers, strings, or structures. When using pointer parameters, you need to be careful to modify the memory pointed to by the pointer to avoid errors or memory problems. For double pointers to strings, modifying the pointer itself will lead to pointing to new strings, and memory management needs to be paid attention to. When handling pointer parameters to structures or arrays, you need to carefully check the pointer type and boundaries to avoid out-of-bounds access.
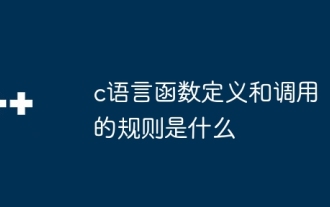
A C language function consists of a parameter list, function body, return value type and function name. When a function is called, the parameters are copied to the function through the value transfer mechanism, and will not affect external variables. Pointer passes directly to the memory address, modifying the pointer will affect external variables. Function prototype declaration is used to inform the compiler of function signatures to avoid compilation errors. Stack space is used to store function local variables and parameters. Too much recursion or too much space can cause stack overflow.
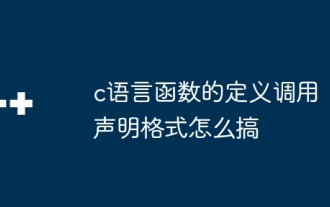
C language functions include definitions, calls and declarations. Function definition specifies function name, parameters and return type, function body implements functions; function calls execute functions and provide parameters; function declarations inform the compiler of function type. Value pass is used for parameter pass, pay attention to the return type, maintain a consistent code style, and handle errors in functions. Mastering this knowledge can help write elegant, robust C code.
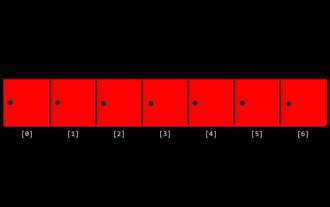
Algorithms are the set of instructions to solve problems, and their execution speed and memory usage vary. In programming, many algorithms are based on data search and sorting. This article will introduce several data retrieval and sorting algorithms. Linear search assumes that there is an array [20,500,10,5,100,1,50] and needs to find the number 50. The linear search algorithm checks each element in the array one by one until the target value is found or the complete array is traversed. The algorithm flowchart is as follows: The pseudo-code for linear search is as follows: Check each element: If the target value is found: Return true Return false C language implementation: #include#includeintmain(void){i
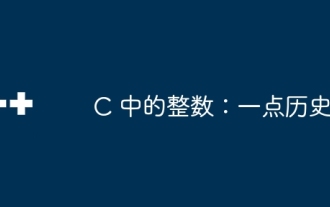
Integers are the most basic data type in programming and can be regarded as the cornerstone of programming. The job of a programmer is to give these numbers meanings. No matter how complex the software is, it ultimately comes down to integer operations, because the processor only understands integers. To represent negative numbers, we introduced two's complement; to represent decimal numbers, we created scientific notation, so there are floating-point numbers. But in the final analysis, everything is still inseparable from 0 and 1. A brief history of integers In C, int is almost the default type. Although the compiler may issue a warning, in many cases you can still write code like this: main(void){return0;} From a technical point of view, this is equivalent to the following code: intmain(void){return0;}
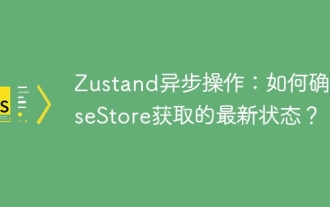
Data update problems in zustand asynchronous operations. When using the zustand state management library, you often encounter the problem of data updates that cause asynchronous operations to be untimely. �...
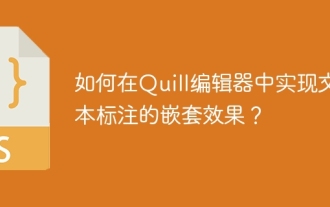
A solution to implement text annotation nesting in Quill Editor. When using Quill Editor for text annotation, we often need to use the Quill Editor to...
