A little bit about the implementation of BEV lane lines
A seed was planted in 2021
Students who have read the BEV obstacle story should know that our group started doing BEV obstacles around October 21 material. At that time, I didn’t dare to think about doing BEV lane markings because there was no manpower. But I remember that around December, we met with a candidate. During the interview, we heard that they had been working on BEV lane markings for more than half a year. The entire technical route was used as a BEV lane marking network through high-precision maps. Train the true value and say that the effect is not bad. Unfortunately, that candidate did not come to us in the end. Combined with the content of lane markings taught at Telsa AI day in 2021, the seed of making BEV lane markings was planted in the group.
Taking the right first step in 2022
Throughout 2022, the manpower in our team was very tight. I remember that in June and July, We just have the manpower to explore the BEV lane lines. But at that time, there was only one classmate in our group (let’s call him Xiaoxuan for now) who had 2 months to do this. Then the seeds of 2021 began to sprout. We were going to start with the data. Student Xiaoxuan was still very good (very imaginative, and Xiaoxuan also made more things that surprised everyone in the future), and it was almost used. In February, we can extract lane line data around the corresponding car through high-speed high-precision maps. When it was made, I remember everyone was still very excited.
Figure 1: The effect of high-precision map lane lines projected onto the image system
As you can see from Figure 1, there are still some fitting problems problem, so Xiaoxuan made a series of optimizations. Two months later, Xiaoxuan went to do other tasks. Looking back now, we have taken the right step in exploring BEV lane lines. Because in 2021 and 22, many excellent BEV lane line papers and codes have been gradually open sourced. Seeing this, you may think that there must be a perfect story about the implementation of BEV lane lines in 2023. However, ideals are often very fulfilling, but the reality is very cruel.
23 years of stumbling
Because our BEV obstacles have proven that BEV can go down this road, and it has also shown good results in road tests. The group began to have more resources to consider lane lines. Note that this is not BEV. why? Because at this time, we were facing a lot of pressure to go online, and we did not have enough experience in BEV lane lines. In other words, there were almost no people in the entire group who had done mass production of 2D lane lines. In the first half of 2023, it can really be described as stumbling. We had many heated discussions internally, and finally decided to form two lines, one of which is the 2D lane line: most of the manpower is on the 2D lane line, and the focus is on the 2D lane line. Post-processing, light model, and accumulation of lane line post-processing mass production experience through 2D lane lines. One line is the BEV lane line: there is only a small number of manpower (actually only 1-2 manpower), focusing on the model design of the BEV lane line and accumulating model experience. There are already many BEV lane marking networks. I will post two papers that have a great impact on us here for your reference. "HDMapNet: An Online HD Map Construction and Evaluation Framework" and "MapTR: Structured Modeling and Learning for Online Vectorized HD Map Construction"
However, a story often has twists and turns. We can only use high-speed high-precision maps to produce lane line data. What to do about the city? There are still so many bad cases that need to be solved. At this time, the important person will finally appear. Let's call him Classmate Xiaotang (the big steward of our data group). Classmate Xiaotang and the others used point cloud reconstruction to reconstruct the clip for us ( This process was quite painful. I remember those two months were the most stressful time for them, haha. Of course, classmate Xiaotang and we often fell in love and killed each other. , after all, I often say that there is no data again during meetings. ). Then how to label after reconstruction? Looking at the suppliers at the time, none of them had such labeling tools, let alone labeling experience. Together with Xiaotang and others, after a long month, the annotation tool was finally polished with the supplier. (We often joke that we are empowering the entire self-driving annotation industry. This process is really painful, and rebuilding clips is really slow to load). However, the whole labeling is still relatively slow or expensive. At this time, Xiaoxuan made his debut with his large model of lane line pre-labeling (the effect of the large model of lane line pre-labeling is still outstanding), and everyone looked at him with amazement. In sparkling. After this set of combinations, our lane line data production is finally almost ready. In August, our BEV lane line control lane line has been iterated well, which is suitable for simple high-speed piloting functions. Now Xiaoxuan is still bringing us more surprises in the pre-marked direction of the large model. We and Xiaotang are still in love with each other.
However, a story does not end so easily. In September, we started working on multi-modal (Lidar, camera, Radar) multi-task (lane lines, obstacles, Occ) pre-fusion models. It will also subsequently support City Navigation (NCP), a so-called solution that emphasizes perception and ignores maps. Based on the experience of BEV obstacles and BEV lane lines, we will soon deploy the converged network on vehicles, probably by the end of September. Many subtasks have also been added to lane lines, such as road sign recognition, intersection topology, etc. In this process, we upgraded the post-processing of BEV lane lines, abandoned the lane line cubic spline fitting, and adopted a point tracking scheme. The output of the point tracking scheme and our lane line model can be easily Good combination. This process was also painful. We held a special meeting once a week for 2 consecutive months. After all, we have done well based on the fitting plan, but in order to reach a higher limit, we can only suffer from pain and happiness. Finally, we have already put the basic functions into road testing.
Let me briefly explain Figure 4. The left side is the effect of lane line point tracking. Currently, the perception range of our model is only the first 80 meters. You can see that there are some points behind the car, which are left by tracking. On the right is the real-time perceptual map we have established. Of course, it is still in a rapid iteration process, and there are still many problems being solved.
The new beginning of 24 years
At this moment, standing in 24 years and looking back at our growth and accumulation from 21 years to now, I am very fortunate to be at that point in 21 years , I have the opportunity to do BEV, and I am very fortunate to have a group of like-minded friends who can help each other along the way. In 24 years, there are many things for us to pursue, including the mass production of pre-fusion models, efforts in data direction, exploration of timing models, end-to-end imagination, etc.
The above is the detailed content of A little bit about the implementation of BEV lane lines. For more information, please follow other related articles on the PHP Chinese website!

Hot AI Tools

Undresser.AI Undress
AI-powered app for creating realistic nude photos

AI Clothes Remover
Online AI tool for removing clothes from photos.

Undress AI Tool
Undress images for free

Clothoff.io
AI clothes remover

Video Face Swap
Swap faces in any video effortlessly with our completely free AI face swap tool!

Hot Article

Hot Tools

Notepad++7.3.1
Easy-to-use and free code editor

SublimeText3 Chinese version
Chinese version, very easy to use

Zend Studio 13.0.1
Powerful PHP integrated development environment

Dreamweaver CS6
Visual web development tools

SublimeText3 Mac version
God-level code editing software (SublimeText3)

Hot Topics


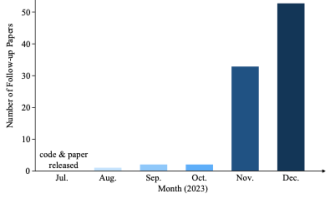
Written above & the author’s personal understanding Three-dimensional Gaussiansplatting (3DGS) is a transformative technology that has emerged in the fields of explicit radiation fields and computer graphics in recent years. This innovative method is characterized by the use of millions of 3D Gaussians, which is very different from the neural radiation field (NeRF) method, which mainly uses an implicit coordinate-based model to map spatial coordinates to pixel values. With its explicit scene representation and differentiable rendering algorithms, 3DGS not only guarantees real-time rendering capabilities, but also introduces an unprecedented level of control and scene editing. This positions 3DGS as a potential game-changer for next-generation 3D reconstruction and representation. To this end, we provide a systematic overview of the latest developments and concerns in the field of 3DGS for the first time.
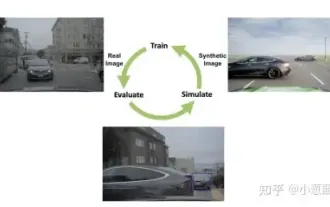
Yesterday during the interview, I was asked whether I had done any long-tail related questions, so I thought I would give a brief summary. The long-tail problem of autonomous driving refers to edge cases in autonomous vehicles, that is, possible scenarios with a low probability of occurrence. The perceived long-tail problem is one of the main reasons currently limiting the operational design domain of single-vehicle intelligent autonomous vehicles. The underlying architecture and most technical issues of autonomous driving have been solved, and the remaining 5% of long-tail problems have gradually become the key to restricting the development of autonomous driving. These problems include a variety of fragmented scenarios, extreme situations, and unpredictable human behavior. The "long tail" of edge scenarios in autonomous driving refers to edge cases in autonomous vehicles (AVs). Edge cases are possible scenarios with a low probability of occurrence. these rare events
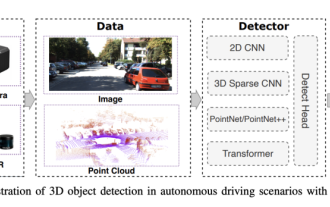
0.Written in front&& Personal understanding that autonomous driving systems rely on advanced perception, decision-making and control technologies, by using various sensors (such as cameras, lidar, radar, etc.) to perceive the surrounding environment, and using algorithms and models for real-time analysis and decision-making. This enables vehicles to recognize road signs, detect and track other vehicles, predict pedestrian behavior, etc., thereby safely operating and adapting to complex traffic environments. This technology is currently attracting widespread attention and is considered an important development area in the future of transportation. one. But what makes autonomous driving difficult is figuring out how to make the car understand what's going on around it. This requires that the three-dimensional object detection algorithm in the autonomous driving system can accurately perceive and describe objects in the surrounding environment, including their locations,
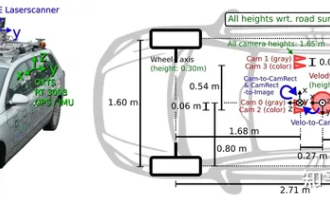
The first pilot and key article mainly introduces several commonly used coordinate systems in autonomous driving technology, and how to complete the correlation and conversion between them, and finally build a unified environment model. The focus here is to understand the conversion from vehicle to camera rigid body (external parameters), camera to image conversion (internal parameters), and image to pixel unit conversion. The conversion from 3D to 2D will have corresponding distortion, translation, etc. Key points: The vehicle coordinate system and the camera body coordinate system need to be rewritten: the plane coordinate system and the pixel coordinate system. Difficulty: image distortion must be considered. Both de-distortion and distortion addition are compensated on the image plane. 2. Introduction There are four vision systems in total. Coordinate system: pixel plane coordinate system (u, v), image coordinate system (x, y), camera coordinate system () and world coordinate system (). There is a relationship between each coordinate system,
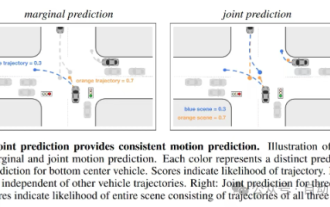
Trajectory prediction plays an important role in autonomous driving. Autonomous driving trajectory prediction refers to predicting the future driving trajectory of the vehicle by analyzing various data during the vehicle's driving process. As the core module of autonomous driving, the quality of trajectory prediction is crucial to downstream planning control. The trajectory prediction task has a rich technology stack and requires familiarity with autonomous driving dynamic/static perception, high-precision maps, lane lines, neural network architecture (CNN&GNN&Transformer) skills, etc. It is very difficult to get started! Many fans hope to get started with trajectory prediction as soon as possible and avoid pitfalls. Today I will take stock of some common problems and introductory learning methods for trajectory prediction! Introductory related knowledge 1. Are the preview papers in order? A: Look at the survey first, p

Original title: SIMPL: ASimpleandEfficientMulti-agentMotionPredictionBaselineforAutonomousDriving Paper link: https://arxiv.org/pdf/2402.02519.pdf Code link: https://github.com/HKUST-Aerial-Robotics/SIMPL Author unit: Hong Kong University of Science and Technology DJI Paper idea: This paper proposes a simple and efficient motion prediction baseline (SIMPL) for autonomous vehicles. Compared with traditional agent-cent
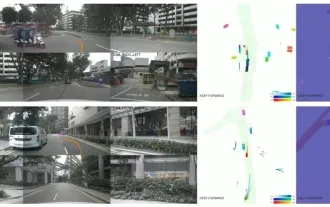
In the past month, due to some well-known reasons, I have had very intensive exchanges with various teachers and classmates in the industry. An inevitable topic in the exchange is naturally end-to-end and the popular Tesla FSDV12. I would like to take this opportunity to sort out some of my thoughts and opinions at this moment for your reference and discussion. How to define an end-to-end autonomous driving system, and what problems should be expected to be solved end-to-end? According to the most traditional definition, an end-to-end system refers to a system that inputs raw information from sensors and directly outputs variables of concern to the task. For example, in image recognition, CNN can be called end-to-end compared to the traditional feature extractor + classifier method. In autonomous driving tasks, input data from various sensors (camera/LiDAR
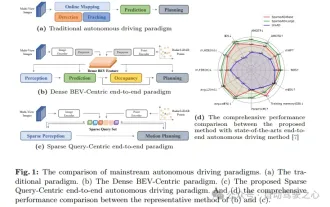
Written in front & starting point The end-to-end paradigm uses a unified framework to achieve multi-tasking in autonomous driving systems. Despite the simplicity and clarity of this paradigm, the performance of end-to-end autonomous driving methods on subtasks still lags far behind single-task methods. At the same time, the dense bird's-eye view (BEV) features widely used in previous end-to-end methods make it difficult to scale to more modalities or tasks. A sparse search-centric end-to-end autonomous driving paradigm (SparseAD) is proposed here, in which sparse search fully represents the entire driving scenario, including space, time, and tasks, without any dense BEV representation. Specifically, a unified sparse architecture is designed for task awareness including detection, tracking, and online mapping. In addition, heavy
