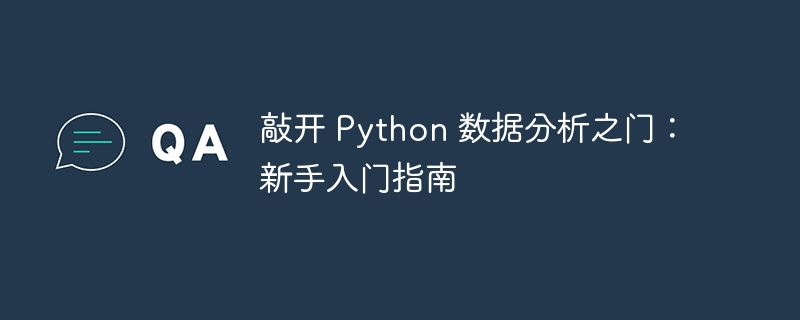
prerequisites
- Basic computer knowledge
- BasicProgrammingConcepts (such as variables, data types, and conditional statements)
- Install python and necessary libraries (such as pandas, NumPy and Matplotlib)
Step 1: Data Import and Exploration
- Use the Pandas library to read data from CSV, excel or database
- ExploreData Structures, Data Types and Statistics
- Find missing values, outliers and data distribution patterns
Step 2: Data Cleaning
- Handle missing values (e.g. fill with mean or median)
- Delete Duplicates
- Convert data type (e.g. convert string to number)
- Normalize data (e.g. convert different measurement units to the same unit)
Step 3: Data Analysis
-
Descriptive statistics: Calculate statistics such as mean, median, standard deviation, etc.
-
Visualization: Use Matplotlib or Seaborn to create charts and graphs to visualize data distribution and trends
-
Hypothesis Testing: Use statistical tests to test hypotheses about the distribution of data and differences between groups
Step 4: Machine Learning
- Use the Scikit-learn library to apply Machine learningAlgorithms, such as regression, classification, and clustering
- Train and evaluate models using cross-validation techniques
- Predict and explain model output
Step 5: Data Mining
- Discover hidden patterns, trends and association rules using data miningtechniques
- Explore correlation analysis, clustering and classification algorithms
- Focus on interpretability and insights into results
Tips and Advice
- Use interactive like Jupyter Notebook or SpyderDevelopmentEnvironment
-
LearnBasic data structures (such as Pandas DataFrame and Series)
- Familiar with data manipulation functions (such as filtering, grouping and merging)
- Get help and support using online tutorials, books, and community forums
- Start with small data sets and simple analysis tasks and gradually increase the difficulty
in conclusion
Mastering the basics of Python Data Analysis takes time and effort, but it is a crucial first step for those working as professionals in the field of data. By following the steps in this guide, newbies can develop the skills they need in data analysis to open career doors for their careers. Leveraging the power of Python, they can discover valuable insights in their data, make informed decisions, and drive business results.
The above is the detailed content of Knocking on the Door of Python Data Analysis: A Beginner's Guide. For more information, please follow other related articles on the PHP Chinese website!