


'AI Perspective Eye', three-time Marr Prize winner Andrew leads a team to solve the problem of occlusion and completion of any object
Occlusion is one of the most basic but still unsolved problems in computer vision, because occlusion means the lack of visual information, but the machine vision system relies on visual information for perception and understanding, and in reality In the world, mutual occlusion between objects is everywhere. The latest work of Andrew Zisserman's team at the VGG Laboratory at the University of Oxford systematically solved the problem of occlusion completion of arbitrary objects and proposed a new and more accurate evaluation data set for this problem. This work was praised by MPI boss Michael Black, the official account of CVPR, the official account of the Department of Computer Science of the University of Southern California, etc. on the X platform. The following is the main content of the paper "Amodal Ground Truth and Completion in the Wild".
- Paper link: https://arxiv.org/pdf/2312.17247.pdf
- Project homepage: https://www.robots.ox.ac.uk/~vgg/research/amodal/
- Code address: https://github.com/Championchess/Amodal-Completion-in-the-Wild
Amodal Segmentation is designed to complete objects that are occluded Part, that is, a shape mask that gives the visible and invisible parts of the object. This task can benefit many downstream tasks: object recognition, target detection, instance segmentation, image editing, 3D reconstruction, video object segmentation, support relationship reasoning between objects, robot manipulation and navigation, because in these tasks it is known that the occluded object is intact The shape will help.
However, how to evaluate the performance of a model for non-modal segmentation in the real world is a difficult problem: although there are a large number of Occluded objects, but how to get the reference standard or non-modal mask of the complete shape of these objects? Previous work has involved manual annotation of non-modal masks, but the reference standards for such annotation are difficult to avoid introducing human errors; there are also works by creating synthetic data sets, such as directly attaching another object to a complete object. Obtain the complete shape of the occluded object, but the pictures obtained in this way are not real picture scenes. Therefore, this work proposes a method through 3D model projection to construct a large-scale real image dataset (MP3D-Amodal) covering multiple object categories and providing amodal masks to accurately evaluate the performance of amodal segmentation. The comparison of different data sets is as follows:
Specifically, taking the MatterPort3D data set as an example, for any real photos and scenes For a three-dimensional structured data set, we can simultaneously project the three-dimensional shapes of all objects in the scene onto the camera to obtain the modal mask of each object (visible shape, because objects are occluding each other), and then project each object in the scene The three-dimensional shape of the object is projected to the camera respectively to obtain the non-modal mask of the object, that is, the complete shape. By comparing the modal mask and the non-modal mask, occluded objects can be picked out.
The statistics of the data set are as follows:
A sample of the data set is as follows:
#In addition, in order to solve the complete shape reconstruction task of any object, the author extracted Extract the prior knowledge about the complete shape of the object from the features of the Stable Diffusion model to perform non-modal segmentation of any occluded object. The specific architecture is as follows (SDAmodal):
The motivation for using Stable Diffusion Feature is that Stable Diffusion has the ability to complete pictures, so it may contain all the information about the object to a certain extent; and because Stable Diffusion After training with a large number of pictures, we can expect its features to have the ability to process any object in any environment. Different from previous two-stage frameworks, SDAmodal does not require marked occlusion masks as input; SDAmodal has a simple structure, but shows strong zero-sample generalization ability (compare Settings F and H in the following table, only in training on COCOA can improve on another data set in a different domain and different categories); even if there is no annotation of occluded objects, SDAmodal can improve on the existing data set COCOA covering multiple types of occluded objects and the newly proposed On the MP3D-Amodal data set, SOTA performance (Setting H) has been achieved.
In addition to quantitative experiments, qualitative comparisons also reflect the advantages of the SDAmodal model: It can be observed from the figure below (all models are only in COCOA training), for different types of occluded objects, whether from COCOA or another MP3D-Amodal, SDAmodal can greatly improve the effect of non-modal segmentation, and the predicted non-modal mask is closer to reality of.
For more details, please read the original paper.
The above is the detailed content of 'AI Perspective Eye', three-time Marr Prize winner Andrew leads a team to solve the problem of occlusion and completion of any object. For more information, please follow other related articles on the PHP Chinese website!

Hot AI Tools

Undresser.AI Undress
AI-powered app for creating realistic nude photos

AI Clothes Remover
Online AI tool for removing clothes from photos.

Undress AI Tool
Undress images for free

Clothoff.io
AI clothes remover

AI Hentai Generator
Generate AI Hentai for free.

Hot Article

Hot Tools

Notepad++7.3.1
Easy-to-use and free code editor

SublimeText3 Chinese version
Chinese version, very easy to use

Zend Studio 13.0.1
Powerful PHP integrated development environment

Dreamweaver CS6
Visual web development tools

SublimeText3 Mac version
God-level code editing software (SublimeText3)

Hot Topics
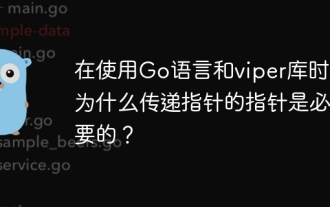
Go pointer syntax and addressing problems in the use of viper library When programming in Go language, it is crucial to understand the syntax and usage of pointers, especially in...
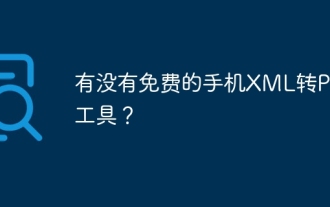
There is no simple and direct free XML to PDF tool on mobile. The required data visualization process involves complex data understanding and rendering, and most of the so-called "free" tools on the market have poor experience. It is recommended to use computer-side tools or use cloud services, or develop apps yourself to obtain more reliable conversion effects.
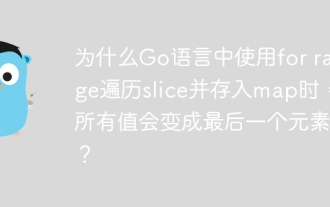
Why does map iteration in Go cause all values to become the last element? In Go language, when faced with some interview questions, you often encounter maps...
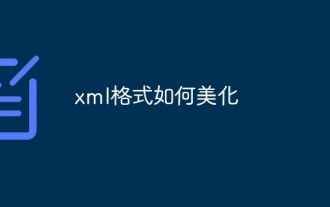
XML beautification is essentially improving its readability, including reasonable indentation, line breaks and tag organization. The principle is to traverse the XML tree, add indentation according to the level, and handle empty tags and tags containing text. Python's xml.etree.ElementTree library provides a convenient pretty_xml() function that can implement the above beautification process.
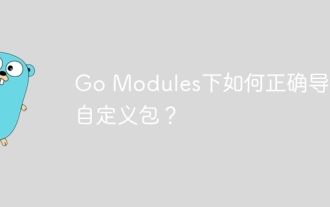
In Go language development, properly introducing custom packages is a crucial step. This article will target "Golang...
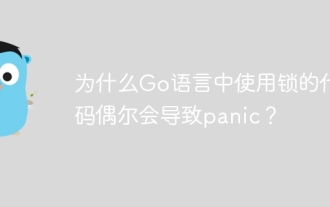
Why does using locks cause panic occasionally? Let's take a look at an interesting question: Why in Go, even if locks are added in the code, sometimes...
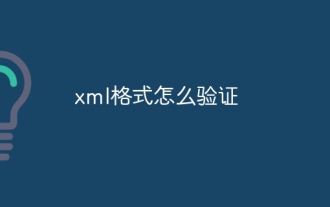
XML format validation involves checking its structure and compliance with DTD or Schema. An XML parser is required, such as ElementTree (basic syntax checking) or lxml (more powerful verification, XSD support). The verification process involves parsing the XML file, loading the XSD Schema, and executing the assertValid method to throw an exception when an error is detected. Verifying the XML format also requires handling various exceptions and gaining insight into the XSD Schema language.
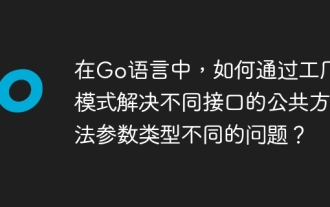
In Go language, how to define a common interface and constrain the methods implemented by the interface, and simultaneously handle the same methods of different interfaces but different parameter types...
