


The technical details of the Byte Wanka cluster are disclosed: GPT-3 training was completed in 2 days, and the computing power utilization exceeded NVIDIA Megatron-LM
As the technical analysis of Sora unfolds, the importance of AI infrastructure becomes increasingly prominent.
A new paper from Byte and Peking University attracted attention at this time:
The article disclosed that the Wanka cluster built by Byte can be used in ## Complete the training of GPT-3 scale model (175B) within #1.75 days.
MegaScale, which aims to solve the problems faced when training large models on the Wanka cluster. efficiency and stability challenges.
When training a 175 billion parameter large language model on 12288 GPUs, MegaScale achieved a computing power utilization of 55.2%(MFU) , which is 1.34 times that of NVIDIA Megatron-LM.
The paper also revealed that as of September 2023, Byte has established an Ampere architecture GPU(A100/A800) cluster with more than 10,000 cards, and is currently building a large-scale Hopper architecture (H100/H800)Cluster.
Production system suitable for Wanka clusterIn the era of large models, the importance of GPU no longer needs elaboration. But the training of large models cannot be started directly when the number of cards is full - when the scale of the GPU cluster reaches the "10,000" level, how to achieveefficiency and stability# The training of ## is itself a challenging engineering problem.
The first challenge: efficiency.
Training a large language model is not a simple parallel task. The model needs to be distributed among multiple GPUs, and these GPUs require frequent communication to jointly advance the training process. In addition to communication, factors such as operator optimization, data preprocessing and GPU memory consumption all have an impact on computing power utilization
(MFU), an indicator that measures training efficiency.
MFU is the ratio of actual throughput to theoretical maximum throughput.The second challenge: stability.
We know that training large language models often takes a very long time, which also means that failures and delays during the training process are not uncommon.
The cost of failure is high, so how to shorten the fault recovery time becomes particularly important.
In order to address these challenges, ByteDance researchers built MegaScale and have deployed it in Byte's data center to support the training of various large models.
MegaScale is improved on the basis of NVIDIA Megatron-LM.
# Specific improvements include the co-design of algorithms and system components, optimization of communication and computational overlap, operator optimization, data pipeline optimization, and network performance Tuning, etc.:
- Algorithm optimization: Researchers introduced parallelized Transformer block, sliding window attention mechanism(SWA) and LAMB in the model architecture Optimizer to improve training efficiency without sacrificing model convergence.
- Communication overlap: Based on a detailed analysis of the operations of each computing unit in 3D parallelism(data parallelism, pipeline parallelism, tensor parallelism) , the researchers designed technical strategies to effectively reduce the delay caused by operations on non-critical execution paths and shorten the iteration time of each round in model training.
- Efficient operators: The GEMM operator has been optimized, and operations such as LayerNorm and GeLU have been integrated to reduce the overhead of starting multiple cores and optimize memory access patterns.
- Data pipeline optimization: Optimize data preprocessing and loading and reduce GPU idle time through asynchronous data preprocessing and elimination of redundant data loaders.
- Collective communication group initialization: Optimized the initialization process of NVIDIA multi-card communication framework NCCL in distributed training. Without optimization, the initialization time of a 2048-GPU cluster is 1047 seconds, which can be reduced to less than 5 seconds after optimization; the initialization time of a Wanka GPU cluster can be reduced to less than 30 seconds.
- Network Performance Tuning: Analyze inter-machine traffic in 3D parallelism, and design technical solutions to improve network performance, including network topology design, ECMP hash conflict reduction, and congestion control and retransmission timeout settings.
- Fault Tolerance: In Wanka cluster, software and hardware failures are unavoidable. The researchers designed a training framework to achieve automatic fault identification and rapid recovery. Specifically, it includes developing diagnostic tools to monitor system components and events, optimizing checkpoint high-frequency saving training processes, etc.
The paper mentioned that MegaScale can automatically detect and repair more than 90% of software and hardware failures.
Experimental results show that MegaScale achieved 55.2% MFU when training a 175B large language model on 12288 GPUs, which is the Megatrion-LM algorithm 1.34 times the power utilization rate.
The MFU comparison results of training a 530B large language model are as follows:
One More Thing
Just after this technical paper triggered a discussion Recently, new news has also come out about the byte-based Sora product:
Jiangying's Sora-like AI video tool has launched an invitation-only beta test.
#It seems that the foundation has been laid, so are you looking forward to Byte's large model products?
Paper address: https://arxiv.org/abs/2402.15627
The above is the detailed content of The technical details of the Byte Wanka cluster are disclosed: GPT-3 training was completed in 2 days, and the computing power utilization exceeded NVIDIA Megatron-LM. For more information, please follow other related articles on the PHP Chinese website!

Hot AI Tools

Undresser.AI Undress
AI-powered app for creating realistic nude photos

AI Clothes Remover
Online AI tool for removing clothes from photos.

Undress AI Tool
Undress images for free

Clothoff.io
AI clothes remover

AI Hentai Generator
Generate AI Hentai for free.

Hot Article

Hot Tools

Notepad++7.3.1
Easy-to-use and free code editor

SublimeText3 Chinese version
Chinese version, very easy to use

Zend Studio 13.0.1
Powerful PHP integrated development environment

Dreamweaver CS6
Visual web development tools

SublimeText3 Mac version
God-level code editing software (SublimeText3)

Hot Topics
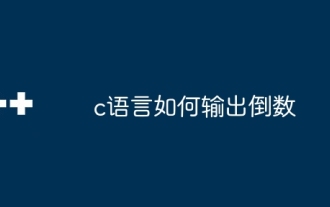
How to output a countdown in C? Answer: Use loop statements. Steps: 1. Define the variable n and store the countdown number to output; 2. Use the while loop to continuously print n until n is less than 1; 3. In the loop body, print out the value of n; 4. At the end of the loop, subtract n by 1 to output the next smaller reciprocal.
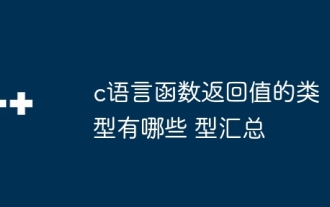
The return value types of C language function include int, float, double, char, void and pointer types. int is used to return integers, float and double are used to return floats, and char returns characters. void means that the function does not return any value. The pointer type returns the memory address, be careful to avoid memory leakage.结构体或联合体可返回多个相关数据。
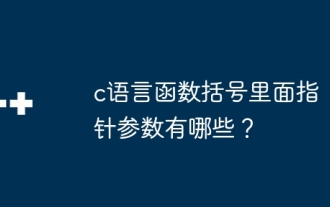
The pointer parameters of C language function directly operate the memory area passed by the caller, including pointers to integers, strings, or structures. When using pointer parameters, you need to be careful to modify the memory pointed to by the pointer to avoid errors or memory problems. For double pointers to strings, modifying the pointer itself will lead to pointing to new strings, and memory management needs to be paid attention to. When handling pointer parameters to structures or arrays, you need to carefully check the pointer type and boundaries to avoid out-of-bounds access.
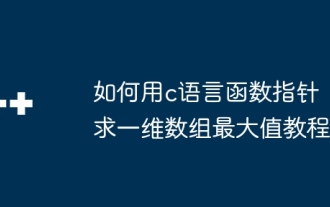
Flexible application of function pointers: use comparison functions to find the maximum value of an array. First, define the comparison function type CompareFunc, and then write the comparison function compareMax(a, b). The findMax function accepts array, array size, and comparison function parameters, and uses the comparison function to loop to compare array elements to find the maximum value. This method has strong code reusability, reflects the idea of higher-order programming, and is conducive to solving more complex problems.
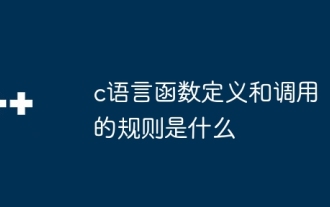
A C language function consists of a parameter list, function body, return value type and function name. When a function is called, the parameters are copied to the function through the value transfer mechanism, and will not affect external variables. Pointer passes directly to the memory address, modifying the pointer will affect external variables. Function prototype declaration is used to inform the compiler of function signatures to avoid compilation errors. Stack space is used to store function local variables and parameters. Too much recursion or too much space can cause stack overflow.
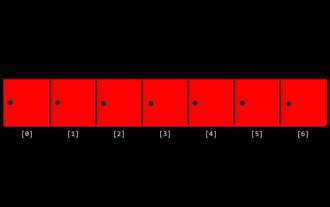
Algorithms are the set of instructions to solve problems, and their execution speed and memory usage vary. In programming, many algorithms are based on data search and sorting. This article will introduce several data retrieval and sorting algorithms. Linear search assumes that there is an array [20,500,10,5,100,1,50] and needs to find the number 50. The linear search algorithm checks each element in the array one by one until the target value is found or the complete array is traversed. The algorithm flowchart is as follows: The pseudo-code for linear search is as follows: Check each element: If the target value is found: Return true Return false C language implementation: #include#includeintmain(void){i
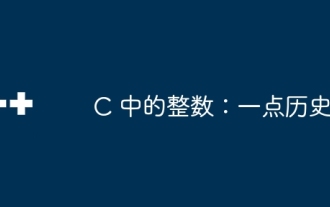
Integers are the most basic data type in programming and can be regarded as the cornerstone of programming. The job of a programmer is to give these numbers meanings. No matter how complex the software is, it ultimately comes down to integer operations, because the processor only understands integers. To represent negative numbers, we introduced two's complement; to represent decimal numbers, we created scientific notation, so there are floating-point numbers. But in the final analysis, everything is still inseparable from 0 and 1. A brief history of integers In C, int is almost the default type. Although the compiler may issue a warning, in many cases you can still write code like this: main(void){return0;} From a technical point of view, this is equivalent to the following code: intmain(void){return0;}
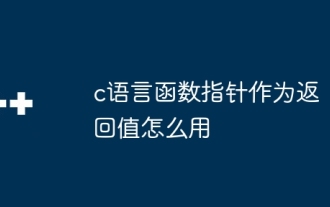
Function pointers can be used as return values to implement the mechanism of returning different functions according to different inputs. By defining the function type and returning the corresponding function pointer according to the selection, you can dynamically call functions, enhancing the flexibility of the code. However, pay attention to the definition of function pointer types, exception handling and memory management to ensure the robustness of the code.
