


Using AI short videos to 'feed back' long video understanding, Tencent's MovieLLM framework aims at movie-level continuous frame generation
In the field of video understanding, although multi-modal models have made breakthroughs in short video analysis and demonstrated strong understanding capabilities, when they face movie-level long videos, In the video, it seems powerless. Therefore, the analysis and understanding of long videos, especially the understanding of hours-long movie content, has become a huge challenge today.
The difficulty of the model in understanding long videos mainly stems from the lack of long video data resources, which have defects in quality and diversity. Additionally, collecting and labeling this data requires a lot of work.
Faced with such a problem, the research team from Tencent and Fudan University proposed MovieLLM, an innovative AI generation framework. MovieLLM adopts an innovative method that not only generates high-quality and diverse video data, but also automatically generates a large number of related question and answer data sets, greatly enriching the dimension and depth of the data. At the same time, the entire automated process is also extremely Dadi reduces human investment.
- ##Paper address: https://arxiv.org/abs/2403.01422
- Home page address: https://deaddawn.github.io/MovieLLM/
this Important advances not only improve the model's understanding of complex video narratives, but also enhance the model's analytical capabilities when processing hours-long movie content. At the same time, it overcomes the limitations of scarcity and bias of existing data sets and provides a new and effective way to understand ultra-long video content.
MovieLLM cleverly takes advantage of the powerful generation capabilities of GPT-4 and diffusion models, and adopts a "story expanding" continuous frame description generation strategy. The "textual inversion" method is used to guide the diffusion model to generate scene images that are consistent with the text description, thereby creating continuous frames of a complete movie.
Method Overview
MovieLLM combines GPT-4 and diffusion models to improve large models Understanding long videos. This clever combination produces high-quality, diverse long video data and QA questions and answers, helping to enhance the model's generative capabilities.
MovieLLM mainly includes three stages:
1. Movie plot generation.
Rather than relying on the web or existing datasets to generate plots, MovieLLM fully leverages the power of GPT-4 to produce synthetic data. By providing specific elements such as theme, overview, and style, GPT-4 is guided to produce cinematic keyframe descriptions tailored to the subsequent generation process.
#2. Style fixing process.
MovieLLM cleverly uses "textual inversion" technology to fix the style description generated in the script to the latent space of the diffusion model. This method guides the model to generate scenes with a fixed style and maintain diversity while maintaining a unified aesthetic.
#3. Video command data generation.
Based on the first two steps, fixed style embedding and key frame description have been obtained. Based on these, MovieLLM uses style embedding to guide the diffusion model to generate key frames that conform to key frame descriptions and gradually generates various instructional question and answer pairs according to the movie plot.
#After the above steps, MovieLLM creates high-quality, diverse styles, coherent movie frames and corresponding question and answer pair data. The detailed distribution of movie data types is as follows:
Experimental results
By fine-tuning LLaMA-VID, a large model focused on long video understanding, using data constructed based on MovieLLM, this paper significantly enhances The model's understanding of video content of various lengths. For long video understanding, there is currently no work proposing a test benchmark, so this article also proposes a benchmark for testing long video understanding capabilities.
Although MovieLLM did not specifically construct short video data for training, through training, performance improvements on various short video benchmarks were still observed. The results are as follows:
Compared with the baseline model, there is a significant improvement in the two test data sets of MSVD-QA and MSRVTT-QA.
On the performance benchmark based on video generation, performance improvements were achieved in all five evaluation areas.
#In terms of long video understanding, through the training of MovieLLM, the model's understanding of summary, plot and timing has been significantly improved.
In addition, MovieLLM also has better results in terms of generation quality compared to other similar methods of generating images with fixed styles.
In short, the data generation workflow proposed by MovieLLM significantly reduces the challenge of producing movie-level video data for the model and improves the generation of content. control and diversity. At the same time, MovieLLM significantly enhances the multi-modal model's ability to understand movie-level long videos, providing a valuable reference for other fields to adopt similar data generation methods.
Readers who are interested in this research can read the original text of the paper to learn more about the research content.
The above is the detailed content of Using AI short videos to 'feed back' long video understanding, Tencent's MovieLLM framework aims at movie-level continuous frame generation. For more information, please follow other related articles on the PHP Chinese website!

Hot AI Tools

Undresser.AI Undress
AI-powered app for creating realistic nude photos

AI Clothes Remover
Online AI tool for removing clothes from photos.

Undress AI Tool
Undress images for free

Clothoff.io
AI clothes remover

AI Hentai Generator
Generate AI Hentai for free.

Hot Article

Hot Tools

Notepad++7.3.1
Easy-to-use and free code editor

SublimeText3 Chinese version
Chinese version, very easy to use

Zend Studio 13.0.1
Powerful PHP integrated development environment

Dreamweaver CS6
Visual web development tools

SublimeText3 Mac version
God-level code editing software (SublimeText3)

Hot Topics


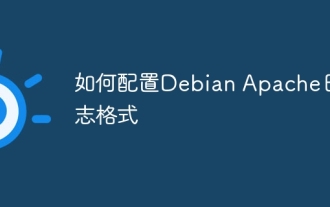
This article describes how to customize Apache's log format on Debian systems. The following steps will guide you through the configuration process: Step 1: Access the Apache configuration file The main Apache configuration file of the Debian system is usually located in /etc/apache2/apache2.conf or /etc/apache2/httpd.conf. Open the configuration file with root permissions using the following command: sudonano/etc/apache2/apache2.conf or sudonano/etc/apache2/httpd.conf Step 2: Define custom log formats to find or
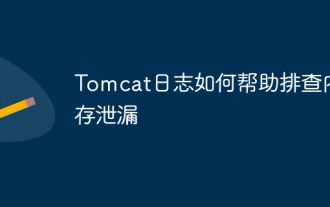
Tomcat logs are the key to diagnosing memory leak problems. By analyzing Tomcat logs, you can gain insight into memory usage and garbage collection (GC) behavior, effectively locate and resolve memory leaks. Here is how to troubleshoot memory leaks using Tomcat logs: 1. GC log analysis First, enable detailed GC logging. Add the following JVM options to the Tomcat startup parameters: -XX: PrintGCDetails-XX: PrintGCDateStamps-Xloggc:gc.log These parameters will generate a detailed GC log (gc.log), including information such as GC type, recycling object size and time. Analysis gc.log
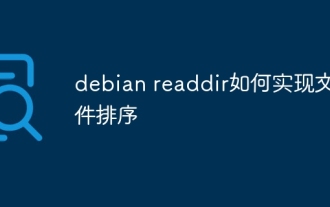
In Debian systems, the readdir function is used to read directory contents, but the order in which it returns is not predefined. To sort files in a directory, you need to read all files first, and then sort them using the qsort function. The following code demonstrates how to sort directory files using readdir and qsort in Debian system: #include#include#include#include#include//Custom comparison function, used for qsortintcompare(constvoid*a,constvoid*b){returnstrcmp(*(
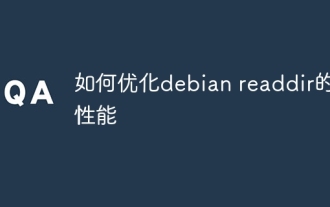
In Debian systems, readdir system calls are used to read directory contents. If its performance is not good, try the following optimization strategy: Simplify the number of directory files: Split large directories into multiple small directories as much as possible, reducing the number of items processed per readdir call. Enable directory content caching: build a cache mechanism, update the cache regularly or when directory content changes, and reduce frequent calls to readdir. Memory caches (such as Memcached or Redis) or local caches (such as files or databases) can be considered. Adopt efficient data structure: If you implement directory traversal by yourself, select more efficient data structures (such as hash tables instead of linear search) to store and access directory information
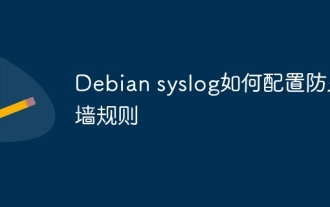
This article describes how to configure firewall rules using iptables or ufw in Debian systems and use Syslog to record firewall activities. Method 1: Use iptablesiptables is a powerful command line firewall tool in Debian system. View existing rules: Use the following command to view the current iptables rules: sudoiptables-L-n-v allows specific IP access: For example, allow IP address 192.168.1.100 to access port 80: sudoiptables-AINPUT-ptcp--dport80-s192.16
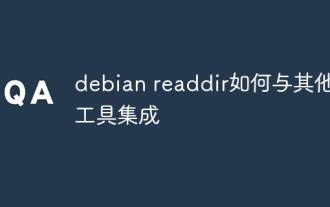
The readdir function in the Debian system is a system call used to read directory contents and is often used in C programming. This article will explain how to integrate readdir with other tools to enhance its functionality. Method 1: Combining C language program and pipeline First, write a C program to call the readdir function and output the result: #include#include#include#includeintmain(intargc,char*argv[]){DIR*dir;structdirent*entry;if(argc!=2){
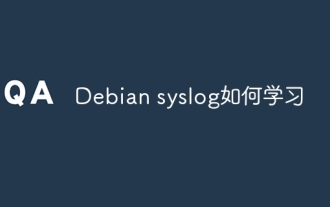
This guide will guide you to learn how to use Syslog in Debian systems. Syslog is a key service in Linux systems for logging system and application log messages. It helps administrators monitor and analyze system activity to quickly identify and resolve problems. 1. Basic knowledge of Syslog The core functions of Syslog include: centrally collecting and managing log messages; supporting multiple log output formats and target locations (such as files or networks); providing real-time log viewing and filtering functions. 2. Install and configure Syslog (using Rsyslog) The Debian system uses Rsyslog by default. You can install it with the following command: sudoaptupdatesud
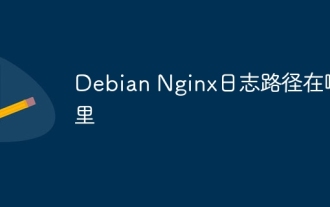
In the Debian system, the default storage locations of Nginx's access log and error log are as follows: Access log (accesslog):/var/log/nginx/access.log Error log (errorlog):/var/log/nginx/error.log The above path is the default configuration of standard DebianNginx installation. If you have modified the log file storage location during the installation process, please check your Nginx configuration file (usually located in /etc/nginx/nginx.conf or /etc/nginx/sites-available/ directory). In the configuration file
